What is Age Estimation? Age estimation is the process of predicting a person's age from images or videos.
Papers and Code
Jul 17, 2025
Abstract:Accurate age verification can protect underage users from unauthorized access to online platforms and e-commerce sites that provide age-restricted services. However, accurate age estimation can be confounded by several factors, including facial makeup that can induce changes to alter perceived identity and age to fool both humans and machines. In this work, we propose DiffClean which erases makeup traces using a text-guided diffusion model to defend against makeup attacks. DiffClean improves age estimation (minor vs. adult accuracy by 4.8%) and face verification (TMR by 8.9% at FMR=0.01%) over competing baselines on digitally simulated and real makeup images.
Via
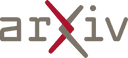
Jul 10, 2025
Abstract:Facial Expression Recognition (FER) systems based on deep learning have achieved impressive performance in recent years. However, these models often exhibit demographic biases, particularly with respect to age, which can compromise their fairness and reliability. In this work, we present a comprehensive study of age-related bias in deep FER models, with a particular focus on the elderly population. We first investigate whether recognition performance varies across age groups, which expressions are most affected, and whether model attention differs depending on age. Using Explainable AI (XAI) techniques, we identify systematic disparities in expression recognition and attention patterns, especially for "neutral", "sadness", and "anger" in elderly individuals. Based on these findings, we propose and evaluate three bias mitigation strategies: Multi-task Learning, Multi-modal Input, and Age-weighted Loss. Our models are trained on a large-scale dataset, AffectNet, with automatically estimated age labels and validated on balanced benchmark datasets that include underrepresented age groups. Results show consistent improvements in recognition accuracy for elderly individuals, particularly for the most error-prone expressions. Saliency heatmap analysis reveals that models trained with age-aware strategies attend to more relevant facial regions for each age group, helping to explain the observed improvements. These findings suggest that age-related bias in FER can be effectively mitigated using simple training modifications, and that even approximate demographic labels can be valuable for promoting fairness in large-scale affective computing systems.
Via
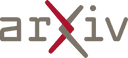
Jul 10, 2025
Abstract:In pediatric orthodontics, accurate estimation of growth potential is essential for developing effective treatment strategies. Our research aims to predict this potential by identifying the growth peak and analyzing cervical vertebra morphology solely through lateral cephalometric radiographs. We accomplish this by comprehensively analyzing cervical vertebral maturation (CVM) features from these radiographs. This methodology provides clinicians with a reliable and efficient tool to determine the optimal timings for orthodontic interventions, ultimately enhancing patient outcomes. A crucial aspect of this approach is the meticulous annotation of keypoints on the cervical vertebrae, a task often challenged by its labor-intensive nature. To mitigate this, we introduce Attend-and-Refine Network (ARNet), a user-interactive, deep learning-based model designed to streamline the annotation process. ARNet features Interaction-guided recalibration network, which adaptively recalibrates image features in response to user feedback, coupled with a morphology-aware loss function that preserves the structural consistency of keypoints. This novel approach substantially reduces manual effort in keypoint identification, thereby enhancing the efficiency and accuracy of the process. Extensively validated across various datasets, ARNet demonstrates remarkable performance and exhibits wide-ranging applicability in medical imaging. In conclusion, our research offers an effective AI-assisted diagnostic tool for assessing growth potential in pediatric orthodontics, marking a significant advancement in the field.
* Accepted to Medical Image Analysis (2025)
Via
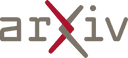
Jul 10, 2025
Abstract:In the age of large and heterogeneous datasets, the integration of information from diverse sources is essential to improve parameter estimation. Multi-task learning offers a powerful approach by enabling simultaneous learning across related tasks. In this work, we introduce a late fusion framework for multi-task learning with semiparametric models that involve infinite-dimensional nuisance parameters, focusing on applications such as heterogeneous treatment effect estimation across multiple data sources, including electronic health records from different hospitals or clinical trial data. Our framework is two-step: first, initial double machine-learning estimators are obtained through individual task learning; second, these estimators are adaptively aggregated to exploit task similarities while remaining robust to task-specific differences. In particular, the framework avoids individual level data sharing, preserving privacy. Additionally, we propose a novel multi-task learning method for nuisance parameter estimation, which further enhances parameter estimation when nuisance parameters exhibit similarity across tasks. We establish theoretical guarantees for the method, demonstrating faster convergence rates compared to individual task learning when tasks share similar parametric components. Extensive simulations and real data applications complement the theoretical findings of our work while highlight the effectiveness of our framework even in moderate sample sizes.
