What is cancer detection? Cancer detection using Artificial Intelligence (AI) involves leveraging advanced machine learning algorithms and techniques to identify and diagnose cancer from various medical data sources. The goal is to enhance early detection, improve diagnostic accuracy, and potentially reduce the need for invasive procedures.
Papers and Code
Jan 13, 2025
Abstract:In this paper we discuss lung cancer detection using hybrid model of Convolutional-Neural-Networks (CNNs) and Support-Vector-Machines-(SVMs) in order to gain early detection of tumors, benign or malignant. The work uses this hybrid model by training upon the Computed Tomography scans (CT scans) as dataset. Using deep learning for detecting lung cancer early is a cutting-edge method.
Via
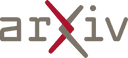
Feb 11, 2025
Abstract:Prostate cancer is a leading health concern among men, requiring accurate and accessible methods for early detection and risk stratification. Prostate volume (PV) is a key parameter in multivariate risk stratification for early prostate cancer detection, commonly estimated using transrectal ultrasound (TRUS). While TRUS provides precise prostate volume measurements, its invasive nature often compromises patient comfort. Transabdominal ultrasound (TAUS) provides a non-invasive alternative but faces challenges such as lower image quality, complex interpretation, and reliance on operator expertise. This study introduces a new deep-learning-based framework for automatic PV estimation using TAUS, emphasizing its potential to enable accurate and non-invasive prostate cancer risk stratification. A dataset of TAUS videos from 100 individual patients was curated, with manually delineated prostate boundaries and calculated diameters by an expert clinician as ground truth. The introduced framework integrates deep-learning models for prostate segmentation in both axial and sagittal planes, automatic prostate diameter estimation, and PV calculation. Segmentation performance was evaluated using Dice correlation coefficient (%) and Hausdorff distance (mm). Framework's volume estimation capabilities were evaluated on volumetric error (mL). The framework demonstrates that it can estimate PV from TAUS videos with a mean volumetric error of -5.5 mL, which results in an average relative error between 5 and 15%. The introduced framework for automatic PV estimation from TAUS images, utilizing deep learning models for prostate segmentation, shows promising results. It effectively segments the prostate and estimates its volume, offering potential for reliable, non-invasive risk stratification for early prostate detection.
Via
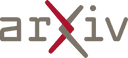
Feb 25, 2025
Abstract:Colorectal cancer (CRC) is a significant global health concern, and early detection through screening plays a critical role in reducing mortality. While deep learning models have shown promise in improving polyp detection, classification, and segmentation, their generalization across diverse clinical environments, particularly with out-of-distribution (OOD) data, remains a challenge. Multi-center datasets like PolypGen have been developed to address these issues, but their collection is costly and time-consuming. Traditional data augmentation techniques provide limited variability, failing to capture the complexity of medical images. Diffusion models have emerged as a promising solution for generating synthetic polyp images, but the image generation process in current models mainly relies on segmentation masks as the condition, limiting their ability to capture the full clinical context. To overcome these limitations, we propose a Progressive Spectrum Diffusion Model (PSDM) that integrates diverse clinical annotations-such as segmentation masks, bounding boxes, and colonoscopy reports-by transforming them into compositional prompts. These prompts are organized into coarse and fine components, allowing the model to capture both broad spatial structures and fine details, generating clinically accurate synthetic images. By augmenting training data with PSDM-generated samples, our model significantly improves polyp detection, classification, and segmentation. For instance, on the PolypGen dataset, PSDM increases the F1 score by 2.12% and the mean average precision by 3.09%, demonstrating superior performance in OOD scenarios and enhanced generalization.
Via
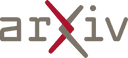
Feb 08, 2025
Abstract:Neural networks have become the standard technique for medical diagnostics, especially in cancer detection and classification. This work evaluates the performance of Vision Transformers architectures, including Swin Transformer and MaxViT, in several datasets of magnetic resonance imaging (MRI) and computed tomography (CT) scans. We used three training sets of images with brain, lung, and kidney tumors. Each dataset includes different classification labels, from brain gliomas and meningiomas to benign and malignant lung conditions and kidney anomalies such as cysts and cancers. This work aims to analyze the behavior of the neural networks in each dataset and the benefits of combining different image modalities and tumor classes. We designed several experiments by fine-tuning the models on combined and individual image modalities. The results revealed that the Swin Transformer provided high accuracy, achieving up to 99.9\% for kidney tumor classification and 99.3\% accuracy in a combined dataset. MaxViT also provided excellent results in individual datasets but performed poorly when data is combined. This research highlights the adaptability of Transformer-based models to various image modalities and features. However, challenges persist, including limited annotated data and interpretability issues. Future works will expand this study by incorporating other image modalities and enhancing diagnostic capabilities. Integrating these models across diverse datasets could mark a pivotal advance in precision medicine, paving the way for more efficient and comprehensive healthcare solutions.
