Topic:Line Art Colorization
What is Line Art Colorization? Line art colorization is the process of adding color to black and white line art using deep learning techniques.
Papers and Code
Apr 16, 2025
Abstract:The comic production industry requires reference-based line art colorization with high accuracy, efficiency, contextual consistency, and flexible control. A comic page often involves diverse characters, objects, and backgrounds, which complicates the coloring process. Despite advancements in diffusion models for image generation, their application in line art colorization remains limited, facing challenges related to handling extensive reference images, time-consuming inference, and flexible control. We investigate the necessity of extensive contextual image guidance on the quality of line art colorization. To address these challenges, we introduce Cobra, an efficient and versatile method that supports color hints and utilizes over 200 reference images while maintaining low latency. Central to Cobra is a Causal Sparse DiT architecture, which leverages specially designed positional encodings, causal sparse attention, and Key-Value Cache to effectively manage long-context references and ensure color identity consistency. Results demonstrate that Cobra achieves accurate line art colorization through extensive contextual reference, significantly enhancing inference speed and interactivity, thereby meeting critical industrial demands. We release our codes and models on our project page: https://zhuang2002.github.io/Cobra/.
Via
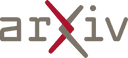
Mar 20, 2025
Abstract:Recovering high-frequency textures in image demosaicking remains a challenging issue. While existing methods introduced elaborate spatial learning methods, they still exhibit limited performance. To address this issue, a frequency enhancement approach is proposed. Based on the frequency analysis of color filter array (CFA)/demosaicked/ground truth images, we propose Dual-path Frequency Enhancement Network (DFENet), which reconstructs RGB images in a divide-and-conquer manner through fourier-domain frequency selection. In DFENet, two frequency selectors are employed, each selecting a set of frequency components for processing along separate paths. One path focuses on generating missing information through detail refinement in spatial domain, while the other aims at suppressing undesirable frequencies with the guidance of CFA images in frequency domain. Multi-level frequency supervision with a stagewise training strategy is employed to further improve the reconstruction performance. With these designs, the proposed DFENet outperforms other state-of-the-art algorithms on different datasets and demonstrates significant advantages on hard cases. Moreover, to better assess algorithms' ability to reconstruct high-frequency textures, a new dataset, LineSet37, is contributed, which consists of 37 artificially designed and generated images. These images feature complex line patterns and are prone to severe visual artifacts like color moir\'e after demosaicking. Experiments on LineSet37 offer a more targeted evaluation of performance on challenging cases. The code and dataset are available at https://github.com/VelvetReverie/DFENet-demosaicking.
* 14 pages, 8 figures
Via
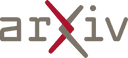
Jan 14, 2025
Abstract:Derived from diffusion models, MangaNinjia specializes in the task of reference-guided line art colorization. We incorporate two thoughtful designs to ensure precise character detail transcription, including a patch shuffling module to facilitate correspondence learning between the reference color image and the target line art, and a point-driven control scheme to enable fine-grained color matching. Experiments on a self-collected benchmark demonstrate the superiority of our model over current solutions in terms of precise colorization. We further showcase the potential of the proposed interactive point control in handling challenging cases, cross-character colorization, multi-reference harmonization, beyond the reach of existing algorithms.
Via
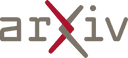
Dec 18, 2024
Abstract:The production of 2D animation follows an industry-standard workflow, encompassing four essential stages: character design, keyframe animation, in-betweening, and coloring. Our research focuses on reducing the labor costs in the above process by harnessing the potential of increasingly powerful generative AI. Using video diffusion models as the foundation, AniDoc emerges as a video line art colorization tool, which automatically converts sketch sequences into colored animations following the reference character specification. Our model exploits correspondence matching as an explicit guidance, yielding strong robustness to the variations (e.g., posture) between the reference character and each line art frame. In addition, our model could even automate the in-betweening process, such that users can easily create a temporally consistent animation by simply providing a character image as well as the start and end sketches. Our code is available at: https://yihao-meng.github.io/AniDoc_demo.
Via
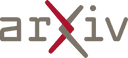
Jan 14, 2025
Abstract:Depth completion is a key task in autonomous driving, aiming to complete sparse LiDAR depth measurements into high-quality dense depth maps through image guidance. However, existing methods usually treat depth maps as an additional channel of color images, or directly perform convolution on sparse data, failing to fully exploit the 3D geometric information in depth maps, especially with limited performance in complex boundaries and sparse areas. To address these issues, this paper proposes a depth completion network combining channel attention mechanism and 3D global feature perception (CGA-Net). The main innovations include: 1) Utilizing PointNet++ to extract global 3D geometric features from sparse depth maps, enhancing the scene perception ability of low-line LiDAR data; 2) Designing a channel-attention-based multimodal feature fusion module to efficiently integrate sparse depth, RGB images, and 3D geometric features; 3) Combining residual learning with CSPN++ to optimize the depth refinement stage, further improving the completion quality in edge areas and complex scenes. Experiments on the KITTI depth completion dataset show that CGA-Net can significantly improve the prediction accuracy of dense depth maps, achieving a new state-of-the-art (SOTA), and demonstrating strong robustness to sparse and complex scenes.