* 21 pages, 3 figures
Via
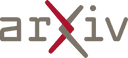
Jul 02, 2025
Abstract:Estimating brain age from structural MRI has emerged as a powerful tool for characterizing normative and pathological aging. In this work, we explore contrastive learning as a scalable and robust alternative to supervised approaches for brain age estimation. We introduce a novel contrastive loss function, $\mathcal{L}^{exp}$, and evaluate it across multiple public neuroimaging datasets comprising over 20,000 scans. Our experiments reveal four key findings. First, scaling pre-training on diverse, multi-site data consistently improves generalization performance, cutting external mean absolute error (MAE) nearly in half. Second, $\mathcal{L}^{exp}$ is robust to site-related confounds, maintaining low scanner-predictability as training size increases. Third, contrastive models reliably capture accelerated aging in patients with cognitive impairment and Alzheimer's disease, as shown through brain age gap analysis, ROC curves, and longitudinal trends. Lastly, unlike supervised baselines, $\mathcal{L}^{exp}$ maintains a strong correlation between brain age accuracy and downstream diagnostic performance, supporting its potential as a foundation model for neuroimaging. These results position contrastive learning as a promising direction for building generalizable and clinically meaningful brain representations.
* 11 pages
Via
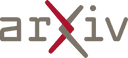
Jul 02, 2025
Abstract:Massive multi-input multi-output (MIMO) combined with orthogonal time frequency space (OTFS) modulation has emerged as a promising technique for high-mobility scenarios. However, its performance could be severely degraded due to channel aging caused by user mobility and high processing latency. In this paper, an integrated scheme of uplink (UL) channel estimation and downlink (DL) channel prediction is proposed to alleviate channel aging in time division duplex (TDD) massive MIMO-OTFS systems. Specifically, first, an iterative basis expansion model (BEM) based UL channel estimation scheme is proposed to accurately estimate UL channels with the aid of carefully designed OTFS frame pattern. Then a set of Slepian sequences are used to model the estimated UL channels, and the dynamic Slepian coefficients are fitted by a set of orthogonal polynomials. A channel predictor is derived to predict DL channels by iteratively extrapolating the Slepian coefficients. Simulation results verify that the proposed UL channel estimation and DL channel prediction schemes outperform the existing schemes in terms of normalized mean square error of channel estimation/prediction and DL spectral efficiency, with less pilot overhead.
Via
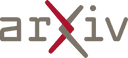
Jul 02, 2025
Abstract:Purpose: This study evaluated whether articulatory kinematics, inferred by Articulatory Phonology speech inversion neural networks, aligned with perceptual ratings of /r/ and /s/ in the speech of children with speech sound disorders. Methods: Articulatory Phonology vocal tract variables were inferred for 5,961 utterances from 118 children and 3 adults, aged 2.25-45 years. Perceptual ratings were standardized using the novel 5-point PERCEPT Rating Scale and training protocol. Two research questions examined if the articulatory patterns of inferred vocal tract variables aligned with the perceptual error category for the phones investigated (e.g., tongue tip is more anterior in dentalized /s/ productions than in correct /s/). A third research question examined if gradient PERCEPT Rating Scale scores predicted articulatory proximity to correct productions. Results: Estimated marginal means from linear mixed models supported 17 of 18 /r/ hypotheses, involving tongue tip and tongue body constrictions. For /s/, estimated marginal means from a second linear mixed model supported 7 of 15 hypotheses, particularly those related to the tongue tip. A third linear mixed model revealed that PERCEPT Rating Scale scores significantly predicted articulatory proximity of errored phones to correct productions. Conclusion: Inferred vocal tract variables differentiated category and magnitude of articulatory errors for /r/, and to a lesser extent for /s/, aligning with perceptual judgments. These findings support the clinical interpretability of speech inversion vocal tract variables and the PERCEPT Rating Scale in quantifying articulatory proximity to the target sound, particularly for /r/.
