Topic:Text Classification
What is Text Classification? Text classification is the process of categorizing text documents into predefined categories or labels.
Papers and Code
May 05, 2025
Abstract:Identifying the finer details of a book's genres enhances user experience by enabling efficient book discovery and personalized recommendations, ultimately improving reader engagement and satisfaction. It also provides valuable insights into market trends and consumer preferences, allowing publishers and marketers to make data-driven decisions regarding book production and marketing strategies. While traditional book genre classification methods primarily rely on review data or textual analysis, incorporating additional modalities, such as book covers, blurbs, and metadata, can offer richer context and improve prediction accuracy. However, the presence of incomplete or noisy information across these modalities presents a significant challenge. This paper introduces IMAGINE (Intelligent Multi-modal Adaptive Genre Identification NEtwork), a framework designed to address these complexities. IMAGINE extracts robust feature representations from multiple modalities and dynamically selects the most informative sources based on data availability. It employs a hierarchical classification strategy to capture genre relationships and remains adaptable to varying input conditions. Additionally, we curate a hierarchical genre classification dataset that structures genres into a well-defined taxonomy, accommodating the diverse nature of literary works. IMAGINE integrates information from multiple sources and assigns multiple genre labels to each book, ensuring a more comprehensive classification. A key feature of our framework is its resilience to incomplete data, enabling accurate predictions even when certain modalities, such as text, images, or metadata, are missing or incomplete. Experimental results show that IMAGINE outperformed existing baselines in genre classification accuracy, particularly in scenarios with insufficient modality-specific data.
Via
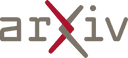
May 06, 2025
Abstract:Vision-language models (VLMs) allow to embed texts and images in a shared representation space. However, it has been shown that these models are subject to a modality gap phenomenon meaning there exists a clear separation between the embeddings from one modality and another in the embedding space. While this misalignment is detrimental for downstream tasks such as multimodal retrieval, multimodal clustering or zero-shot classification, etc. no generic and practical methods have so far been proposed to assess it precisely and even reduce it. We therefore propose novel measures and effective techniques (spectral- and optimal transport-based methods) to achieve this goal. Extensive experiments conducted on several image-text datasets and models demonstrate their effectiveness and beneficial effects on downstream tasks. Our code is available at the URL provided in the paper's abstract.
Via
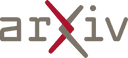
May 05, 2025
Abstract:Second language proficiency (L2) in English is usually perceptually evaluated by English teachers or expert evaluators, with the inherent intra- and inter-rater variability. This paper explores deep learning techniques for comprehensive L2 proficiency assessment, addressing both the speech signal and its correspondent transcription. We analyze spoken proficiency classification prediction using diverse architectures, including 2D CNN, frequency-based CNN, ResNet, and a pretrained wav2vec 2.0 model. Additionally, we examine text-based proficiency assessment by fine-tuning a BERT language model within resource constraints. Finally, we tackle the complex task of spontaneous dialogue assessment, managing long-form audio and speaker interactions through separate applications of wav2vec 2.0 and BERT models. Results from experiments on EFCamDat and ANGLISH datasets and a private dataset highlight the potential of deep learning, especially the pretrained wav2vec 2.0 model, for robust automated L2 proficiency evaluation.
* 6 pages
Via
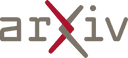
May 06, 2025
Abstract:Social Determinants of Health (SDoH) are economic, social and personal circumstances that affect or influence an individual's health status. SDoHs have shown to be correlated to wellness outcomes, and therefore, are useful to physicians in diagnosing diseases and in decision-making. In this work, we automatically extract SDoHs from clinical text using traditional deep learning and Large Language Models (LLMs) to find the advantages and disadvantages of each on an existing publicly available dataset. Our models outperform a previous reference point on a multilabel SDoH classification by 10 points, and we present a method and model to drastically speed up classification (12X execution time) by eliminating expensive LLM processing. The method we present combines a more nimble and efficient solution that leverages the power of the LLM for precision and traditional deep learning methods for efficiency. We also show highly performant results on a dataset supplemented with synthetic data and several traditional deep learning models that outperform LLMs. Our models and methods offer the next iteration of automatic prediction of SDoHs that impact at-risk patients.
