Topic:Information Extraction
What is Information Extraction? Information extraction is the process of automatically extracting structured information from unstructured text data.
Papers and Code
Jun 24, 2025
Abstract:Catastrophic forgetting can be trivially alleviated by keeping all data from previous tasks in memory. Therefore, minimizing the memory footprint while maximizing the amount of relevant information is crucial to the challenge of continual learning. This paper aims to decrease required memory for memory-based continuous learning algorithms. We explore the options of extracting a minimal amount of information, while maximally alleviating forgetting. We propose the usage of lightweight generators based on Singular Value Decomposition to enhance existing continual learning methods, such as A-GEM and Experience Replay. These generators need a minimal amount of memory while being maximally effective. They require no training time, just a single linear-time fitting step, and can capture a distribution effectively from a small number of data samples. Depending on the dataset and network architecture, our results show a significant increase in average accuracy compared to the original methods. Our method shows great potential in minimizing the memory footprint of memory-based continual learning algorithms.
Via
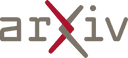
Jun 24, 2025
Abstract:Electrocardiogram (ECG), as a crucial find-grained cardiac feature, has been successfully recovered from radar signals in the literature, but the performance heavily relies on the high-quality radar signal and numerous radar-ECG pairs for training, restricting the applications in new scenarios due to data scarcity. Therefore, this work will focus on radar-based ECG recovery in new scenarios with limited data and propose a cardio-focusing and -tracking (CFT) algorithm to precisely track the cardiac location to ensure an efficient acquisition of high-quality radar signals. Furthermore, a transfer learning model (RFcardi) is proposed to extract cardio-related information from the radar signal without ECG ground truth based on the intrinsic sparsity of cardiac features, and only a few synchronous radar-ECG pairs are required to fine-tune the pre-trained model for the ECG recovery. The experimental results reveal that the proposed CFT can dynamically identify the cardiac location, and the RFcardi model can effectively generate faithful ECG recoveries after using a small number of radar-ECG pairs for training. The code and dataset are available after the publication.
Via
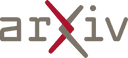
Jun 24, 2025
Abstract:Precision oncology requires accurate molecular insights, yet obtaining these directly from genomics is costly and time-consuming for broad clinical use. Predicting complex molecular features and patient prognosis directly from routine whole-slide images (WSI) remains a major challenge for current deep learning methods. Here we introduce PathLUPI, which uses transcriptomic privileged information during training to extract genome-anchored histological embeddings, enabling effective molecular prediction using only WSIs at inference. Through extensive evaluation across 49 molecular oncology tasks using 11,257 cases among 20 cohorts, PathLUPI demonstrated superior performance compared to conventional methods trained solely on WSIs. Crucially, it achieves AUC $\geq$ 0.80 in 14 of the biomarker prediction and molecular subtyping tasks and C-index $\geq$ 0.70 in survival cohorts of 5 major cancer types. Moreover, PathLUPI embeddings reveal distinct cellular morphological signatures associated with specific genotypes and related biological pathways within WSIs. By effectively encoding molecular context to refine WSI representations, PathLUPI overcomes a key limitation of existing models and offers a novel strategy to bridge molecular insights with routine pathology workflows for wider clinical application.
* Under Review
Via
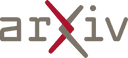
Jun 26, 2025
Abstract:Continuous sign language recognition (CSLR) aims to transcribe untrimmed videos into glosses, which are typically textual words. Recent studies indicate that the lack of large datasets and precise annotations has become a bottleneck for CSLR due to insufficient training data. To address this, some works have developed cross-modal solutions to align visual and textual modalities. However, they typically extract textual features from glosses without fully utilizing their knowledge. In this paper, we propose the Hierarchical Sub-action Tree (HST), termed HST-CSLR, to efficiently combine gloss knowledge with visual representation learning. By incorporating gloss-specific knowledge from large language models, our approach leverages textual information more effectively. Specifically, we construct an HST for textual information representation, aligning visual and textual modalities step-by-step and benefiting from the tree structure to reduce computational complexity. Additionally, we impose a contrastive alignment enhancement to bridge the gap between the two modalities. Experiments on four datasets (PHOENIX-2014, PHOENIX-2014T, CSL-Daily, and Sign Language Gesture) demonstrate the effectiveness of our HST-CSLR.
