What is Object Detection? Object detection is a computer vision task in which the goal is to detect and locate objects of interest in an image or video. The task involves identifying the position and boundaries of objects in an image, and classifying the objects into different categories. It forms a crucial part of vision recognition, alongside image classification and retrieval.
Papers and Code
Jun 26, 2025
Abstract:Deep neural networks have set the state-of-the-art in computer vision tasks such as bounding box detection and semantic segmentation. Object detectors and segmentation models assign confidence scores to predictions, reflecting the model's uncertainty in object detection or pixel-wise classification. However, these confidence estimates are often miscalibrated, as their architectures and loss functions are tailored to task performance rather than probabilistic foundation. Even with well calibrated predictions, object detectors fail to quantify uncertainty outside detected bounding boxes, i.e., the model does not make a probability assessment of whether an area without detected objects is truly free of obstacles. This poses a safety risk in applications such as automated driving, where uncertainty in empty areas remains unexplored. In this work, we propose an object detection model grounded in spatial statistics. Bounding box data matches realizations of a marked point process, commonly used to describe the probabilistic occurrence of spatial point events identified as bounding box centers, where marks are used to describe the spatial extension of bounding boxes and classes. Our statistical framework enables a likelihood-based training and provides well-defined confidence estimates for whether a region is drivable, i.e., free of objects. We demonstrate the effectiveness of our method through calibration assessments and evaluation of performance.
* 15 pages, 4 figures, 3 tables
Via
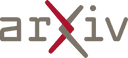
Jun 26, 2025
Abstract:Effective deep feature extraction via feature-level fusion is crucial for multimodal object detection. However, previous studies often involve complex training processes that integrate modality-specific features by stacking multiple feature-level fusion units, leading to significant computational overhead. To address this issue, we propose a new fusion detection baseline that uses a single feature-level fusion unit to enable high-performance detection, thereby simplifying the training process. Based on this approach, we propose a lightweight attention-guided self-modulation feature fusion network (LASFNet), which introduces a novel attention-guided self-modulation feature fusion (ASFF) module that adaptively adjusts the responses of fusion features at both global and local levels based on attention information from different modalities, thereby promoting comprehensive and enriched feature generation. Additionally, a lightweight feature attention transformation module (FATM) is designed at the neck of LASFNet to enhance the focus on fused features and minimize information loss. Extensive experiments on three representative datasets demonstrate that, compared to state-of-the-art methods, our approach achieves a favorable efficiency-accuracy trade-off, reducing the number of parameters and computational cost by as much as 90% and 85%, respectively, while improving detection accuracy (mAP) by 1%-3%. The code will be open-sourced at https://github.com/leileilei2000/LASFNet.
Via
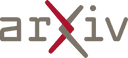
Jun 26, 2025
Abstract:Autonomous systems rely on sensors to estimate the environment around them. However, cameras, LiDARs, and RADARs have their own limitations. In nighttime or degraded environments such as fog, mist, or dust, thermal cameras can provide valuable information regarding the presence of objects of interest due to their heat signature. They make it easy to identify humans and vehicles that are usually at higher temperatures compared to their surroundings. In this paper, we focus on the adaptation of thermal cameras for robotics and automation, where the biggest hurdle is the lack of data. Several multi-modal datasets are available for driving robotics research in tasks such as scene segmentation, object detection, and depth estimation, which are the cornerstone of autonomous systems. However, they are found to be lacking in thermal imagery. Our paper proposes a solution to augment these datasets with synthetic thermal data to enable widespread and rapid adaptation of thermal cameras. We explore the use of conditional diffusion models to convert existing RGB images to thermal images using self-attention to learn the thermal properties of real-world objects.
