Topic:Image To Image Translation
What is Image To Image Translation? Image-to-image translation is the process of converting an image from one domain to another using deep learning techniques.
Papers and Code
Apr 04, 2025
Abstract:We introduce Diffusion Active Learning, a novel approach that combines generative diffusion modeling with data-driven sequential experimental design to adaptively acquire data for inverse problems. Although broadly applicable, we focus on scientific computed tomography (CT) for experimental validation, where structured prior datasets are available, and reducing data requirements directly translates to shorter measurement times and lower X-ray doses. We first pre-train an unconditional diffusion model on domain-specific CT reconstructions. The diffusion model acts as a learned prior that is data-dependent and captures the structure of the underlying data distribution, which is then used in two ways: It drives the active learning process and also improves the quality of the reconstructions. During the active learning loop, we employ a variant of diffusion posterior sampling to generate conditional data samples from the posterior distribution, ensuring consistency with the current measurements. Using these samples, we quantify the uncertainty in the current estimate to select the most informative next measurement. Our results show substantial reductions in data acquisition requirements, corresponding to lower X-ray doses, while simultaneously improving image reconstruction quality across multiple real-world tomography datasets.
Via
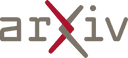
Mar 27, 2025
Abstract:Workflows are a fundamental component of automation in enterprise platforms, enabling the orchestration of tasks, data processing, and system integrations. Despite being widely used, building workflows can be complex, often requiring manual configuration through low-code platforms or visual programming tools. To simplify this process, we explore the use of generative foundation models, particularly vision-language models (VLMs), to automatically generate structured workflows from visual inputs. Translating hand-drawn sketches or computer-generated diagrams into executable workflows is challenging due to the ambiguity of free-form drawings, variations in diagram styles, and the difficulty of inferring execution logic from visual elements. To address this, we introduce StarFlow, a framework for generating structured workflow outputs from sketches using vision-language models. We curate a diverse dataset of workflow diagrams -- including synthetic, manually annotated, and real-world samples -- to enable robust training and evaluation. We finetune and benchmark multiple vision-language models, conducting a series of ablation studies to analyze the strengths and limitations of our approach. Our results show that finetuning significantly enhances structured workflow generation, outperforming large vision-language models on this task.
Via
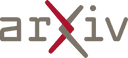
Mar 20, 2025
Abstract:Surgical automation requires precise guidance and understanding of the scene. Current methods in the literature rely on bulky depth cameras to create maps of the anatomy, however this does not translate well to space-limited clinical applications. Monocular cameras are small and allow minimally invasive surgeries in tight spaces but additional processing is required to generate 3D scene understanding. We propose a 3D mapping pipeline that uses only RGB images to create segmented point clouds of the target anatomy. To ensure the most precise reconstruction, we compare different structure from motion algorithms' performance on mapping the central airway obstructions, and test the pipeline on a downstream task of tumor resection. In several metrics, including post-procedure tissue model evaluation, our pipeline performs comparably to RGB-D cameras and, in some cases, even surpasses their performance. These promising results demonstrate that automation guidance can be achieved in minimally invasive procedures with monocular cameras. This study is a step toward the complete autonomy of surgical robots.
* 7 Pages, 8 Figures, 1 Table. This work has been submitted IEEE/RSJ
International Conference on Intelligent Robots and Systems (IROS) for
possible publication
Via
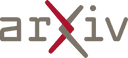
Mar 19, 2025
Abstract:Computed Tomography (CT) plays a pivotal role in medical diagnosis; however, variability across reconstruction kernels hinders data-driven approaches, such as deep learning models, from achieving reliable and generalized performance. To this end, CT data harmonization has emerged as a promising solution to minimize such non-biological variances by standardizing data across different sources or conditions. In this context, Generative Adversarial Networks (GANs) have proved to be a powerful framework for harmonization, framing it as a style-transfer problem. However, GAN-based approaches still face limitations in capturing complex relationships within the images, which are essential for effective harmonization. In this work, we propose a novel texture-aware StarGAN for CT data harmonization, enabling one-to-many translations across different reconstruction kernels. Although the StarGAN model has been successfully applied in other domains, its potential for CT data harmonization remains unexplored. Furthermore, our approach introduces a multi-scale texture loss function that embeds texture information across different spatial and angular scales into the harmonization process, effectively addressing kernel-induced texture variations. We conducted extensive experimentation on a publicly available dataset, utilizing a total of 48667 chest CT slices from 197 patients distributed over three different reconstruction kernels, demonstrating the superiority of our method over the baseline StarGAN.
Via
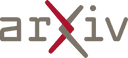
Mar 13, 2025
Abstract:With the rapid advancement of large language models (LLMs) and vision-language models (VLMs), significant progress has been made in developing open-vocabulary robotic manipulation systems. However, many existing approaches overlook the importance of object dynamics, limiting their applicability to more complex, dynamic tasks. In this work, we introduce KUDA, an open-vocabulary manipulation system that integrates dynamics learning and visual prompting through keypoints, leveraging both VLMs and learning-based neural dynamics models. Our key insight is that a keypoint-based target specification is simultaneously interpretable by VLMs and can be efficiently translated into cost functions for model-based planning. Given language instructions and visual observations, KUDA first assigns keypoints to the RGB image and queries the VLM to generate target specifications. These abstract keypoint-based representations are then converted into cost functions, which are optimized using a learned dynamics model to produce robotic trajectories. We evaluate KUDA on a range of manipulation tasks, including free-form language instructions across diverse object categories, multi-object interactions, and deformable or granular objects, demonstrating the effectiveness of our framework. The project page is available at http://kuda-dynamics.github.io.
