What is speech recognition? Speech recognition is the task of identifying words spoken aloud, analyzing the voice and language, and accurately transcribing the words.
Papers and Code
Jun 07, 2025
Abstract:Audio Large Language Models (AudioLLMs) have achieved strong results in semantic tasks like speech recognition and translation, but remain limited in modeling paralinguistic cues such as emotion. Existing approaches often treat emotion understanding as a classification problem, offering little insight into the underlying rationale behind predictions. In this work, we explore emotion reasoning, a strategy that leverages the generative capabilities of AudioLLMs to enhance emotion recognition by producing semantically aligned, evidence-grounded explanations. To support this in multitask AudioLLMs, we introduce a unified framework combining reasoning-augmented data supervision, dual-encoder architecture, and task-alternating training. This approach enables AudioLLMs to effectively learn different tasks while incorporating emotional reasoning. Experiments on IEMOCAP and MELD show that our approach not only improves emotion prediction accuracy but also enhances the coherence and evidential grounding of the generated responses.
Via
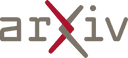
Jun 07, 2025
Abstract:Retentive Network (RetNet) represents a significant advancement in neural network architecture, offering an efficient alternative to the Transformer. While Transformers rely on self-attention to model dependencies, they suffer from high memory costs and limited scalability when handling long sequences due to their quadratic complexity. To mitigate these limitations, RetNet introduces a retention mechanism that unifies the inductive bias of recurrence with the global dependency modeling of attention. This mechanism enables linear-time inference, facilitates efficient modeling of extended contexts, and remains compatible with fully parallelizable training pipelines. RetNet has garnered significant research interest due to its consistently demonstrated cross-domain effectiveness, achieving robust performance across machine learning paradigms including natural language processing, speech recognition, and time-series analysis. However, a comprehensive review of RetNet is still missing from the current literature. This paper aims to fill that gap by offering the first detailed survey of the RetNet architecture, its key innovations, and its diverse applications. We also explore the main challenges associated with RetNet and propose future research directions to support its continued advancement in both academic research and practical deployment.
* 15 pages, 3 figures
Via
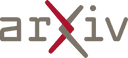
Jun 06, 2025
Abstract:Recent advances in automatic speech recognition (ASR) have combined speech encoders with large language models (LLMs) through projection, forming Speech LLMs with strong performance. However, adapting them to new domains remains challenging, especially in low-resource settings where paired speech-text data is scarce. We propose a text-only fine-tuning strategy for Speech LLMs using unpaired target-domain text without requiring additional audio. To preserve speech-text alignment, we introduce a real-time evaluation mechanism during fine-tuning. This enables effective domain adaptation while maintaining source-domain performance. Experiments on LibriSpeech, SlideSpeech, and Medical datasets show that our method achieves competitive recognition performance, with minimal degradation compared to full audio-text fine-tuning. It also improves generalization to new domains without catastrophic forgetting, highlighting the potential of text-only fine-tuning for low-resource domain adaptation of ASR.
Via
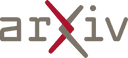
Jun 06, 2025
Abstract:End-to-End Automatic Speech Recognition (ASR) has advanced significantly yet still struggles with rare and domain-specific entities. This paper introduces a simple yet efficient prompt-based biasing technique for contextualized ASR, enhancing recognition accuracy by leverage a unified multitask learning framework. The approach comprises two key components: a prompt biasing model which is trained to determine when to focus on entities in prompt, and a entity filtering mechanism which efficiently filters out irrelevant entities. Our method significantly enhances ASR accuracy on entities, achieving a relative 30.7% and 18.0% reduction in Entity Word Error Rate compared to the baseline model with shallow fusion on in-house domain dataset with small and large entity lists, respectively. The primary advantage of this method lies in its efficiency and simplicity without any structure change, making it lightweight and highly efficient.
Via
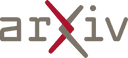
Jun 06, 2025
Abstract:End-to-end (E2E) Automatic Speech Recognition (ASR) models are trained using paired audio-text samples that are expensive to obtain, since high-quality ground-truth data requires human annotators. Voice search applications, such as digital media players, leverage ASR to allow users to search by voice as opposed to an on-screen keyboard. However, recent or infrequent movie titles may not be sufficiently represented in the E2E ASR system's training data, and hence, may suffer poor recognition. In this paper, we propose a phonetic correction system that consists of (a) a phonetic search based on the ASR model's output that generates phonetic alternatives that may not be considered by the E2E system, and (b) a rescorer component that combines the ASR model recognition and the phonetic alternatives, and select a final system output. We find that our approach improves word error rate between 4.4 and 7.6% relative on benchmarks of popular movie titles over a series of competitive baselines.