* Accepted at Thermal Infrared in Robotics (TIRO) Workshop, ICRA 2025
Via
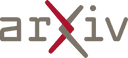
Jun 26, 2025
Abstract:Underground mining operations face significant safety challenges that make emergency response capabilities crucial. While robots have shown promise in assisting with search and rescue operations, their effectiveness depends on reliable miner detection capabilities. Deep learning algorithms offer potential solutions for automated miner detection, but require comprehensive training datasets, which are currently lacking for underground mining environments. This paper presents a novel thermal imaging dataset specifically designed to enable the development and validation of miner detection systems for potential emergency applications. We systematically captured thermal imagery of various mining activities and scenarios to create a robust foundation for detection algorithms. To establish baseline performance metrics, we evaluated several state-of-the-art object detection algorithms including YOLOv8, YOLOv10, YOLO11, and RT-DETR on our dataset. While not exhaustive of all possible emergency situations, this dataset serves as a crucial first step toward developing reliable thermal-based miner detection systems that could eventually be deployed in real emergency scenarios. This work demonstrates the feasibility of using thermal imaging for miner detection and establishes a foundation for future research in this critical safety application.
Via
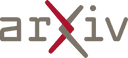
Jun 26, 2025
Abstract:We focus on the source-free domain adaptive object detection (SF-DAOD) problem when source data is unavailable during adaptation and the model must adapt to an unlabeled target domain. The majority of approaches for the problem employ a self-supervised approach using a student-teacher (ST) framework where pseudo-labels are generated via a source-pretrained model for further fine-tuning. We observe that the performance of a student model often degrades drastically, due to the collapse of the teacher model, primarily caused by high noise in pseudo-labels, resulting from domain bias, discrepancies, and a significant domain shift across domains. To obtain reliable pseudo-labels, we propose a Target-based Iterative Query-Token Adversarial Network (TITAN), which separates the target images into two subsets: those similar to the source (easy) and those dissimilar (hard). We propose a strategy to estimate variance to partition the target domain. This approach leverages the insight that higher detection variances correspond to higher recall and greater similarity to the source domain. Also, we incorporate query-token-based adversarial modules into a student-teacher baseline framework to reduce the domain gaps between two feature representations. Experiments conducted on four natural imaging datasets and two challenging medical datasets have substantiated the superior performance of TITAN compared to existing state-of-the-art (SOTA) methodologies. We report an mAP improvement of +22.7, +22.2, +21.1, and +3.7 percent over the current SOTA on C2F, C2B, S2C, and K2C benchmarks, respectively.
* ICCV 2025
Via
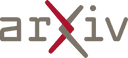
Jun 25, 2025
Abstract:Modern image-based object detection models, such as YOLOv7, primarily process individual frames independently, thus ignoring valuable temporal context naturally present in videos. Meanwhile, existing video-based detection methods often introduce complex temporal modules, significantly increasing model size and computational complexity. In practical applications such as surveillance and autonomous driving, transient challenges including motion blur, occlusions, and abrupt appearance changes can severely degrade single-frame detection performance. To address these issues, we propose a straightforward yet highly effective strategy: stacking multiple consecutive frames as input to a YOLO-based detector while supervising only the output corresponding to a single target frame. This approach leverages temporal information with minimal modifications to existing architectures, preserving simplicity, computational efficiency, and real-time inference capability. Extensive experiments on the challenging MOT20Det and our BOAT360 datasets demonstrate that our method improves detection robustness, especially for lightweight models, effectively narrowing the gap between compact and heavy detection networks. Additionally, we contribute the BOAT360 benchmark dataset, comprising annotated fisheye video sequences captured from a boat, to support future research in multi-frame video object detection in challenging real-world scenarios.
* Submitted to ECMR 2025
Via
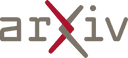
Jun 26, 2025
Abstract:We propose SiM3D, the first benchmark considering the integration of multiview and multimodal information for comprehensive 3D anomaly detection and segmentation (ADS), where the task is to produce a voxel-based Anomaly Volume. Moreover, SiM3D focuses on a scenario of high interest in manufacturing: single-instance anomaly detection, where only one object, either real or synthetic, is available for training. In this respect, SiM3D stands out as the first ADS benchmark that addresses the challenge of generalising from synthetic training data to real test data. SiM3D includes a novel multimodal multiview dataset acquired using top-tier industrial sensors and robots. The dataset features multiview high-resolution images (12 Mpx) and point clouds (7M points) for 333 instances of eight types of objects, alongside a CAD model for each type. We also provide manually annotated 3D segmentation GTs for anomalous test samples. To establish reference baselines for the proposed multiview 3D ADS task, we adapt prominent singleview methods and assess their performance using novel metrics that operate on Anomaly Volumes.