Via
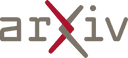
Jun 24, 2025
Abstract:Modern deep learning architectures excel at optimization, but only after the data has entered the network. The true bottleneck lies in preparing the right input: minimal, salient, and structured in a way that reflects the essential patterns of the data. We propose DRIFT (Data Reduction via Informative Feature Transformation), a novel preprocessing technique inspired by vibrational analysis in physical systems, to identify and extract the most resonant modes of input data prior to training. Unlike traditional models that attempt to learn amidst both signal and noise, DRIFT mimics physics perception by emphasizing informative features while discarding irrelevant elements. The result is a more compact and interpretable representation that enhances training stability and generalization performance. In DRIFT, images are projected onto a low-dimensional basis formed by spatial vibration mode shapes of plates, offering a physically grounded feature set. This enables neural networks to operate with drastically fewer input dimensions (~ 50 features on MNIST and less than 100 on CIFAR100) while achieving competitive classification accuracy. Extensive experiments across MNIST and CIFAR100 demonstrate DRIFT's superiority over standard pixel-based models and PCA in terms of training stability, resistance to overfitting, and generalization robustness. Notably, DRIFT displays minimal sensitivity to changes in batch size, network architecture, and image resolution, further establishing it as a resilient and efficient data representation strategy. This work shifts the focus from architecture engineering to input curation and underscores the power of physics-driven data transformations in advancing deep learning performance.
Via
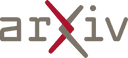
Jun 17, 2025
Abstract:Many recent approaches to structured NLP tasks use an autoregressive language model $M$ to map unstructured input text $x$ to output text $y$ representing structured objects (such as tuples, lists, trees, code, etc.), where the desired output structure is enforced via constrained decoding. During training, these approaches do not require the model to be aware of the constraints, which are merely implicit in the training outputs $y$. This is advantageous as it allows for dynamic constraints without requiring retraining, but can lead to low-quality output during constrained decoding at test time. We overcome this problem with Boosted Constrained Decoding (BoostCD), which combines constrained and unconstrained decoding in two phases: Phase 1 decodes from the base model $M$ twice, in constrained and unconstrained mode, obtaining two weak predictions. In phase 2, a learned autoregressive boosted model combines the two weak predictions into one final prediction. The mistakes made by the base model with vs. without constraints tend to be complementary, which the boosted model learns to exploit for improved performance. We demonstrate the power of BoostCD by applying it to closed information extraction. Our model, BoostIE, outperforms prior approaches both in and out of distribution, addressing several common errors identified in those approaches.
Via
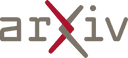
Jun 16, 2025
Abstract:Rules could be an information extraction (IE) default option, compared to ML and LLMs in terms of sustainability, transferability, interpretability, and development burden. We suggest a sustainable and combined use of rules and ML as an IE method. Our approach starts with an exhaustive expert manual highlighting in a single working session of a representative subset of the data corpus. We developed and validated the feasibility and the performance metrics of the REST decision tool to help the annotator choose between rules as a by default option and ML for each entity of an IE task. REST makes the annotator visualize the characteristics of each entity formalization in the free texts and the expected rule development feasibility and IE performance metrics. ML is considered as a backup IE option and manual annotation for training is therefore minimized. The external validity of REST on a 12-entity use case showed good reproducibility.
