What is speech recognition? Speech recognition is the task of identifying words spoken aloud, analyzing the voice and language, and accurately transcribing the words.
Papers and Code
May 28, 2025
Abstract:Traditional audiometry often fails to fully characterize the functional impact of hearing loss on speech understanding, particularly supra-threshold deficits and frequency-specific perception challenges in conditions like presbycusis. This paper presents the development and simulated evaluation of a novel Automatic Speech Recognition (ASR)-based frequency-specific speech test designed to provide granular diagnostic insights. Our approach leverages ASR to simulate the perceptual effects of moderate sloping hearing loss by processing speech stimuli under controlled acoustic degradation and subsequently analyzing phoneme-level confusion patterns. Key findings indicate that simulated hearing loss introduces specific phoneme confusions, predominantly affecting high-frequency consonants (e.g., alveolar/palatal to labiodental substitutions) and leading to significant phoneme deletions, consistent with the acoustic cues degraded in presbycusis. A test battery curated from these ASR-derived confusions demonstrated diagnostic value, effectively differentiating between simulated normal-hearing and hearing-impaired listeners in a comprehensive simulation. This ASR-driven methodology offers a promising avenue for developing objective, granular, and frequency-specific hearing assessment tools that complement traditional audiometry. Future work will focus on validating these findings with human participants and exploring the integration of advanced AI models for enhanced diagnostic precision.
Via
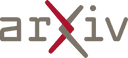
May 27, 2025
Abstract:Whisper's robust performance in automatic speech recognition (ASR) is often attributed to its massive 680k-hour training set, an impractical scale for most researchers. In this work, we examine how linguistic and acoustic diversity in training data affect the robustness of the ASR model and reveal that transcription generalization is primarily driven by acoustic variation rather than linguistic richness. We find that targeted acoustic augmentation methods could significantly improve the generalization ability of ASR models, reducing word-error rates by up to 19.24 percent on unseen datasets when training on the 960-hour Librispeech dataset. These findings highlight strategic acoustically focused data augmentation as a promising alternative to massive datasets for building robust ASR models, offering a potential solution to future foundation ASR models when massive human speech data is lacking.
* in submission
Via
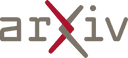
May 29, 2025
Abstract:Traditional anti-spoofing focuses on models and datasets built on synthetic speech with mostly neutral state, neglecting diverse emotional variations. As a result, their robustness against high-quality, emotionally expressive synthetic speech is uncertain. We address this by introducing EmoSpoof-TTS, a corpus of emotional text-to-speech samples. Our analysis shows existing anti-spoofing models struggle with emotional synthetic speech, exposing risks of emotion-targeted attacks. Even trained on emotional data, the models underperform due to limited focus on emotional aspect and show performance disparities across emotions. This highlights the need for emotion-focused anti-spoofing paradigm in both dataset and methodology. We propose GEM, a gated ensemble of emotion-specialized models with a speech emotion recognition gating network. GEM performs effectively across all emotions and neutral state, improving defenses against spoofing attacks. We release the EmoSpoof-TTS Dataset: https://emospoof-tts.github.io/Dataset/
* Accepted to Interspeech 2025
Via
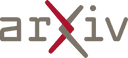
May 27, 2025
Abstract:This paper describes the GMU systems for the IWSLT 2025 low-resource speech translation shared task. We trained systems for all language pairs, except for Levantine Arabic. We fine-tuned SeamlessM4T-v2 for automatic speech recognition (ASR), machine translation (MT), and end-to-end speech translation (E2E ST). The ASR and MT models are also used to form cascaded ST systems. Additionally, we explored various training paradigms for E2E ST fine-tuning, including direct E2E fine-tuning, multi-task training, and parameter initialization using components from fine-tuned ASR and/or MT models. Our results show that (1) direct E2E fine-tuning yields strong results; (2) initializing with a fine-tuned ASR encoder improves ST performance on languages SeamlessM4T-v2 has not been trained on; (3) multi-task training can be slightly helpful.
* IWSLT 2025
Via
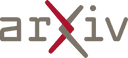
Jun 04, 2025
Abstract:Sign Language Recognition (SLR) involves the automatic identification and classification of sign gestures from images or video, converting them into text or speech to improve accessibility for the hearing-impaired community. In Bangladesh, Bangla Sign Language (BdSL) serves as the primary mode of communication for many individuals with hearing impairments. This study fine-tunes state-of-the-art video transformer architectures -- VideoMAE, ViViT, and TimeSformer -- on BdSLW60 (arXiv:2402.08635), a small-scale BdSL dataset with 60 frequent signs. We standardized the videos to 30 FPS, resulting in 9,307 user trial clips. To evaluate scalability and robustness, the models were also fine-tuned on BdSLW401 (arXiv:2503.02360), a large-scale dataset with 401 sign classes. Additionally, we benchmark performance against public datasets, including LSA64 and WLASL. Data augmentation techniques such as random cropping, horizontal flipping, and short-side scaling were applied to improve model robustness. To ensure balanced evaluation across folds during model selection, we employed 10-fold stratified cross-validation on the training set, while signer-independent evaluation was carried out using held-out test data from unseen users U4 and U8. Results show that video transformer models significantly outperform traditional machine learning and deep learning approaches. Performance is influenced by factors such as dataset size, video quality, frame distribution, frame rate, and model architecture. Among the models, the VideoMAE variant (MCG-NJU/videomae-base-finetuned-kinetics) achieved the highest accuracies of 95.5% on the frame rate corrected BdSLW60 dataset and 81.04% on the front-facing signs of BdSLW401 -- demonstrating strong potential for scalable and accurate BdSL recognition.
