What is Sentiment Analysis? Sentiment analysis is the process of determining the sentiment of a piece of text, such as a tweet or a review.
Papers and Code
Oct 21, 2024
Abstract:Detecting fake news in large datasets is challenging due to its diversity and complexity, with traditional approaches often focusing on textual features while underutilizing semantic and emotional elements. Current methods also rely heavily on large annotated datasets, limiting their effectiveness in more nuanced analysis. To address these challenges, this paper introduces Emotion-\textbf{A}ware \textbf{M}ultimodal Fusion \textbf{P}rompt \textbf{L}\textbf{E}arning (\textbf{AMPLE}) framework to address the above issue by combining text sentiment analysis with multimodal data and hybrid prompt templates. This framework extracts emotional elements from texts by leveraging sentiment analysis tools. It then employs Multi-Head Cross-Attention (MCA) mechanisms and similarity-aware fusion methods to integrate multimodal data. The proposed AMPLE framework demonstrates strong performance on two public datasets in both few-shot and data-rich settings, with results indicating the potential of emotional aspects in fake news detection. Furthermore, the study explores the impact of integrating large language models with this method for text sentiment extraction, revealing substantial room for further improvement. The code can be found at :\url{https://github.com/xxm1215/MMM2025_few-shot/
Via
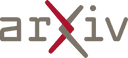
Oct 21, 2024
Abstract:The global rise in depression necessitates innovative detection methods for early intervention. Social media provides a unique opportunity to identify depression through user-generated posts. This systematic review evaluates machine learning (ML) models for depression detection on social media, focusing on biases and methodological challenges throughout the ML lifecycle. A search of PubMed, IEEE Xplore, and Google Scholar identified 47 relevant studies published after 2010. The Prediction model Risk Of Bias ASsessment Tool (PROBAST) was utilized to assess methodological quality and risk of bias. Significant biases impacting model reliability and generalizability were found. There is a predominant reliance on Twitter (63.8%) and English-language content (over 90%), with most studies focusing on users from the United States and Europe. Non-probability sampling methods (approximately 80%) limit representativeness. Only 23% of studies explicitly addressed linguistic nuances like negations, crucial for accurate sentiment analysis. Inconsistent hyperparameter tuning was observed, with only 27.7% properly tuning models. About 17% did not adequately partition data into training, validation, and test sets, risking overfitting. While 74.5% used appropriate evaluation metrics for imbalanced data, others relied on accuracy without addressing class imbalance, potentially skewing results. Reporting transparency varied, often lacking critical methodological details. These findings highlight the need to diversify data sources, standardize preprocessing protocols, ensure consistent model development practices, address class imbalance, and enhance reporting transparency. By overcoming these challenges, future research can develop more robust and generalizable ML models for depression detection on social media, contributing to improved mental health outcomes globally.
Via
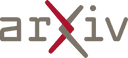
Oct 19, 2024
Abstract:In multimodal sentiment analysis, collecting text data is often more challenging than video or audio due to higher annotation costs and inconsistent automatic speech recognition (ASR) quality. To address this challenge, our study has developed a robust model that effectively integrates multimodal sentiment information, even in the absence of text modality. Specifically, we have developed a Double-Flow Self-Distillation Framework, including Unified Modality Cross-Attention (UMCA) and Modality Imagination Autoencoder (MIA), which excels at processing both scenarios with complete modalities and those with missing text modality. In detail, when the text modality is missing, our framework uses the LLM-based model to simulate the text representation from the audio modality, while the MIA module supplements information from the other two modalities to make the simulated text representation similar to the real text representation. To further align the simulated and real representations, and to enable the model to capture the continuous nature of sample orders in sentiment valence regression tasks, we have also introduced the Rank-N Contrast (RNC) loss function. When testing on the CMU-MOSEI, our model achieved outstanding performance on MAE and significantly outperformed other models when text modality is missing. The code is available at: https://github.com/WarmCongee/SDUMC
Via
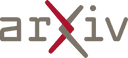
Oct 19, 2024
Abstract:Users of the transit system flood social networks daily with messages that contain valuable insights crucial for improving service quality. These posts help transit agencies quickly identify emerging issues. Parsing topics and sentiments is key to gaining comprehensive insights to foster service excellence. However, the volume of messages makes manual analysis impractical, and standard NLP techniques like Term Frequency-Inverse Document Frequency (TF-IDF) fall short in nuanced interpretation. Traditional sentiment analysis separates topics and sentiments before integrating them, often missing the interaction between them. This incremental approach complicates classification and reduces analytical productivity. To address these challenges, we propose a novel approach to extracting and analyzing transit-related information, including sentiment and sarcasm detection, identification of unusual system problems, and location data from social media. Our method employs Large Language Models (LLM), specifically Llama 3, for a streamlined analysis free from pre-established topic labels. To enhance the model's domain-specific knowledge, we utilize Retrieval-Augmented Generation (RAG), integrating external knowledge sources into the information extraction pipeline. We validated our method through extensive experiments comparing its performance with traditional NLP approaches on user tweet data from the real world transit system. Our results demonstrate the potential of LLMs to transform social media data analysis in the public transit domain, providing actionable insights and enhancing transit agencies' responsiveness by extracting a broader range of information.