* 13pages,4 figures, 2 tables
Via
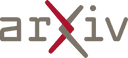
Oct 25, 2024
Abstract:Line art colorization plays a crucial role in hand-drawn animation production, where digital artists manually colorize segments using a paint bucket tool, guided by RGB values from character color design sheets. This process, often called paint bucket colorization, involves two main tasks: keyframe colorization, where colors are applied according to the character's color design sheet, and consecutive frame colorization, where these colors are replicated across adjacent frames. Current automated colorization methods primarily focus on reference-based and segment-matching approaches. However, reference-based methods often fail to accurately assign specific colors to each region, while matching-based methods are limited to consecutive frame colorization and struggle with issues like significant deformation and occlusion. In this work, we introduce inclusion matching, which allows the network to understand the inclusion relationships between segments, rather than relying solely on direct visual correspondences. By integrating this approach with segment parsing and color warping modules, our inclusion matching pipeline significantly improves performance in both keyframe colorization and consecutive frame colorization. To support our network's training, we have developed a unique dataset named PaintBucket-Character, which includes rendered line arts alongside their colorized versions and shading annotations for various 3D characters. To replicate industry animation data formats, we also created color design sheets for each character, with semantic information for each color and standard pose reference images. Experiments highlight the superiority of our method, demonstrating accurate and consistent colorization across both our proposed benchmarks and hand-drawn animations.
* Extension of arXiv:2403.18342; Project page at
https://github.com/ykdai/BasicPBC
Via
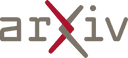
Nov 22, 2024
Abstract:As the text-to-image (T2I) domain progresses, generating text that seamlessly integrates with visual content has garnered significant attention. However, even with accurate text generation, the inability to control font and color can greatly limit certain applications, and this issue remains insufficiently addressed. This paper introduces AnyText2, a novel method that enables precise control over multilingual text attributes in natural scene image generation and editing. Our approach consists of two main components. First, we propose a WriteNet+AttnX architecture that injects text rendering capabilities into a pre-trained T2I model. Compared to its predecessor, AnyText, our new approach not only enhances image realism but also achieves a 19.8% increase in inference speed. Second, we explore techniques for extracting fonts and colors from scene images and develop a Text Embedding Module that encodes these text attributes separately as conditions. As an extension of AnyText, this method allows for customization of attributes for each line of text, leading to improvements of 3.3% and 9.3% in text accuracy for Chinese and English, respectively. Through comprehensive experiments, we demonstrate the state-of-the-art performance of our method. The code and model will be made open-source in https://github.com/tyxsspa/AnyText2.
Via
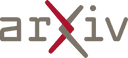
Oct 22, 2024
Abstract:Jigsaw puzzle solving is a challenging task for computer vision since it requires high-level spatial and semantic reasoning. To solve the problem, existing approaches invariably use color and/or shape information but in many real-world scenarios, such as in archaeological fresco reconstruction, this kind of clues is often unreliable due to severe physical and pictorial deterioration of the individual fragments. This makes state-of-the-art approaches entirely unusable in practice. On the other hand, in such cases, simple geometrical patterns such as lines or curves offer a powerful yet unexplored clue. In an attempt to fill in this gap, in this paper we introduce a new challenging version of the puzzle solving problem in which one deliberately ignores conventional color and shape features and relies solely on the presence of linear geometrical patterns. The reconstruction process is then only driven by one of the most fundamental principles of Gestalt perceptual organization, namely Wertheimer's {\em law of good continuation}. In order to tackle this problem, we formulate the puzzle solving problem as the problem of finding a Nash equilibrium of a (noncooperative) multiplayer game and use classical multi-population replicator dynamics to solve it. The proposed approach is general and allows us to deal with pieces of arbitrary shape, size and orientation. We evaluate our approach on both synthetic and real-world data and compare it with state-of-the-art algorithms. The results show the intrinsic complexity of our purely line-based puzzle problem as well as the relative effectiveness of our game-theoretic formulation.
* ACCV2024
* to be published in ACCV2024
Via
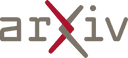
Mar 27, 2024
Abstract:Colorizing line art is a pivotal task in the production of hand-drawn cel animation. This typically involves digital painters using a paint bucket tool to manually color each segment enclosed by lines, based on RGB values predetermined by a color designer. This frame-by-frame process is both arduous and time-intensive. Current automated methods mainly focus on segment matching. This technique migrates colors from a reference to the target frame by aligning features within line-enclosed segments across frames. However, issues like occlusion and wrinkles in animations often disrupt these direct correspondences, leading to mismatches. In this work, we introduce a new learning-based inclusion matching pipeline, which directs the network to comprehend the inclusion relationships between segments rather than relying solely on direct visual correspondences. Our method features a two-stage pipeline that integrates a coarse color warping module with an inclusion matching module, enabling more nuanced and accurate colorization. To facilitate the training of our network, we also develope a unique dataset, referred to as PaintBucket-Character. This dataset includes rendered line arts alongside their colorized counterparts, featuring various 3D characters. Extensive experiments demonstrate the effectiveness and superiority of our method over existing techniques.
Via
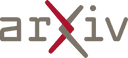
Jul 03, 2024
Abstract:Social networks are creating a digital world in which the cognitive, emotional, and pragmatic value of the imagery of human faces and bodies is arguably changing. However, researchers in the digital humanities are often ill-equipped to study these phenomena at scale. This work presents FRESCO (Face Representation in E-Societies through Computational Observation), a framework designed to explore the socio-cultural implications of images on social media platforms at scale. FRESCO deconstructs images into numerical and categorical variables using state-of-the-art computer vision techniques, aligning with the principles of visual semiotics. The framework analyzes images across three levels: the plastic level, encompassing fundamental visual features like lines and colors; the figurative level, representing specific entities or concepts; and the enunciation level, which focuses particularly on constructing the point of view of the spectator and observer. These levels are analyzed to discern deeper narrative layers within the imagery. Experimental validation confirms the reliability and utility of FRESCO, and we assess its consistency and precision across two public datasets. Subsequently, we introduce the FRESCO score, a metric derived from the framework's output that serves as a reliable measure of similarity in image content.
Via
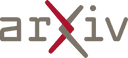