What is music generation? Music generation is the task of generating music or music-like sounds from a model or algorithm.
Papers and Code
Jan 03, 2025
Abstract:Recent years have witnessed the success of foundation models pre-trained with self-supervised learning (SSL) in various music informatics understanding tasks, including music tagging, instrument classification, key detection, and more. In this paper, we propose a self-supervised music representation learning model for music understanding. Distinguished from previous studies adopting random projection or existing neural codec, the proposed model, named MuQ, is trained to predict tokens generated by Mel Residual Vector Quantization (Mel-RVQ). Our Mel-RVQ utilizes residual linear projection structure for Mel spectrum quantization to enhance the stability and efficiency of target extraction and lead to better performance. Experiments in a large variety of downstream tasks demonstrate that MuQ outperforms previous self-supervised music representation models with only 0.9K hours of open-source pre-training data. Scaling up the data to over 160K hours and adopting iterative training consistently improve the model performance. To further validate the strength of our model, we present MuQ-MuLan, a joint music-text embedding model based on contrastive learning, which achieves state-of-the-art performance in the zero-shot music tagging task on the MagnaTagATune dataset. Code and checkpoints are open source in https://github.com/tencent-ailab/MuQ.
Via
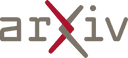
Dec 12, 2024
Abstract:Human motion generation involves creating natural sequences of human body poses, widely used in gaming, virtual reality, and human-computer interaction. It aims to produce lifelike virtual characters with realistic movements, enhancing virtual agents and immersive experiences. While previous work has focused on motion generation based on signals like movement, music, text, or scene background, the complexity of human motion and its relationships with these signals often results in unsatisfactory outputs. Manifold learning offers a solution by reducing data dimensionality and capturing subspaces of effective motion. In this review, we present a comprehensive overview of manifold applications in human motion generation, one of the first in this domain. We explore methods for extracting manifolds from unstructured data, their application in motion generation, and discuss their advantages and future directions. This survey aims to provide a broad perspective on the field and stimulate new approaches to ongoing challenges.
Via
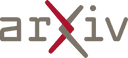
Dec 13, 2024
Abstract:Tipping points are moments of change that characterise crucial turning points in a piece of music. This study presents a first step towards quantitatively and systematically describing the musical properties of tipping points. Timing information and computationally-derived tonal tension values which correspond to dissonance, distance from key, and harmonic motion are compared to tipping points in Ashkenazy's recordings of six Chopin Mazurkas, as identified by 35 listeners. The analysis shows that all popular tipping points but one could be explained by statistically significant timing deviations or changepoints in at least one of the three tension parameters.
* International Society for Music Information Retrieval Conference, Oct
2017, Suzhou, China, China
Via
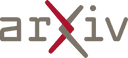
Jan 10, 2025
Abstract:The restoration of nonlinearly distorted audio signals, alongside the identification of the applied memoryless nonlinear operation, is studied. The paper focuses on the difficult but practically important case in which both the nonlinearity and the original input signal are unknown. The proposed method uses a generative diffusion model trained unconditionally on guitar or speech signals to jointly model and invert the nonlinear system at inference time. Both the memoryless nonlinear function model and the restored audio signal are obtained as output. Successful example case studies are presented including inversion of hard and soft clipping, digital quantization, half-wave rectification, and wavefolding nonlinearities. Our results suggest that, out of the nonlinear functions tested here, the cubic Catmull-Rom spline is best suited to approximating these nonlinearities. In the case of guitar recordings, comparisons with informed and supervised methods show that the proposed blind method is at least as good as they are in terms of objective metrics. Experiments on distorted speech show that the proposed blind method outperforms general-purpose speech enhancement techniques and restores the original voice quality. The proposed method can be applied to audio effects modeling, restoration of music and speech recordings, and characterization of analog recording media.
* Submitted to the Journal of Audio Engineering Society, special issue
"The Sound of Digital Audio Effects"
Via
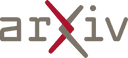
Dec 12, 2024
Abstract:Singing Voice Synthesis (SVS) {aims} to generate singing voices {of high} fidelity and expressiveness. {Conventional SVS systems usually utilize} an acoustic model to transform a music score into acoustic features, {followed by a vocoder to reconstruct the} singing voice. It was recently shown that end-to-end modeling is effective in the fields of SVS and Text to Speech (TTS). In this work, we thus present a fully end-to-end SVS method together with a chunkwise streaming inference to address the latency issue for practical usages. Note that this is the first attempt to fully implement end-to-end streaming audio synthesis using latent representations in VAE. We have made specific improvements to enhance the performance of streaming SVS using latent representations. Experimental results demonstrate that the proposed method achieves synthesized audio with high expressiveness and pitch accuracy in both streaming SVS and TTS tasks.
* Accepted by AAAI2025
Via
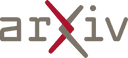
Dec 23, 2024
Abstract:As artificial intelligence (AI) becomes increasingly central to various fields, there is a growing need to equip K-12 students with AI literacy skills that extend beyond computer science. This paper explores the integration of a Project-Based Learning (PBL) AI toolkit into diverse subject areas, aimed at helping educators teach AI concepts more effectively. Through interviews and co-design sessions with K-12 teachers, we examined current AI literacy levels and how teachers adapt AI tools like the AI Art Lab, AI Music Studio, and AI Chatbot into their course designs. While teachers appreciated the potential of AI tools to foster creativity and critical thinking, they also expressed concerns about the accuracy, trustworthiness, and ethical implications of AI-generated content. Our findings reveal the challenges teachers face, including limited resources, varying student and instructor skill levels, and the need for scalable, adaptable AI tools. This research contributes insights that can inform the development of AI curricula tailored to diverse educational contexts.