* 17 pages, 21 figures
Via
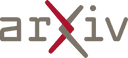
Oct 18, 2024
Abstract:If sentiment analysis tools were valid classifiers, one would expect them to provide comparable results for sentiment classification on different kinds of corpora and for different languages. In line with results of previous studies we show that sentiment analysis tools disagree on the same dataset. Going beyond previous studies we show that the sentiment tool used for sentiment annotation can even be predicted from its outcome, revealing an algorithmic bias of sentiment analysis. Based on Twitter, Wikipedia and different news corpora from the English, German and French languages, our classifiers separate sentiment tools with an averaged F1-score of 0.89 (for the English corpora). We therefore warn against taking sentiment annotations as face value and argue for the need of more and systematic NLP evaluation studies.
Via
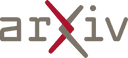
Oct 17, 2024
Abstract:The advancement of large language model (LLM) based artificial intelligence technologies has been a game-changer, particularly in sentiment analysis. This progress has enabled a shift from highly specialized research environments to practical, widespread applications within the industry. However, integrating diverse AI models for processing complex multimodal data and the associated high costs of feature extraction presents significant challenges. Motivated by the marketing oriented software development +needs, our study introduces a collaborative AI framework designed to efficiently distribute and resolve tasks across various AI systems to address these issues. Initially, we elucidate the key solutions derived from our development process, highlighting the role of generative AI models like \emph{chatgpt}, \emph{google gemini} in simplifying intricate sentiment analysis tasks into manageable, phased objectives. Furthermore, we present a detailed case study utilizing our collaborative AI system in edge and cloud, showcasing its effectiveness in analyzing sentiments across diverse online media channels.
Via
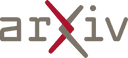
Oct 17, 2024
Abstract:This chapter introduces a research project titled "Analyzing the Political Discourse: A Collaboration Between Humans and Artificial Intelligence", which was initiated in preparation for Greece's 2023 general elections. The project focused on the analysis of political leaders' campaign speeches, employing Artificial Intelligence (AI), in conjunction with an interdisciplinary team comprising journalists, a political scientist, and data scientists. The chapter delves into various aspects of political discourse analysis, including sentiment analysis, polarization, populism, topic detection, and Named Entities Recognition (NER). This experimental study investigates the capabilities of large language model (LLMs), and in particular OpenAI's ChatGPT, for analyzing political speech, evaluates its strengths and weaknesses, and highlights the essential role of human oversight in using AI in journalism projects and potentially other societal sectors. The project stands as an innovative example of human-AI collaboration (known also as "hybrid intelligence") within the realm of digital humanities, offering valuable insights for future initiatives.
Via
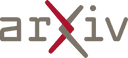
Oct 16, 2024
Abstract:Crude oil, a critical component of the global economy, has its prices influenced by various factors such as economic trends, political events, and natural disasters. Traditional prediction methods based on historical data have their limits in forecasting, but recent advancements in natural language processing bring new possibilities for event-based analysis. In particular, Language Models (LM) and their advancement, the Generative Pre-trained Transformer (GPT), have shown potential in classifying vast amounts of natural language. However, these LMs often have difficulty with domain-specific terminology, limiting their effectiveness in the crude oil sector. Addressing this gap, we introduce CrudeBERT, a fine-tuned LM specifically for the crude oil market. The results indicate that CrudeBERT's sentiment scores align more closely with the WTI Futures curve and significantly enhance price predictions, underscoring the crucial role of integrating economic principles into LMs.
* Enterprise Information Systems. ICEIS 2023. Lecture Notes in
Business Information Processing, vol 518. Springer, Cham
Via
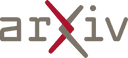
Oct 16, 2024
Abstract:The sarcasm detection task in natural language processing tries to classify whether an utterance is sarcastic or not. It is related to sentiment analysis since it often inverts surface sentiment. Because sarcastic sentences are highly dependent on context, and they are often accompanied by various non-verbal cues, the task is challenging. Most of related work focuses on high-resourced languages like English. To build a sarcasm detection dataset for a less-resourced language, such as Slovenian, we leverage two modern techniques: a machine translation specific medium-size transformer model, and a very large generative language model. We explore the viability of translated datasets and how the size of a pretrained transformer affects its ability to detect sarcasm. We train ensembles of detection models and evaluate models' performance. The results show that larger models generally outperform smaller ones and that ensembling can slightly improve sarcasm detection performance. Our best ensemble approach achieves an $\text{F}_1$-score of 0.765 which is close to annotators' agreement in the source language.
* Proceedings of the 27th International Multiconference INFORMATION
SOCIETY - IS 2024, Volume A, 2024, pages 19-22
* 4 pages, published in the Slovenian Conference on Artificial
Intelligence
Via
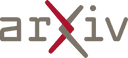
Oct 16, 2024
Abstract:In languages without orthographic word boundaries, NLP models perform word segmentation, either as an explicit preprocessing step or as an implicit step in an end-to-end computation. This paper shows that Chinese NLP models are vulnerable to morphological garden path errors: errors caused by a failure to resolve local word segmentation ambiguities using sentence-level morphosyntactic context. We propose a benchmark, ERAS, that tests a model's vulnerability to morphological garden path errors by comparing its behavior on sentences with and without local segmentation ambiguities. Using ERAS, we show that word segmentation models make garden path errors on locally ambiguous sentences, but do not make equivalent errors on unambiguous sentences. We further show that sentiment analysis models with character-level tokenization make implicit garden path errors, even without an explicit word segmentation step in the pipeline. Our results indicate that models' segmentation of Chinese text often fails to account for morphosyntactic context.
* Under review in ARR/NAACL
Via
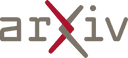