What is music generation? Music generation is the task of generating music or music-like sounds from a model or algorithm.
Papers and Code
May 26, 2025
Abstract:Quantum computing can be employed in computer-aided music composition to control various attributes of the music at different structural levels. This article describes the application of quantum simulation to model compositional decision making, the simulation of quantum particle tracking to produce noise-based timbres, the use of basis state vector rotation to cause changing probabilistic behaviors in granular harmonic textures, and the exploitation of quantum measurement error to cause noisy perturbations of spatial soundpaths. We describe the concepts fundamental to these techniques, we provide algorithms and software enacting them, and we provide examples demonstrating their implementation in computer-generated music.
Via
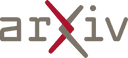
May 19, 2025
Abstract:We present Text2midi-InferAlign, a novel technique for improving symbolic music generation at inference time. Our method leverages text-to-audio alignment and music structural alignment rewards during inference to encourage the generated music to be consistent with the input caption. Specifically, we introduce two objectives scores: a text-audio consistency score that measures rhythmic alignment between the generated music and the original text caption, and a harmonic consistency score that penalizes generated music containing notes inconsistent with the key. By optimizing these alignment-based objectives during the generation process, our model produces symbolic music that is more closely tied to the input captions, thereby improving the overall quality and coherence of the generated compositions. Our approach can extend any existing autoregressive model without requiring further training or fine-tuning. We evaluate our work on top of Text2midi - an existing text-to-midi generation model, demonstrating significant improvements in both objective and subjective evaluation metrics.
* 7 pages, 1 figure, 5 tables
Via
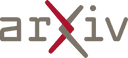
May 26, 2025
Abstract:Computational dance generation is crucial in many areas, such as art, human-computer interaction, virtual reality, and digital entertainment, particularly for generating coherent and expressive long dance sequences. Diffusion-based music-to-dance generation has made significant progress, yet existing methods still struggle to produce physically plausible motions. To address this, we propose Plausibility-Aware Motion Diffusion (PAMD), a framework for generating dances that are both musically aligned and physically realistic. The core of PAMD lies in the Plausible Motion Constraint (PMC), which leverages Neural Distance Fields (NDFs) to model the actual pose manifold and guide generated motions toward a physically valid pose manifold. To provide more effective guidance during generation, we incorporate Prior Motion Guidance (PMG), which uses standing poses as auxiliary conditions alongside music features. To further enhance realism for complex movements, we introduce the Motion Refinement with Foot-ground Contact (MRFC) module, which addresses foot-skating artifacts by bridging the gap between the optimization objective in linear joint position space and the data representation in nonlinear rotation space. Extensive experiments show that PAMD significantly improves musical alignment and enhances the physical plausibility of generated motions. This project page is available at: https://mucunzhuzhu.github.io/PAMD-page/.
Via
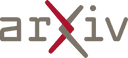
May 21, 2025
Abstract:Moonbeam is a transformer-based foundation model for symbolic music, pretrained on a large and diverse collection of MIDI data totaling 81.6K hours of music and 18 billion tokens. Moonbeam incorporates music-domain inductive biases by capturing both absolute and relative musical attributes through the introduction of a novel domain-knowledge-inspired tokenization method and Multidimensional Relative Attention (MRA), which captures relative music information without additional trainable parameters. Leveraging the pretrained Moonbeam, we propose 2 finetuning architectures with full anticipatory capabilities, targeting 2 categories of downstream tasks: symbolic music understanding and conditional music generation (including music infilling). Our model outperforms other large-scale pretrained music models in most cases in terms of accuracy and F1 score across 3 downstream music classification tasks on 4 datasets. Moreover, our finetuned conditional music generation model outperforms a strong transformer baseline with a REMI-like tokenizer. We open-source the code, pretrained model, and generated samples on Github.
Via
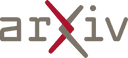
May 23, 2025
Abstract:Music-driven 3D dance generation has attracted increasing attention in recent years, with promising applications in choreography, virtual reality, and creative content creation. Previous research has generated promising realistic dance movement from audio signals. However, traditional methods underutilize genre conditioning, often treating it as auxiliary modifiers rather than core semantic drivers. This oversight compromises music-motion synchronization and disrupts dance genre continuity, particularly during complex rhythmic transitions, thereby leading to visually unsatisfactory effects. To address the challenge, we propose MEGADance, a novel architecture for music-driven 3D dance generation. By decoupling choreographic consistency into dance generality and genre specificity, MEGADance demonstrates significant dance quality and strong genre controllability. It consists of two stages: (1) High-Fidelity Dance Quantization Stage (HFDQ), which encodes dance motions into a latent representation by Finite Scalar Quantization (FSQ) and reconstructs them with kinematic-dynamic constraints, and (2) Genre-Aware Dance Generation Stage (GADG), which maps music into the latent representation by synergistic utilization of Mixture-of-Experts (MoE) mechanism with Mamba-Transformer hybrid backbone. Extensive experiments on the FineDance and AIST++ dataset demonstrate the state-of-the-art performance of MEGADance both qualitatively and quantitatively. Code will be released upon acceptance.
