May 05, 2025
Abstract:In this paper,we explore the application of Back translation (BT) as a semi-supervised technique to enhance Neural Machine Translation(NMT) models for the English-Luganda language pair, specifically addressing the challenges faced by low-resource languages. The purpose of our study is to demonstrate how BT can mitigate the scarcity of bilingual data by generating synthetic data from monolingual corpora. Our methodology involves developing custom NMT models using both publicly available and web-crawled data, and applying Iterative and Incremental Back translation techniques. We strategically select datasets for incremental back translation across multiple small datasets, which is a novel element of our approach. The results of our study show significant improvements, with translation performance for the English-Luganda pair exceeding previous benchmarks by more than 10 BLEU score units across all translation directions. Additionally, our evaluation incorporates comprehensive assessment metrics such as SacreBLEU, ChrF2, and TER, providing a nuanced understanding of translation quality. The conclusion drawn from our research confirms the efficacy of BT when strategically curated datasets are utilized, establishing new performance benchmarks and demonstrating the potential of BT in enhancing NMT models for low-resource languages.
* ACM Digital Library, 2025, Pages 142-148
* NLPIR '24: Proceedings of the 2024 8th International Conference on
Natural Language Processing and Information Retrieval
Via
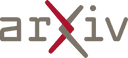