* This manuscript is in submission for publication. It has not yet been
peer reviewed
Via
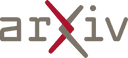
Jun 16, 2025
Abstract:Facial age estimation has achieved considerable success under controlled conditions. However, in unconstrained real-world scenarios, which are often referred to as 'in the wild', age estimation remains challenging, especially when faces are partially occluded, which may obscure their visibility. To address this limitation, we propose a new approach integrating generative adversarial networks (GANs) and transformer architectures to enable robust age estimation from occluded faces. We employ an SN-Patch GAN to effectively remove occlusions, while an Attentive Residual Convolution Module (ARCM), paired with a Swin Transformer, enhances feature representation. Additionally, we introduce a Multi-Task Age Head (MTAH) that combines regression and distribution learning, further improving age estimation under occlusion. Experimental results on the FG-NET, UTKFace, and MORPH datasets demonstrate that our proposed approach surpasses existing state-of-the-art techniques for occluded facial age estimation by achieving an MAE of $3.00$, $4.54$, and $2.53$ years, respectively.
Via
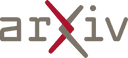
Jun 17, 2025
Abstract:Background: Facial appearance offers a noninvasive window into health. We built FAHR-Face, a foundation model trained on >40 million facial images and fine-tuned it for two distinct tasks: biological age estimation (FAHR-FaceAge) and survival risk prediction (FAHR-FaceSurvival). Methods: FAHR-FaceAge underwent a two-stage, age-balanced fine-tuning on 749,935 public images; FAHR-FaceSurvival was fine-tuned on 34,389 photos of cancer patients. Model robustness (cosmetic surgery, makeup, pose, lighting) and independence (saliency mapping) was tested extensively. Both models were clinically tested in two independent cancer patient datasets with survival analyzed by multivariable Cox models and adjusted for clinical prognostic factors. Findings: For age estimation, FAHR-FaceAge had the lowest mean absolute error of 5.1 years on public datasets, outperforming benchmark models and maintaining accuracy across the full human lifespan. In cancer patients, FAHR-FaceAge outperformed a prior facial age estimation model in survival prognostication. FAHR-FaceSurvival demonstrated robust prediction of mortality, and the highest-risk quartile had more than triple the mortality of the lowest (adjusted hazard ratio 3.22; P<0.001). These findings were validated in the independent cohort and both models showed generalizability across age, sex, race and cancer subgroups. The two algorithms provided distinct, complementary prognostic information; saliency mapping revealed each model relied on distinct facial regions. The combination of FAHR-FaceAge and FAHR-FaceSurvival improved prognostic accuracy. Interpretation: A single foundation model can generate inexpensive, scalable facial biomarkers that capture both biological ageing and disease-related mortality risk. The foundation model enabled effective training using relatively small clinical datasets.
Via
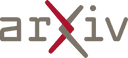
Jun 11, 2025
Abstract:Platforms and the law regulate digital content depicting minors (defined as individuals under 18 years of age) differently from other types of content. Given the sheer amount of content that needs to be assessed, machine learning-based automation tools are commonly used to detect content depicting minors. To our knowledge, no dataset or benchmark currently exists for detecting these identification methods in a multi-modal environment. To fill this gap, we release the Image-Caption Children in the Wild Dataset (ICCWD), an image-caption dataset aimed at benchmarking tools that detect depictions of minors. Our dataset is richer than previous child image datasets, containing images of children in a variety of contexts, including fictional depictions and partially visible bodies. ICCWD contains 10,000 image-caption pairs manually labeled to indicate the presence or absence of a child in the image. To demonstrate the possible utility of our dataset, we use it to benchmark three different detectors, including a commercial age estimation system applied to images. Our results suggest that child detection is a challenging task, with the best method achieving a 75.3% true positive rate. We hope the release of our dataset will aid in the design of better minor detection methods in a wide range of scenarios.
* 14 pages, 6 figures
Via
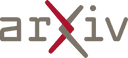