Jun 16, 2025
Abstract:Recent advancements in diffusion-based text-to-image (T2I) models have enabled the generation of high-quality and photorealistic images from text descriptions. However, they often exhibit societal biases related to gender, race, and socioeconomic status, thereby reinforcing harmful stereotypes and shaping public perception in unintended ways. While existing bias mitigation methods demonstrate effectiveness, they often encounter attribute entanglement, where adjustments to attributes relevant to the bias (i.e., target attributes) unintentionally alter attributes unassociated with the bias (i.e., non-target attributes), causing undesirable distribution shifts. To address this challenge, we introduce Entanglement-Free Attention (EFA), a method that accurately incorporates target attributes (e.g., White, Black, Asian, and Indian) while preserving non-target attributes (e.g., background details) during bias mitigation. At inference time, EFA randomly samples a target attribute with equal probability and adjusts the cross-attention in selected layers to incorporate the sampled attribute, achieving a fair distribution of target attributes. Extensive experiments demonstrate that EFA outperforms existing methods in mitigating bias while preserving non-target attributes, thereby maintaining the output distribution and generation capability of the original model.
Via
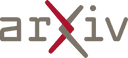