May 04, 2025
Abstract:Nanobodies, single-domain antibody fragments derived from camelid heavy-chain-only antibodies, exhibit unique advantages such as compact size, high stability, and strong binding affinity, making them valuable tools in therapeutics and diagnostics. While recent advances in pretrained protein and antibody language models (PPLMs and PALMs) have greatly enhanced biomolecular understanding, nanobody-specific modeling remains underexplored and lacks a unified benchmark. To address this gap, we introduce NbBench, the first comprehensive benchmark suite for nanobody representation learning. Spanning eight biologically meaningful tasks across nine curated datasets, NbBench encompasses structure annotation, binding prediction, and developability assessment. We systematically evaluate eleven representative models--including general-purpose protein LMs, antibody-specific LMs, and nanobody-specific LMs--in a frozen setting. Our analysis reveals that antibody language models excel in antigen-related tasks, while performance on regression tasks such as thermostability and affinity remains challenging across all models. Notably, no single model consistently outperforms others across all tasks. By standardizing datasets, task definitions, and evaluation protocols, NbBench offers a reproducible foundation for assessing and advancing nanobody modeling.
Via
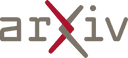