What is music generation? Music generation is the task of generating music or music-like sounds from a model or algorithm.
Papers and Code
Jan 07, 2025
Abstract:Guitar-related machine listening research involves tasks like timbre transfer, performance generation, and automatic transcription. However, small datasets often limit model robustness due to insufficient acoustic diversity and musical content. To address these issues, we introduce Guitar-TECHS, a comprehensive dataset featuring a variety of guitar techniques, musical excerpts, chords, and scales. These elements are performed by diverse musicians across various recording settings. Guitar-TECHS incorporates recordings from two stereo microphones: an egocentric microphone positioned on the performer's head and an exocentric microphone placed in front of the performer. It also includes direct input recordings and microphoned amplifier outputs, offering a wide spectrum of audio inputs and recording qualities. All signals and MIDI labels are properly synchronized. Its multi-perspective and multi-modal content makes Guitar-TECHS a valuable resource for advancing data-driven guitar research, and to develop robust guitar listening algorithms. We provide empirical data to demonstrate the dataset's effectiveness in training robust models for Guitar Tablature Transcription.
* IEEE International Conference on Acoustics, Speech and Signal
Processing (ICASSP) 2025
Via
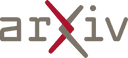
Dec 14, 2024
Abstract:We present a study that explores the role of user-centred design in developing Generative AI (GenAI) tools for music composition. Through semi-structured interviews with professional composers, we gathered insights on a novel generative model for creating variations, highlighting concerns around trust, transparency, and ethical design. The findings helped form a feedback loop, guiding improvements to the model that emphasised traceability, transparency and explainability. They also revealed new areas for innovation, including novel features for controllability and research questions on the ethical and practical implementation of GenAI models.
* Accepted to NeurIPS 2024 Workshop on Generative AI and Creativity: A
dialogue between machine learning researchers and creative professionals in
Vancouver, Canada
Via
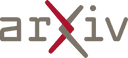
Dec 16, 2024
Abstract:We propose WHISPER-GPT: A generative large language model (LLM) for speech and music that allows us to work with continuous audio representations and discrete tokens simultaneously as part of a single architecture. There has been a huge surge in generative audio, speech, and music models that utilize discrete audio tokens derived from neural compression algorithms, e.g. ENCODEC. However, one of the major drawbacks of this approach is handling the context length. It blows up for high-fidelity generative architecture if one has to account for all the audio contents at various frequencies for the next token prediction. By combining continuous audio representation like the spectrogram and discrete acoustic tokens, we retain the best of both worlds: Have all the information needed from the audio at a specific time instance in a single token, yet allow LLM to predict the future token to allow for sampling and other benefits discrete space provides. We show how our architecture improves the perplexity and negative log-likelihood scores for the next token prediction compared to a token-based LLM for speech and music.
* 6 pages, 3 figures. 50th International Conference on Acoustics,
Speech and Signal Processing, Hyderabad, India
Via
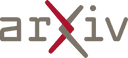
Jan 25, 2025
Abstract:Artificial intelligence is reshaping creative domains, yet its co-creative processes, especially in group settings with novice users, remain under explored. To bridge this gap, we conducted a case study in a college-level course where nine undergraduate students were tasked with creating three original music tracks using AI tools over 10 weeks. The study spanned the entire creative journey from ideation to releasing these songs on Spotify. Participants leveraged AI for music and lyric production, cover art, and distribution. Our findings highlight how AI transforms creative workflows: accelerating ideation but compressing the traditional preparation stage, and requiring novices to navigate a challenging idea selection and validation phase. We also identified a new "collaging and refinement" stage, where participants creatively combined diverse AI-generated outputs into cohesive works. Furthermore, AI influenced group social dynamics and role division among human creators. Based on these insights, we propose the Human-AI Co-Creation Stage Model and the Human-AI Agency Model, offering new perspectives on collaborative co-creation with AI.
Via
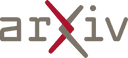
Feb 05, 2025
Abstract:Movable antennas (MAs) show great promise for enhancing the sensing capabilities of future sixth-generation (6G) networks. With the growing prevalence of near-field propagation at ultra-high frequencies, this paper focuses on the application of MAs for near-field sensing to jointly estimate the angle and distance information of a target. First, to gain essential insights into MA-enhanced near-field sensing, we investigate two simplified cases with only the spatial angle-of-arrival (AoA) or distance estimation, respectively, assuming that the other information is already known. We derive the worst-case Cramer-Rao bounds (CRBs) on the mean square errors (MSEs) of the AoA estimation and the distance estimation via the multiple signal classification (MUSIC) algorithm in these two cases. Then, we jointly optimize the positions of the MAs within a linear array to minimize these CRBs and derive their closed-form solutions, which yield an identical array geometry to MA-aided far-field sensing. Furthermore, we proceed to the more challenging case with the joint AoA and distance estimation and derive the worst-case CRB under the two-dimensional (2D) MUSIC algorithm. The corresponding CRB minimization problem is efficiently solved by adopting a discrete sampling-based approach. Numerical results demonstrate that the proposed MA-enhanced near-field sensing significantly outperforms conventional sensing with fixed-position antennas (FPAs). Moreover, the joint angle and distance estimation results in a different array geometry from that in the individual estimation of angle or distance.