Via
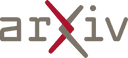
Jun 24, 2025
Abstract:Existing Mamba-based approaches in remote sensing change detection have enhanced scanning models, yet remain limited by their inability to capture long-range dependencies between image channels effectively, which restricts their feature representation capabilities. To address this limitation, we propose a 3D selective scan module (3D-SSM) that captures global information from both the spatial plane and channel perspectives, enabling a more comprehensive understanding of the data.Based on the 3D-SSM, we present two key components: a spatiotemporal interaction module (SIM) and a multi-branch feature extraction module (MBFEM). The SIM facilitates bi-temporal feature integration by enabling interactions between global and local features across images from different time points, thereby enhancing the detection of subtle changes. Meanwhile, the MBFEM combines features from the frequency domain, spatial domain, and 3D-SSM to provide a rich representation of contextual information within the image. Our proposed method demonstrates favourable performance compared to state-of-the-art change detection methods on five benchmark datasets through extensive experiments. Code is available at https://github.com/VerdantMist/3D-SSM
Via
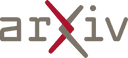
Jun 24, 2025
Abstract:Prostate gland segmentation from T2-weighted MRI is a critical yet challenging task in clinical prostate cancer assessment. While deep learning-based methods have significantly advanced automated segmentation, most conventional approaches-particularly 2D convolutional neural networks (CNNs)-fail to leverage inter-slice anatomical continuity, limiting their accuracy and robustness. Fully 3D models offer improved spatial coherence but require large amounts of annotated data, which is often impractical in clinical settings. To address these limitations, we propose a hybrid architecture that models MRI sequences as spatiotemporal data. Our method uses a deep, pretrained DeepLabV3 backbone to extract high-level semantic features from each MRI slice and a recurrent convolutional head, built with ConvLSTM layers, to integrate information across slices while preserving spatial structure. This combination enables context-aware segmentation with improved consistency, particularly in data-limited and noisy imaging conditions. We evaluate our method on the PROMISE12 benchmark under both clean and contrast-degraded test settings. Compared to state-of-the-art 2D and 3D segmentation models, our approach demonstrates superior performance in terms of precision, recall, Intersection over Union (IoU), and Dice Similarity Coefficient (DSC), highlighting its potential for robust clinical deployment.
Via
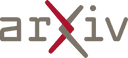
Jun 24, 2025
Abstract:Recent advances in deep learning for vision tasks have seen the rise of State Space Models (SSMs) like Mamba, celebrated for their linear scalability. However, their adaptation to 2D visual data often necessitates complex modifications that may diminish efficiency. In this paper, we introduce MambaOutRS, a novel hybrid convolutional architecture for remote sensing image classification that re-evaluates the necessity of recurrent SSMs. MambaOutRS builds upon stacked Gated CNN blocks for local feature extraction and introduces a novel Fourier Filter Gate (FFG) module that operates in the frequency domain to capture global contextual information efficiently. Our architecture employs a four-stage hierarchical design and was extensively evaluated on challenging remote sensing datasets: UC Merced, AID, NWPU-RESISC45, and EuroSAT. MambaOutRS consistently achieved state-of-the-art (SOTA) performance across these benchmarks. Notably, our MambaOutRS-t variant (24.0M parameters) attained the highest F1-scores of 98.41\% on UC Merced and 95.99\% on AID, significantly outperforming existing baselines, including larger transformer models and Mamba-based architectures, despite using considerably fewer parameters. An ablation study conclusively demonstrates the critical role of the Fourier Filter Gate in enhancing the model's ability to capture global spatial patterns, leading to robust and accurate classification. These results strongly suggest that the complexities of recurrent SSMs can be effectively superseded by a judicious combination of gated convolutions for spatial mixing and frequency-based gates for spectral global context. Thus, MambaOutRS provides a compelling and efficient paradigm for developing high-performance deep learning models in remote sensing and other vision domains, particularly where computational efficiency is paramount.
Via
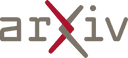