* arXiv admin note: text overlap with arXiv:2505.14222
Via
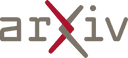
May 21, 2025
Abstract:Music-to-dance generation represents a challenging yet pivotal task at the intersection of choreography, virtual reality, and creative content generation. Despite its significance, existing methods face substantial limitation in achieving choreographic consistency. To address the challenge, we propose MatchDance, a novel framework for music-to-dance generation that constructs a latent representation to enhance choreographic consistency. MatchDance employs a two-stage design: (1) a Kinematic-Dynamic-based Quantization Stage (KDQS), which encodes dance motions into a latent representation by Finite Scalar Quantization (FSQ) with kinematic-dynamic constraints and reconstructs them with high fidelity, and (2) a Hybrid Music-to-Dance Generation Stage(HMDGS), which uses a Mamba-Transformer hybrid architecture to map music into the latent representation, followed by the KDQS decoder to generate 3D dance motions. Additionally, a music-dance retrieval framework and comprehensive metrics are introduced for evaluation. Extensive experiments on the FineDance dataset demonstrate state-of-the-art performance. Code will be released upon acceptance.
Via
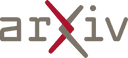
May 29, 2025
Abstract:Recent advances in interactive technologies have highlighted the prominence of audio signals for semantic encoding. This paper explores a new task, where audio signals are used as conditioning inputs to generate motions that align with the semantics of the audio. Unlike text-based interactions, audio provides a more natural and intuitive communication method. However, existing methods typically focus on matching motions with music or speech rhythms, which often results in a weak connection between the semantics of the audio and generated motions. We propose an end-to-end framework using a masked generative transformer, enhanced by a memory-retrieval attention module to handle sparse and lengthy audio inputs. Additionally, we enrich existing datasets by converting descriptions into conversational style and generating corresponding audio with varied speaker identities. Experiments demonstrate the effectiveness and efficiency of the proposed framework, demonstrating that audio instructions can convey semantics similar to text while providing more practical and user-friendly interactions.
* Graphical Models,Volume 139,2025,101268,ISSN 1524-0703,
Via
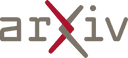
May 27, 2025
Abstract:In music production, manipulating audio effects (Fx) parameters through natural language has the potential to reduce technical barriers for non-experts. We present LLM2Fx, a framework leveraging Large Language Models (LLMs) to predict Fx parameters directly from textual descriptions without requiring task-specific training or fine-tuning. Our approach address the text-to-effect parameter prediction (Text2Fx) task by mapping natural language descriptions to the corresponding Fx parameters for equalization and reverberation. We demonstrate that LLMs can generate Fx parameters in a zero-shot manner that elucidates the relationship between timbre semantics and audio effects in music production. To enhance performance, we introduce three types of in-context examples: audio Digital Signal Processing (DSP) features, DSP function code, and few-shot examples. Our results demonstrate that LLM-based Fx parameter generation outperforms previous optimization approaches, offering competitive performance in translating natural language descriptions to appropriate Fx settings. Furthermore, LLMs can serve as text-driven interfaces for audio production, paving the way for more intuitive and accessible music production tools.
* Submitted to WASPAA 2025
Via
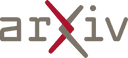
May 19, 2025
Abstract:Reservoir computing is a form of machine learning particularly suited for time series analysis, including forecasting predictions. We take an implementation of \emph{quantum} reservoir computing that was initially designed to generate variants of musical scores and adapt it to create levels of Super Mario Bros. Motivated by our analysis of these levels, we develop a new Roblox \textit{obby} where the courses can be generated in real time on superconducting qubit hardware, and investigate some of the constraints placed by such real-time generation.
Via
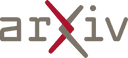
May 19, 2025
Abstract:Music exists in various modalities, such as score images, symbolic scores, MIDI, and audio. Translations between each modality are established as core tasks of music information retrieval, such as automatic music transcription (audio-to-MIDI) and optical music recognition (score image to symbolic score). However, most past work on multimodal translation trains specialized models on individual translation tasks. In this paper, we propose a unified approach, where we train a general-purpose model on many translation tasks simultaneously. Two key factors make this unified approach viable: a new large-scale dataset and the tokenization of each modality. Firstly, we propose a new dataset that consists of more than 1,300 hours of paired audio-score image data collected from YouTube videos, which is an order of magnitude larger than any existing music modal translation datasets. Secondly, our unified tokenization framework discretizes score images, audio, MIDI, and MusicXML into a sequence of tokens, enabling a single encoder-decoder Transformer to tackle multiple cross-modal translation as one coherent sequence-to-sequence task. Experimental results confirm that our unified multitask model improves upon single-task baselines in several key areas, notably reducing the symbol error rate for optical music recognition from 24.58% to a state-of-the-art 13.67%, while similarly substantial improvements are observed across the other translation tasks. Notably, our approach achieves the first successful score-image-conditioned audio generation, marking a significant breakthrough in cross-modal music generation.
* Submitted to IEEE Transactions on Audio, Speech and Language
Processing (TASLPRO)
Via
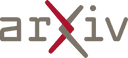