* Accepted to AAAI2025
Via
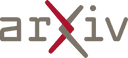
Dec 23, 2024
Abstract:Recommender systems create enormous value for businesses and their consumers. They increase revenue for businesses while improving the consumer experience by recommending relevant products amidst huge product base. Product bundling is an exciting development in the field of product recommendations. It aims at generating new bundles and recommending exciting and relevant bundles to their consumers. Unlike traditional recommender systems that recommend single items to consumers, product bundling aims at targeting a bundle, or a set of items, to the consumers. While bundle recommendation has attracted significant research interest recently, extant literature on bundle generation is scarce. Moreover, metrics to identify if a bundle is popular or not is not well studied. In this work, we aim to fulfill this gap by introducing new bundle popularity metrics based on sales, consumer experience and item diversity in a bundle. We use these metrics in the methodology proposed in this paper to generate new bundles for mobile games using content aware and context aware embeddings. We use opensource Steam Games dataset for our analysis. Our experiments indicate that we can generate new bundles that can outperform the existing bundles on the popularity metrics by 32% - 44%. Our experiments are computationally efficient and the proposed methodology is generic that can be extended to other bundling problems e.g. product bundling, music bundling.
Via
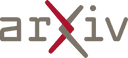
Dec 16, 2024
Abstract:Detecting music entities such as song titles or artist names is a useful application to help use cases like processing music search queries or analyzing music consumption on the web. Recent approaches incorporate smaller language models (SLMs) like BERT and achieve high results. However, further research indicates a high influence of entity exposure during pre-training on the performance of the models. With the advent of large language models (LLMs), these outperform SLMs in a variety of downstream tasks. However, researchers are still divided if this is applicable to tasks like entity detection in texts due to issues like hallucination. In this paper, we provide a novel dataset of user-generated metadata and conduct a benchmark and a robustness study using recent LLMs with in-context-learning (ICL). Our results indicate that LLMs in the ICL setting yield higher performance than SLMs. We further uncover the large impact of entity exposure on the best performing LLM in our study.
Via
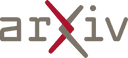
Dec 11, 2024
Abstract:Catalyzed by advancements in hardware and software, drone performances are increasingly making their mark in the entertainment industry. However, designing smooth and safe choreographies for drone swarms is complex and often requires expert domain knowledge. In this work, we introduce SwarmGPT-Primitive, a language-based choreographer that integrates the reasoning capabilities of large language models (LLMs) with safe motion planning to facilitate deployable drone swarm choreographies. The LLM composes choreographies for a given piece of music by utilizing a library of motion primitives; the language-based choreographer is augmented with an optimization-based safety filter, which certifies the choreography for real-world deployment by making minimal adjustments when feasibility and safety constraints are violated. The overall SwarmGPT-Primitive framework decouples choreographic design from safe motion planning, which allows non-expert users to re-prompt and refine compositions without concerns about compliance with constraints such as avoiding collisions or downwash effects or satisfying actuation limits. We demonstrate our approach through simulations and experiments with swarms of up to 20 drones performing choreographies designed based on various songs, highlighting the system's ability to generate effective and synchronized drone choreographies for real-world deployment.
* Submitted to ICRA 2025
Via
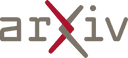
Dec 12, 2024
Abstract:Modern sensors produce increasingly rich streams of high-resolution data. Due to resource constraints, machine learning systems discard the vast majority of this information via resolution reduction. Compressed-domain learning allows models to operate on compact latent representations, allowing higher effective resolution for the same budget. However, existing compression systems are not ideal for compressed learning. Linear transform coding and end-to-end learned compression systems reduce bitrate, but do not uniformly reduce dimensionality; thus, they do not meaningfully increase efficiency. Generative autoencoders reduce dimensionality, but their adversarial or perceptual objectives lead to significant information loss. To address these limitations, we introduce WaLLoC (Wavelet Learned Lossy Compression), a neural codec architecture that combines linear transform coding with nonlinear dimensionality-reducing autoencoders. WaLLoC sandwiches a shallow, asymmetric autoencoder and entropy bottleneck between an invertible wavelet packet transform. Across several key metrics, WaLLoC outperforms the autoencoders used in state-of-the-art latent diffusion models. WaLLoC does not require perceptual or adversarial losses to represent high-frequency detail, providing compatibility with modalities beyond RGB images and stereo audio. WaLLoC's encoder consists almost entirely of linear operations, making it exceptionally efficient and suitable for mobile computing, remote sensing, and learning directly from compressed data. We demonstrate WaLLoC's capability for compressed-domain learning across several tasks, including image classification, colorization, document understanding, and music source separation. Our code, experiments, and pre-trained audio and image codecs are available at https://ut-sysml.org/walloc
* Accepted as paper to 2025 IEEE Data Compression Conference
Via
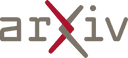