Mar 11, 2025
Abstract:The widespread availability of large language models (LLMs), such as ChatGPT, has significantly impacted education, raising both opportunities and challenges. Students can frequently interact with LLM-powered, interactive learning tools, but their usage patterns need to be analyzed to ensure ethical usage of these tools. To better understand how students interact with LLMs in an academic setting, we introduce \textbf{StudyChat}, a publicly available dataset capturing real-world student interactions with an LLM-powered tutoring chatbot in a semester-long, university-level artificial intelligence (AI) course. We deploy a web application that replicates ChatGPT's core functionalities, and use it to log student interactions with the LLM while working on programming assignments. We collect 1,197 conversations, which we annotate using a dialogue act labeling schema inspired by observed interaction patterns and prior research. Additionally, we analyze these interactions, highlight behavioral trends, and analyze how specific usage patterns relate to course outcomes. \textbf{StudyChat} provides a rich resource for the learning sciences and AI in education communities, enabling further research into the evolving role of LLMs in education.
* Pre-print
Via
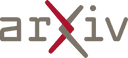