What is Generative AI? Generative AI or generative artificial intelligence refers to a type of AI that can create various types of content including text, audio, music, images, videos, and code. This is powered by large models called foundation models that are trained on massive datasets to perform out-of-the-box tasks including classification, summarization, video and audio comprehension, prediction, Q&A, and more.
Papers and Code
Mar 27, 2025
Abstract:Discrete-event simulation (DES) is widely used in healthcare Operations Research, but the models themselves are rarely shared. This limits their potential for reuse and long-term impact in the modelling and healthcare communities. This study explores the feasibility of using generative artificial intelligence (AI) to recreate published models using Free and Open Source Software (FOSS), based on the descriptions provided in an academic journal. Using a structured methodology, we successfully generated, tested and internally reproduced two DES models, including user interfaces. The reported results were replicated for one model, but not the other, likely due to missing information on distributions. These models are substantially more complex than AI-generated DES models published to date. Given the challenges we faced in prompt engineering, code generation, and model testing, we conclude that our iterative approach to model development, systematic comparison and testing, and the expertise of our team were necessary to the success of our recreated simulation models.
Via
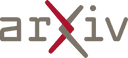
Mar 27, 2025
Abstract:Audio watermarking is increasingly used to verify the provenance of AI-generated content, enabling applications such as detecting AI-generated speech, protecting music IP, and defending against voice cloning. To be effective, audio watermarks must resist removal attacks that distort signals to evade detection. While many schemes claim robustness, these claims are typically tested in isolation and against a limited set of attacks. A systematic evaluation against diverse removal attacks is lacking, hindering practical deployment. In this paper, we investigate whether recent watermarking schemes that claim robustness can withstand a broad range of removal attacks. First, we introduce a taxonomy covering 22 audio watermarking schemes. Next, we summarize their underlying technologies and potential vulnerabilities. We then present a large-scale empirical study to assess their robustness. To support this, we build an evaluation framework encompassing 22 types of removal attacks (109 configurations) including signal-level, physical-level, and AI-induced distortions. We reproduce 9 watermarking schemes using open-source code, identify 8 new highly effective attacks, and highlight 11 key findings that expose the fundamental limitations of these methods across 3 public datasets. Our results reveal that none of the surveyed schemes can withstand all tested distortions. This evaluation offers a comprehensive view of how current watermarking methods perform under real-world threats. Our demo and code are available at https://sokaudiowm.github.io/.
Via
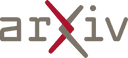
Mar 27, 2025
Abstract:Accurate and interpretable detection of AI-generated images is essential for mitigating risks associated with AI misuse. However, the substantial domain gap among generative models makes it challenging to develop a generalizable forgery detection model. Moreover, since every pixel in an AI-generated image is synthesized, traditional saliency-based forgery explanation methods are not well suited for this task. To address these challenges, we propose modeling AI-generated image detection and explanation as a Forgery Detection and Reasoning task (FDR-Task), leveraging vision-language models (VLMs) to provide accurate detection through structured and reliable reasoning over forgery attributes. To facilitate this task, we introduce the Multi-Modal Forgery Reasoning dataset (MMFR-Dataset), a large-scale dataset containing 100K images across 10 generative models, with 10 types of forgery reasoning annotations, enabling comprehensive evaluation of FDR-Task. Additionally, we propose FakeReasoning, a forgery detection and reasoning framework with two key components. First, Forgery-Aligned Contrastive Learning enhances VLMs' understanding of forgery-related semantics through both cross-modal and intra-modal contrastive learning between images and forgery attribute reasoning. Second, a Classification Probability Mapper bridges the optimization gap between forgery detection and language modeling by mapping the output logits of VLMs to calibrated binary classification probabilities. Experiments across multiple generative models demonstrate that FakeReasoning not only achieves robust generalization but also outperforms state-of-the-art methods on both detection and reasoning tasks.
Via
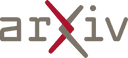
Mar 27, 2025
Abstract:The surge in generative AI workloads has created a need for scalable inference systems that can flexibly harness both GPUs and specialized accelerators while containing operational costs. This paper proposes a hardware-agnostic control loop that adaptively allocates requests across heterogeneous accelerators based on real-time cost and capacity signals. The approach sustains low latency and high throughput by dynamically shifting between cost-optimized and capacity-optimized modes, ensuring the most efficient use of expensive compute resources under fluctuating availability. Evaluated using the Stable Diffusion model, the framework consistently meets latency targets, automatically redirects traffic during capacity shortfalls, and capitalizes on lower-cost accelerators when possible. These results highlight how a feedback-driven deployment strategy, spanning the entire software and hardware stack, can help organizations efficiently scale generative AI workloads while maintaining resilience in the face of limited accelerator capacity.
* 14 pages, 7 figures
Via
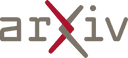
Mar 27, 2025
Abstract:We propose a method to explore the flavor structure of leptons using diffusion models, which are known as one of generative artificial intelligence (generative AI). We consider a simple extension of the Standard Model with the type I seesaw mechanism and train a neural network to generate the neutrino mass matrix. By utilizing transfer learning, the diffusion model generates 104 solutions that are consistent with the neutrino mass squared differences and the leptonic mixing angles. The distributions of the CP phases and the sums of neutrino masses, which are not included in the conditional labels but are calculated from the solutions, exhibit non-trivial tendencies. In addition, the effective mass in neutrinoless double beta decay is concentrated near the boundaries of the existing confidence intervals, allowing us to verify the obtained solutions through future experiments. An inverse approach using the diffusion model is expected to facilitate the experimental verification of flavor models from a perspective distinct from conventional analytical methods.