Via
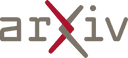
Mar 21, 2025
Abstract:In many robotics and VR/AR applications, fast camera motions cause a high level of motion blur, causing existing camera pose estimation methods to fail. In this work, we propose a novel framework that leverages motion blur as a rich cue for motion estimation rather than treating it as an unwanted artifact. Our approach works by predicting a dense motion flow field and a monocular depth map directly from a single motion-blurred image. We then recover the instantaneous camera velocity by solving a linear least squares problem under the small motion assumption. In essence, our method produces an IMU-like measurement that robustly captures fast and aggressive camera movements. To train our model, we construct a large-scale dataset with realistic synthetic motion blur derived from ScanNet++v2 and further refine our model by training end-to-end on real data using our fully differentiable pipeline. Extensive evaluations on real-world benchmarks demonstrate that our method achieves state-of-the-art angular and translational velocity estimates, outperforming current methods like MASt3R and COLMAP.
Via
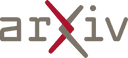
Mar 13, 2025
Abstract:Large language models (LLMs) have shown remarkable performance and generalization capabilities across multiple languages and tasks, making them very attractive targets for multi-modality integration (e.g., images or speech). In this work, we extend an existing LLM to the speech modality via speech discretization and continued pre-training. In particular, we are interested in multilingual LLMs, such as TOWER, as their pre-training setting allows us to treat discretized speech input as an additional translation language. The resulting open-source model, SPIRE, is able to transcribe and translate English speech input while maintaining TOWER's original performance on translation-related tasks, showcasing that discretized speech input integration as an additional language is feasible during LLM adaptation. We make our code and models available to the community.
Via
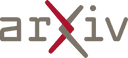
Mar 20, 2025
Abstract:Language models (LMs) are machine learning models designed to predict linguistic patterns by estimating the probability of word sequences based on large-scale datasets, such as text. LMs have a wide range of applications in natural language processing (NLP) tasks, including autocomplete and machine translation. Although larger datasets typically enhance LM performance, scalability remains a challenge due to constraints in computational power and resources. Distributed computing strategies offer essential solutions for improving scalability and managing the growing computational demand. Further, the use of sensitive datasets in training and deployment raises significant privacy concerns. Recent research has focused on developing decentralized techniques to enable distributed training and inference while utilizing diverse computational resources and enabling edge AI. This paper presents a survey on distributed solutions for various LMs, including large language models (LLMs), vision language models (VLMs), multimodal LLMs (MLLMs), and small language models (SLMs). While LLMs focus on processing and generating text, MLLMs are designed to handle multiple modalities of data (e.g., text, images, and audio) and to integrate them for broader applications. To this end, this paper reviews key advancements across the MLLM pipeline, including distributed training, inference, fine-tuning, and deployment, while also identifying the contributions, limitations, and future areas of improvement. Further, it categorizes the literature based on six primary focus areas of decentralization. Our analysis describes gaps in current methodologies for enabling distributed solutions for LMs and outline future research directions, emphasizing the need for novel solutions to enhance the robustness and applicability of distributed LMs.
Via
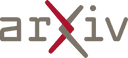
Mar 18, 2025
Abstract:Generating structured ASCII art using computational techniques demands a careful interplay between aesthetic representation and computational precision, requiring models that can effectively translate visual information into symbolic text characters. Although Convolutional Neural Networks (CNNs) have shown promise in this domain, the comparative performance of deep learning architectures and classical machine learning methods remains unexplored. This paper explores the application of contemporary ML and DL methods to generate structured ASCII art, focusing on three key criteria: fidelity, character classification accuracy, and output quality. We investigate deep learning architectures, including Multilayer Perceptrons (MLPs), ResNet, and MobileNetV2, alongside classical approaches such as Random Forests, Support Vector Machines (SVMs) and k-Nearest Neighbors (k-NN), trained on an augmented synthetic dataset of ASCII characters. Our results show that complex neural network architectures often fall short in producing high-quality ASCII art, whereas classical machine learning classifiers, despite their simplicity, achieve performance similar to CNNs. Our findings highlight the strength of classical methods in bridging model simplicity with output quality, offering new insights into ASCII art synthesis and machine learning on image data with low dimensionality.
* 9 pages, 7 figures, 3 tables. Code available at
https://github.com/saiccoumar/deep_ascii_converter
Via
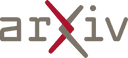
Mar 13, 2025
Abstract:We propose a diffusion-based inverse rendering framework that decomposes a single RGB image into geometry, material, and lighting. Inverse rendering is inherently ill-posed, making it difficult to predict a single accurate solution. To address this challenge, recent generative model-based methods aim to present a range of possible solutions. However, finding a single accurate solution and generating diverse solutions can be conflicting. In this paper, we propose a channel-wise noise scheduling approach that allows a single diffusion model architecture to achieve two conflicting objectives. The resulting two diffusion models, trained with different channel-wise noise schedules, can predict a single highly accurate solution and present multiple possible solutions. The experimental results demonstrate the superiority of our two models in terms of both diversity and accuracy, which translates to enhanced performance in downstream applications such as object insertion and material editing.
* Accepted by CVPR 2025
Via
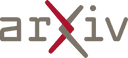