Via
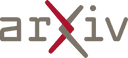
May 09, 2025
Abstract:Medical vision-language models (VLMs) have shown promise as clinical assistants across various medical fields. However, specialized dermatology VLM capable of delivering professional and detailed diagnostic analysis remains underdeveloped, primarily due to less specialized text descriptions in current dermatology multimodal datasets. To address this issue, we propose MM-Skin, the first large-scale multimodal dermatology dataset that encompasses 3 imaging modalities, including clinical, dermoscopic, and pathological and nearly 10k high-quality image-text pairs collected from professional textbooks. In addition, we generate over 27k diverse, instruction-following vision question answering (VQA) samples (9 times the size of current largest dermatology VQA dataset). Leveraging public datasets and MM-Skin, we developed SkinVL, a dermatology-specific VLM designed for precise and nuanced skin disease interpretation. Comprehensive benchmark evaluations of SkinVL on VQA, supervised fine-tuning (SFT) and zero-shot classification tasks across 8 datasets, reveal its exceptional performance for skin diseases in comparison to both general and medical VLM models. The introduction of MM-Skin and SkinVL offers a meaningful contribution to advancing the development of clinical dermatology VLM assistants. MM-Skin is available at https://github.com/ZwQ803/MM-Skin
Via
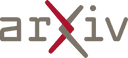
May 11, 2025
Abstract:Temporal medical image analysis is essential for clinical decision-making, yet existing methods either align images and text at a coarse level - causing potential semantic mismatches - or depend solely on visual information, lacking medical semantic integration. We present CheXLearner, the first end-to-end framework that unifies anatomical region detection, Riemannian manifold-based structure alignment, and fine-grained regional semantic guidance. Our proposed Med-Manifold Alignment Module (Med-MAM) leverages hyperbolic geometry to robustly align anatomical structures and capture pathologically meaningful discrepancies across temporal chest X-rays. By introducing regional progression descriptions as supervision, CheXLearner achieves enhanced cross-modal representation learning and supports dynamic low-level feature optimization. Experiments show that CheXLearner achieves 81.12% (+17.2%) average accuracy and 80.32% (+11.05%) F1-score on anatomical region progression detection - substantially outperforming state-of-the-art baselines, especially in structurally complex regions. Additionally, our model attains a 91.52% average AUC score in downstream disease classification, validating its superior feature representation.
Via
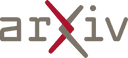
May 12, 2025
Abstract:Remarkable capabilities in understanding and generating text-image content have been demonstrated by recent advancements in multimodal large language models (MLLMs). However, their effectiveness in specialized domains-particularly those requiring resource-efficient and domain-specific adaptations-has remained limited. In this work, a lightweight multimodal language model termed MilChat is introduced, specifically adapted to analyze remote sensing imagery in secluded areas, including challenging missile launch sites. A new dataset, MilData, was compiled by verifying hundreds of aerial images through expert review, and subtle military installations were highlighted via detailed captions. Supervised fine-tuning on a 2B-parameter open-source MLLM with chain-of-thought (CoT) reasoning annotations was performed, enabling more accurate and interpretable explanations. Additionally, Group Relative Policy Optimization (GRPO) was leveraged to enhance the model's ability to detect critical domain-specific cues-such as defensive layouts and key military structures-while minimizing false positives on civilian scenes. Through empirical evaluations, it has been shown that MilChat significantly outperforms both larger, general-purpose multimodal models and existing remote sensing-adapted approaches on open-ended captioning and classification metrics. Over 80% recall and 98% precision were achieved on the newly proposed MilData benchmark, underscoring the potency of targeted fine-tuning and reinforcement learning in specialized real-world applications.