* 16 pages, 8 figures, 6 tables
Via
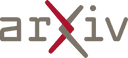
May 29, 2025
Abstract:We present ZIPA, a family of efficient speech models that advances the state-of-the-art performance of crosslinguistic phone recognition. We first curated IPAPack++, a large-scale multilingual speech corpus with 17,132 hours of normalized phone transcriptions and a novel evaluation set capturing unseen languages and sociophonetic variation. With the large-scale training data, ZIPA, including transducer (ZIPA-T) and CTC-based (ZIPA-CR) variants, leverage the efficient Zipformer backbones and outperform existing phone recognition systems with much fewer parameters. Further scaling via noisy student training on 11,000 hours of pseudo-labeled multilingual data yields further improvement. While ZIPA achieves strong performance on benchmarks, error analysis reveals persistent limitations in modeling sociophonetic diversity, underscoring challenges for future research.
* ACL 2025 Main
Via
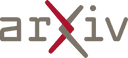
May 27, 2025
Abstract:Model compression has become an emerging need as the sizes of modern speech systems rapidly increase. In this paper, we study model weight quantization, which directly reduces the memory footprint to accommodate computationally resource-constrained applications. We propose novel approaches to perform extremely low-bit (i.e., 2-bit and 1-bit) quantization of Conformer automatic speech recognition systems using multiple precision model co-training, stochastic precision, and tensor-wise learnable scaling factors to alleviate quantization incurred performance loss. The proposed methods can achieve performance-lossless 2-bit and 1-bit quantization of Conformer ASR systems trained with the 300-hr Switchboard and 960-hr LibriSpeech corpus. Maximum overall performance-lossless compression ratios of 16.2 and 16.6 times are achieved without a statistically significant increase in the word error rate (WER) over the full precision baseline systems, respectively.
* Accepted by Interspeech2025
Via
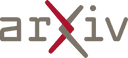
May 26, 2025
Abstract:In this work, we present the first study addressing automatic speech recognition (ASR) for children in an online learning setting. This is particularly important for both child-centric applications and the privacy protection of minors, where training models with sequentially arriving data is critical. The conventional approach of model fine-tuning often suffers from catastrophic forgetting. To tackle this issue, we explore two established techniques: elastic weight consolidation (EWC) and synaptic intelligence (SI). Using a custom protocol on the MyST corpus, tailored to the online learning setting, we achieve relative word error rate (WER) reductions of 5.21% with EWC and 4.36% with SI, compared to the fine-tuning baseline.
* Accepted at INTERSPEECH 2025. 5 pages
Via
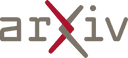
May 28, 2025
Abstract:Speech emotion recognition (SER), particularly for naturally expressed emotions, remains a challenging computational task. Key challenges include the inherent subjectivity in emotion annotation and the imbalanced distribution of emotion labels in datasets. This paper introduces the \texttt{SAILER} system developed for participation in the INTERSPEECH 2025 Emotion Recognition Challenge (Task 1). The challenge dataset, which contains natural emotional speech from podcasts, serves as a valuable resource for studying imbalanced and subjective emotion annotations. Our system is designed to be simple, reproducible, and effective, highlighting critical choices in modeling, learning objectives, data augmentation, and engineering choices. Results show that even a single system (without ensembling) can outperform more than 95\% of the submissions, with a Macro-F1 score exceeding 0.4. Moreover, an ensemble of three systems further improves performance, achieving a competitively ranked score (top-3 performing team). Our model is at: https://github.com/tiantiaf0627/vox-profile-release.
* Accepted to INTERSPEECH 2025
Via
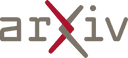
May 23, 2025
Abstract:This work presents a suite of fine-tuned Whisper models for Swedish, trained on a dataset of unprecedented size and variability for this mid-resourced language. As languages of smaller sizes are often underrepresented in multilingual training datasets, substantial improvements in performance can be achieved by fine-tuning existing multilingual models, as shown in this work. This work reports an overall improvement across model sizes compared to OpenAI's Whisper evaluated on Swedish. Most notably, we report an average 47% reduction in WER comparing our best performing model to OpenAI's whisper-large-v3, in evaluations across FLEURS, Common Voice, and NST.
* Submitted to Interspeech 2025
Via
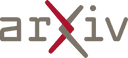