* To appear at Interspeech '25
Via
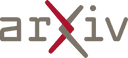
Jun 16, 2025
Abstract:Dynamic facial emotion is essential for believable AI-generated avatars; however, most systems remain visually inert, limiting their utility in high-stakes simulations such as virtual training for investigative interviews with abused children. We introduce and evaluate a real-time architecture fusing Unreal Engine 5 MetaHuman rendering with NVIDIA Omniverse Audio2Face to translate vocal prosody into high-fidelity facial expressions on photorealistic child avatars. We implemented a distributed two-PC setup that decouples language processing and speech synthesis from GPU-intensive rendering, designed to support low-latency interaction in desktop and VR environments. A between-subjects study ($N=70$) using audio+visual and visual-only conditions assessed perceptual impacts as participants rated emotional clarity, facial realism, and empathy for two avatars expressing joy, sadness, and anger. Results demonstrate that avatars could express emotions recognizably, with sadness and joy achieving high identification rates. However, anger recognition significantly dropped without audio, highlighting the importance of congruent vocal cues for high-arousal emotions. Interestingly, removing audio boosted perceived facial realism, suggesting that audiovisual desynchrony remains a key design challenge. These findings confirm the technical feasibility of generating emotionally expressive avatars and provide guidance for improving non-verbal communication in sensitive training simulations.
* 15 pages, 4 figures, 4 tables
Via
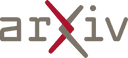
Jun 05, 2025
Abstract:We introduce LESS (Large Language Model Enhanced Semi-supervised Learning), a versatile framework that leverages Large Language Models (LLMs) to correct pseudo labels generated from in-the-wild data. Within the LESS framework, pseudo-labeled text from Automatic Speech Recognition (ASR) or Automatic Speech Translation (AST) of the unsupervised data is refined by an LLM, and augmented by a data filtering strategy to optimize LLM knowledge transfer efficiency. Experiments on both Mandarin ASR and Spanish-to-English AST tasks show that LESS achieves a notable absolute WER reduction of 3.77% on the Wenet Speech test set, as well as BLEU scores of 34.0 and 64.7 on Callhome and Fisher test sets respectively. These results validate the adaptability of LESS across different languages, tasks, and domains. Ablation studies conducted with various LLMs and prompt configurations provide novel insights into leveraging LLM-derived knowledge for speech processing applications.
Via
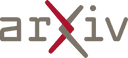
Jun 04, 2025
Abstract:To build an automatic speech recognition (ASR) system that can serve everyone in the world, the ASR needs to be robust to a wide range of accents including unseen accents. We systematically study how three different variables in training data -- the number of speakers, the audio duration per each individual speaker, and the diversity of accents -- affect ASR robustness towards unseen accents in a low-resource training regime. We observe that for a fixed number of ASR training hours, it is more beneficial to increase the number of speakers (which means each speaker contributes less) than the number of hours contributed per speaker. We also observe that more speakers enables ASR performance gains from scaling number of hours. Surprisingly, we observe minimal benefits to prioritizing speakers with different accents when the number of speakers is controlled. Our work suggests that practitioners should prioritize increasing the speaker count in ASR training data composition for new languages.
* Accepted to INTERSPEECH 2025
Via
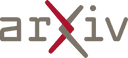
Jun 04, 2025
Abstract:Multi-state spiking neurons such as the adaptive leaky integrate-and-fire (AdLIF) neuron offer compelling alternatives to conventional deep learning models thanks to their sparse binary activations, second-order nonlinear recurrent dynamics, and efficient hardware realizations. However, such internal dynamics can cause instabilities during inference and training, often limiting performance and scalability. Meanwhile, state space models (SSMs) excel in long sequence processing using linear state-intrinsic recurrence resembling spiking neurons' subthreshold regime. Here, we establish a mathematical bridge between SSMs and second-order spiking neuron models. Based on structure and parametrization strategies of diagonal SSMs, we propose two novel spiking neuron models. The first extends the AdLIF neuron through timestep training and logarithmic reparametrization to facilitate training and improve final performance. The second additionally brings initialization and structure from complex-state SSMs, broadening the dynamical regime to oscillatory dynamics. Together, our two models achieve beyond or near state-of-the-art (SOTA) performances for reset-based spiking neuron models across both event-based and raw audio speech recognition datasets. We achieve this with a favorable number of parameters and required dynamic memory while maintaining high activity sparsity. Our models demonstrate enhanced scalability in network size and strike a favorable balance between performance and efficiency with respect to SSM models.
Via
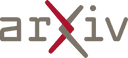
Jun 05, 2025
Abstract:The rapid advancement of deep generative models has significantly improved the realism of synthetic media, presenting both opportunities and security challenges. While deepfake technology has valuable applications in entertainment and accessibility, it has emerged as a potent vector for misinformation campaigns, particularly on social media. Existing detection frameworks struggle to distinguish between benign and adversarially generated deepfakes engineered to manipulate public perception. To address this challenge, we introduce SocialDF, a curated dataset reflecting real-world deepfake challenges on social media platforms. This dataset encompasses high-fidelity deepfakes sourced from various online ecosystems, ensuring broad coverage of manipulative techniques. We propose a novel LLM-based multi-factor detection approach that combines facial recognition, automated speech transcription, and a multi-agent LLM pipeline to cross-verify audio-visual cues. Our methodology emphasizes robust, multi-modal verification techniques that incorporate linguistic, behavioral, and contextual analysis to effectively discern synthetic media from authentic content.
Via
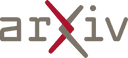