* Submitted to JSTARS on April 2, 2025. Code and dataset will be
available upon acceptance
Via
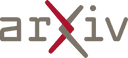
May 09, 2025
Abstract:Engagement between client and therapist is a critical determinant of therapeutic success. We propose a multi-dimensional natural language processing (NLP) framework that objectively classifies engagement quality in counseling sessions based on textual transcripts. Using 253 motivational interviewing transcripts (150 high-quality, 103 low-quality), we extracted 42 features across four domains: conversational dynamics, semantic similarity as topic alignment, sentiment classification, and question detection. Classifiers, including Random Forest (RF), Cat-Boost, and Support Vector Machines (SVM), were hyperparameter tuned and trained using a stratified 5-fold cross-validation and evaluated on a holdout test set. On balanced (non-augmented) data, RF achieved the highest classification accuracy (76.7%), and SVM achieved the highest AUC (85.4%). After SMOTE-Tomek augmentation, performance improved significantly: RF achieved up to 88.9% accuracy, 90.0% F1-score, and 94.6% AUC, while SVM reached 81.1% accuracy, 83.1% F1-score, and 93.6% AUC. The augmented data results reflect the potential of the framework in future larger-scale applications. Feature contribution revealed conversational dynamics and semantic similarity between clients and therapists were among the top contributors, led by words uttered by the client (mean and standard deviation). The framework was robust across the original and augmented datasets and demonstrated consistent improvements in F1 scores and recall. While currently text-based, the framework supports future multimodal extensions (e.g., vocal tone, facial affect) for more holistic assessments. This work introduces a scalable, data-driven method for evaluating engagement quality of the therapy session, offering clinicians real-time feedback to enhance the quality of both virtual and in-person therapeutic interactions.
* 12 pages, 4 figures, 7 tables
Via
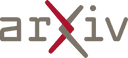
May 05, 2025
Abstract:The recent growth in the use of Large Language Models has made them vulnerable to sophisticated adversarial assaults, manipulative prompts, and encoded malicious inputs. Existing countermeasures frequently necessitate retraining models, which is computationally costly and impracticable for deployment. Without the need for retraining or fine-tuning, this study presents a unique defense paradigm that allows LLMs to recognize, filter, and defend against adversarial or malicious inputs on their own. There are two main parts to the suggested framework: (1) A prompt filtering module that uses sophisticated Natural Language Processing (NLP) techniques, including zero-shot classification, keyword analysis, and encoded content detection (e.g. base64, hexadecimal, URL encoding), to detect, decode, and classify harmful inputs; and (2) A summarization module that processes and summarizes adversarial research literature to give the LLM context-aware defense knowledge. This approach strengthens LLMs' resistance to adversarial exploitation by fusing text extraction, summarization, and harmful prompt analysis. According to experimental results, this integrated technique has a 98.71% success rate in identifying harmful patterns, manipulative language structures, and encoded prompts. By employing a modest amount of adversarial research literature as context, the methodology also allows the model to react correctly to harmful inputs with a larger percentage of jailbreak resistance and refusal rate. While maintaining the quality of LLM responses, the framework dramatically increases LLM's resistance to hostile misuse, demonstrating its efficacy as a quick and easy substitute for time-consuming, retraining-based defenses.
Via
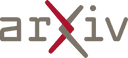
May 09, 2025
Abstract:Financial sentiment analysis (FSA) presents unique challenges to LLMs that surpass those in typical sentiment analysis due to the nuanced language used in financial contexts. The prowess of these models is often undermined by the inherent subjectivity of sentiment classifications in existing benchmark datasets like Financial Phrasebank. These datasets typically feature undefined sentiment classes that reflect the highly individualized perspectives of annotators, leading to significant variability in annotations. This variability results in an unfair expectation for LLMs during benchmarking, where they are tasked to conjecture the subjective viewpoints of human annotators without sufficient context. In this paper, we introduce the Annotators' Instruction Assisted Prompt, a novel evaluation prompt designed to redefine the task definition of FSA for LLMs. By integrating detailed task instructions originally intended for human annotators into the LLMs' prompt framework, AIAP aims to standardize the understanding of sentiment across both human and machine interpretations, providing a fair and context-rich foundation for sentiment analysis. We utilize a new dataset, WSBS, derived from the WallStreetBets subreddit to demonstrate how AIAP significantly enhances LLM performance by aligning machine operations with the refined task definitions. Experimental results demonstrate that AIAP enhances LLM performance significantly, with improvements up to 9.08. This context-aware approach not only yields incremental gains in performance but also introduces an innovative sentiment-indexing method utilizing model confidence scores. This method enhances stock price prediction models and extracts more value from the financial sentiment analysis, underscoring the significance of WSB as a critical source of financial text. Our research offers insights into both improving FSA through better evaluation methods.
Via
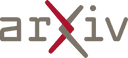