* 23 pages, 5 figures
Via
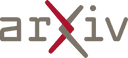
Mar 27, 2025
Abstract:Generative AI models offer many possibilities for text creation and transformation. Current graphical user interfaces (GUIs) for prompting them lack support for iterative exploration, as they do not represent prompts as actionable interface objects. We propose the concept of a composable prompting canvas for text exploration and iteration using dynamic widgets. Users generate widgets through system suggestions, prompting, or manually to capture task-relevant facets that affect the generated text. In a comparative study with a baseline (conversational UI), 18 participants worked on two writing tasks, creating diverse prompting environments with custom widgets and spatial layouts. They reported having more control over the generated text and preferred our system over the baseline. Our design significantly outperformed the baseline on the Creativity Support Index, and participants felt the results were worth the effort. This work highlights the need for GUIs that support user-driven customization and (re-)structuring to increase both the flexibility and efficiency of prompting.
* 11 pages, 9 figures, 2 tables, ACM CHI 2025 LBW
Via
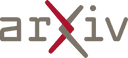
Mar 27, 2025
Abstract:We study the ability of large language models (LLMs) to generate comprehensive and accurate book summaries solely from their internal knowledge, without recourse to the original text. Employing a diverse set of books and multiple LLM architectures, we examine whether these models can synthesize meaningful narratives that align with established human interpretations. Evaluation is performed with a LLM-as-a-judge paradigm: each AI-generated summary is compared against a high-quality, human-written summary via a cross-model assessment, where all participating LLMs evaluate not only their own outputs but also those produced by others. This methodology enables the identification of potential biases, such as the proclivity for models to favor their own summarization style over others. In addition, alignment between the human-crafted and LLM-generated summaries is quantified using ROUGE and BERTScore metrics, assessing the depth of grammatical and semantic correspondence. The results reveal nuanced variations in content representation and stylistic preferences among the models, highlighting both strengths and limitations inherent in relying on internal knowledge for summarization tasks. These findings contribute to a deeper understanding of LLM internal encodings of factual information and the dynamics of cross-model evaluation, with implications for the development of more robust natural language generative systems.
* 22 pages, 6 figures
Via
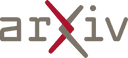
Mar 26, 2025
Abstract:The demand for high-quality synthetic data for model training and augmentation has never been greater in medical imaging. However, current evaluations predominantly rely on computational metrics that fail to align with human expert recognition. This leads to synthetic images that may appear realistic numerically but lack clinical authenticity, posing significant challenges in ensuring the reliability and effectiveness of AI-driven medical tools. To address this gap, we introduce GazeVal, a practical framework that synergizes expert eye-tracking data with direct radiological evaluations to assess the quality of synthetic medical images. GazeVal leverages gaze patterns of radiologists as they provide a deeper understanding of how experts perceive and interact with synthetic data in different tasks (i.e., diagnostic or Turing tests). Experiments with sixteen radiologists revealed that 96.6% of the generated images (by the most recent state-of-the-art AI algorithm) were identified as fake, demonstrating the limitations of generative AI in producing clinically accurate images.
Via
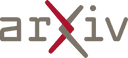
Mar 26, 2025
Abstract:The emergence of advanced AI-based tools to generate realistic images poses significant challenges for forensic detection and source attribution, especially as new generative techniques appear rapidly. Traditional methods often fail to generalize to unseen generators due to reliance on features specific to known sources during training. To address this problem, we propose a novel approach that explicitly models forensic microstructures - subtle, pixel-level patterns unique to the image creation process. Using only real images in a self-supervised manner, we learn a set of diverse predictive filters to extract residuals that capture different aspects of these microstructures. By jointly modeling these residuals across multiple scales, we obtain a compact model whose parameters constitute a unique forensic self-description for each image. This self-description enables us to perform zero-shot detection of synthetic images, open-set source attribution of images, and clustering based on source without prior knowledge. Extensive experiments demonstrate that our method achieves superior accuracy and adaptability compared to competing techniques, advancing the state of the art in synthetic media forensics.
* Proceedings of the IEEE/CVF Conference on Computer Vision and Pattern
Recognition (CVPR) 2025
Via
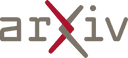
Mar 26, 2025
Abstract:With video games steadily increasing in complexity, automated generation of game content has found widespread interest. However, the task of 3D gaming map art creation remains underexplored to date due to its unique complexity and domain-specific challenges. While recent works have addressed related topics such as retro-style level generation and procedural terrain creation, these works primarily focus on simpler data distributions. To the best of our knowledge, we are the first to demonstrate the application of modern AI techniques for high-resolution texture manipulation in complex, highly detailed AAA 3D game environments. We introduce a novel Smart Brush for map editing, designed to assist artists in seamlessly modifying selected areas of a game map with minimal effort. By leveraging generative adversarial networks and diffusion models we propose two variants of the brush that enable efficient and context-aware generation. Our hybrid workflow aims to enhance both artistic flexibility and production efficiency, enabling the refinement of environments without manually reworking every detail, thus helping to bridge the gap between automation and creative control in game development. A comparative evaluation of our two methods with adapted versions of several state-of-the art models shows that our GAN-based brush produces the sharpest and most detailed outputs while preserving image context while the evaluated state-of-the-art models tend towards blurrier results and exhibit difficulties in maintaining contextual consistency.
Via
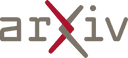