Via
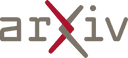
Jun 26, 2025
Abstract:Enforcing helmet regulations among motorcyclists is essential for enhancing road safety and ensuring the effectiveness of traffic management systems. However, automatic detection of helmet violations faces significant challenges due to environmental variability, camera angles, and inconsistencies in the data. These factors hinder reliable detection of motorcycles and riders and disrupt consistent object classification. To address these challenges, we propose VisionGuard, a synergistic multi-stage framework designed to overcome the limitations of frame-wise detectors, especially in scenarios with class imbalance and inconsistent annotations. VisionGuard integrates two key components: Adaptive Labeling and Contextual Expander modules. The Adaptive Labeling module is a tracking-based refinement technique that enhances classification consistency by leveraging a tracking algorithm to assign persistent labels across frames and correct misclassifications. The Contextual Expander module improves recall for underrepresented classes by generating virtual bounding boxes with appropriate confidence scores, effectively addressing the impact of data imbalance. Experimental results show that VisionGuard improves overall mAP by 3.1% compared to baseline detectors, demonstrating its effectiveness and potential for real-world deployment in traffic surveillance systems, ultimately promoting safety and regulatory compliance.
Via
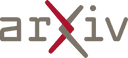
Jun 24, 2025
Abstract:Multi-sensor fusion perception (MSFP) is a key technology for embodied AI, which can serve a variety of downstream tasks (e.g., 3D object detection and semantic segmentation) and application scenarios (e.g., autonomous driving and swarm robotics). Recently, impressive achievements on AI-based MSFP methods have been reviewed in relevant surveys. However, we observe that the existing surveys have some limitations after a rigorous and detailed investigation. For one thing, most surveys are oriented to a single task or research field, such as 3D object detection or autonomous driving. Therefore, researchers in other related tasks often find it difficult to benefit directly. For another, most surveys only introduce MSFP from a single perspective of multi-modal fusion, while lacking consideration of the diversity of MSFP methods, such as multi-view fusion and time-series fusion. To this end, in this paper, we hope to organize MSFP research from a task-agnostic perspective, where methods are reported from various technical views. Specifically, we first introduce the background of MSFP. Next, we review multi-modal and multi-agent fusion methods. A step further, time-series fusion methods are analyzed. In the era of LLM, we also investigate multimodal LLM fusion methods. Finally, we discuss open challenges and future directions for MSFP. We hope this survey can help researchers understand the important progress in MSFP and provide possible insights for future research.
Via
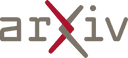
Jun 25, 2025
Abstract:The rapid advancement of generative artificial intelligence is producing fake remote sensing imagery (RSI) that is increasingly difficult to detect, potentially leading to erroneous intelligence, fake news, and even conspiracy theories. Existing forgery detection methods typically rely on single visual features to capture predefined artifacts, such as spatial-domain cues to detect forged objects like roads or buildings in RSI, or frequency-domain features to identify artifacts from up-sampling operations in adversarial generative networks (GANs). However, the nature of artifacts can significantly differ depending on geographic terrain, land cover types, or specific features within the RSI. Moreover, these complex artifacts evolve as generative models become more sophisticated. In short, over-reliance on a single visual cue makes existing forgery detectors struggle to generalize across diverse remote sensing data. This paper proposed a novel forgery detection framework called SFNet, designed to identify fake images in diverse remote sensing data by leveraging spatial and frequency domain features. Specifically, to obtain rich and comprehensive visual information, SFNet employs two independent feature extractors to capture spatial and frequency domain features from input RSIs. To fully utilize the complementary domain features, the domain feature mapping module and the hybrid domain feature refinement module(CBAM attention) of SFNet are designed to successively align and fuse the multi-domain features while suppressing redundant information. Experiments on three datasets show that SFNet achieves an accuracy improvement of 4%-15.18% over the state-of-the-art RS forgery detection methods and exhibits robust generalization capabilities. The code is available at https://github.com/GeoX-Lab/RSTI/tree/main/SFNet.
Via
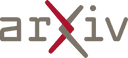