Via
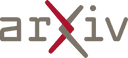
Feb 04, 2025
Abstract:This paper considers an intelligent reflecting surface (IRS)-assisted bi-static localization architecture for the sixth-generation (6G) integrated sensing and communication (ISAC) network. The system consists of a transmit user, a receive base station (BS), an IRS, and multiple targets in either the far-field or near-field region of the IRS. In particular, we focus on the challenging scenario where the line-of-sight (LOS) paths between targets and the BS are blocked, such that the emitted orthogonal frequency division multiplexing (OFDM) signals from the user reach the BS merely via the user-target-IRS-BS path. Based on the signals received by the BS, our goal is to localize the targets by estimating their relative positions to the IRS, instead of to the BS. We show that subspace-based methods, such as the multiple signal classification (MUSIC) algorithm, can be applied onto the BS's received signals to estimate the relative states from the targets to the IRS. To this end, we create a virtual signal via combining user-target-IRS-BS channels over various time slots. By applying MUSIC on such a virtual signal, we are able to detect the far-field targets and the near-field targets, and estimate the angle-of-arrivals (AOAs) and/or ranges from the targets to the IRS. Furthermore, we theoretically verify that the proposed method can perfectly estimate the relative states from the targets to the IRS in the ideal case with infinite coherence blocks. Numerical results verify the effectiveness of our proposed IRS-assisted localization scheme. Our paper demonstrates the potential of employing passive anchors, i.e., IRSs, to improve the sensing coverage of the active anchors, i.e., BSs.
Via
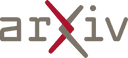
Dec 25, 2024
Abstract:Contrastive learning has proven effective in self-supervised musical representation learning, particularly for Music Information Retrieval (MIR) tasks. However, reliance on augmentation chains for contrastive view generation and the resulting learnt invariances pose challenges when different downstream tasks require sensitivity to certain musical attributes. To address this, we propose the Leave One EquiVariant (LOEV) framework, which introduces a flexible, task-adaptive approach compared to previous work by selectively preserving information about specific augmentations, allowing the model to maintain task-relevant equivariances. We demonstrate that LOEV alleviates information loss related to learned invariances, improving performance on augmentation related tasks and retrieval without sacrificing general representation quality. Furthermore, we introduce a variant of LOEV, LOEV++, which builds a disentangled latent space by design in a self-supervised manner, and enables targeted retrieval based on augmentation related attributes.
Via
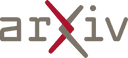
Dec 18, 2024
Abstract:The emergence of novel generative modeling paradigms, particularly audio language models, has significantly advanced the field of song generation. Although state-of-the-art models are capable of synthesizing both vocals and accompaniment tracks up to several minutes long concurrently, research about partial adjustments or editing of existing songs is still underexplored, which allows for more flexible and effective production. In this paper, we present SongEditor, the first song editing paradigm that introduces the editing capabilities into language-modeling song generation approaches, facilitating both segment-wise and track-wise modifications. SongEditor offers the flexibility to adjust lyrics, vocals, and accompaniments, as well as synthesizing songs from scratch. The core components of SongEditor include a music tokenizer, an autoregressive language model, and a diffusion generator, enabling generating an entire section, masked lyrics, or even separated vocals and background music. Extensive experiments demonstrate that the proposed SongEditor achieves exceptional performance in end-to-end song editing, as evidenced by both objective and subjective metrics. Audio samples are available in \url{https://cypress-yang.github.io/SongEditor_demo/}.
* Accepted by AAAI2025
Via
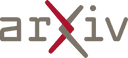
Jan 09, 2025
Abstract:Diffusion models have been widely used in the generative domain due to their convincing performance in modeling complex data distributions. Moreover, they have shown competitive results on discriminative tasks, such as image segmentation. While diffusion models have also been explored for automatic music transcription, their performance has yet to reach a competitive level. In this paper, we focus on discrete diffusion model's refinement capabilities and present a novel architecture for piano transcription. Our model utilizes Neighborhood Attention layers as the denoising module, gradually predicting the target high-resolution piano roll, conditioned on the finetuned features of a pretrained acoustic model. To further enhance refinement, we devise a novel strategy which applies distinct transition states during training and inference stage of discrete diffusion models. Experiments on the MAESTRO dataset show that our approach outperforms previous diffusion-based piano transcription models and the baseline model in terms of F1 score. Our code is available in https://github.com/hanshounsu/d3rm.
* Accepted to ICASSP 2025
Via
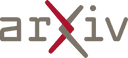
Jan 03, 2025
Abstract:Recent years have witnessed the success of foundation models pre-trained with self-supervised learning (SSL) in various music informatics understanding tasks, including music tagging, instrument classification, key detection, and more. In this paper, we propose a self-supervised music representation learning model for music understanding. Distinguished from previous studies adopting random projection or existing neural codec, the proposed model, named MuQ, is trained to predict tokens generated by Mel Residual Vector Quantization (Mel-RVQ). Our Mel-RVQ utilizes residual linear projection structure for Mel spectrum quantization to enhance the stability and efficiency of target extraction and lead to better performance. Experiments in a large variety of downstream tasks demonstrate that MuQ outperforms previous self-supervised music representation models with only 0.9K hours of open-source pre-training data. Scaling up the data to over 160K hours and adopting iterative training consistently improve the model performance. To further validate the strength of our model, we present MuQ-MuLan, a joint music-text embedding model based on contrastive learning, which achieves state-of-the-art performance in the zero-shot music tagging task on the MagnaTagATune dataset. Code and checkpoints are open source in https://github.com/tencent-ailab/MuQ.
Via
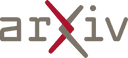