* 13 pages, 3 figures, 8 tables
Via
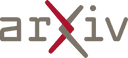
Jan 26, 2025
Abstract:Artificial intelligence (AI) has potential to revolutionize the field of oncology by enhancing the precision of cancer diagnosis, optimizing treatment strategies, and personalizing therapies for a variety of cancers. This review examines the limitations of conventional diagnostic techniques and explores the transformative role of AI in diagnosing and treating cancers such as lung, breast, colorectal, liver, stomach, esophageal, cervical, thyroid, prostate, and skin cancers. The primary objective of this paper is to highlight the significant advancements that AI algorithms have brought to oncology within the medical industry. By enabling early cancer detection, improving diagnostic accuracy, and facilitating targeted treatment delivery, AI contributes to substantial improvements in patient outcomes. The integration of AI in medical imaging, genomic analysis, and pathology enhances diagnostic precision and introduces a novel, less invasive approach to cancer screening. This not only boosts the effectiveness of medical facilities but also reduces operational costs. The study delves into the application of AI in radiomics for detailed cancer characterization, predictive analytics for identifying associated risks, and the development of algorithm-driven robots for immediate diagnosis. Furthermore, it investigates the impact of AI on addressing healthcare challenges, particularly in underserved and remote regions. The overarching goal of this platform is to support the development of expert recommendations and to provide universal, efficient diagnostic procedures. By reviewing existing research and clinical studies, this paper underscores the pivotal role of AI in improving the overall cancer care system. It emphasizes how AI-enabled systems can enhance clinical decision-making and expand treatment options, thereby underscoring the importance of AI in advancing precision oncology
Via
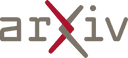
Jan 30, 2025
Abstract:With over 2 million new cases identified annually, skin cancer is the most prevalent type of cancer globally and the second most common in Bangladesh, following breast cancer. Early detection and treatment are crucial for enhancing patient outcomes; however, Bangladesh faces a shortage of dermatologists and qualified medical professionals capable of diagnosing and treating skin cancer. As a result, many cases are diagnosed only at advanced stages. Research indicates that deep learning algorithms can effectively classify skin cancer images. However, these models typically lack interpretability, making it challenging to understand their decision-making processes. This lack of clarity poses barriers to utilizing deep learning in improving skin cancer detection and treatment. In this article, we present a method aimed at enhancing the interpretability of deep learning models for skin cancer classification in Bangladesh. Our technique employs a combination of saliency maps and attention maps to visualize critical features influencing the model's diagnoses.
* 18 pages
Via
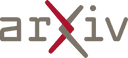
Jan 21, 2025
Abstract:Prostate cancer (PCa) detection using deep learning (DL) models has shown potential for enhancing real-time guidance during biopsies. However, prostate ultrasound images lack pixel-level cancer annotations, introducing label noise. Current approaches often focus on limited regions of interest (ROIs), disregarding anatomical context necessary for accurate diagnosis. Foundation models can overcome this limitation by analyzing entire images to capture global spatial relationships; however, they still encounter challenges stemming from the weak labels associated with coarse pathology annotations in ultrasound data. We introduce Cinepro, a novel framework that strengthens foundation models' ability to localize PCa in ultrasound cineloops. Cinepro adapts robust training by integrating the proportion of cancer tissue reported by pathology in a biopsy core into its loss function to address label noise, providing a more nuanced supervision. Additionally, it leverages temporal data across multiple frames to apply robust augmentations, enhancing the model's ability to learn stable cancer-related features. Cinepro demonstrates superior performance on a multi-center prostate ultrasound dataset, achieving an AUROC of 77.1% and a balanced accuracy of 83.8%, surpassing current benchmarks. These findings underscore Cinepro's promise in advancing foundation models for weakly labeled ultrasound data.
* accepted to IEEE ISBI 2025
Via
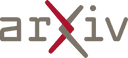
Feb 04, 2025
Abstract:This study proposes a new loss function for deep neural networks, L1-weighted Dice Focal Loss (L1DFL), that leverages L1 norms for adaptive weighting of voxels based on their classification difficulty, towards automated detection and segmentation of metastatic prostate cancer lesions in PET/CT scans. We obtained 380 PSMA [18-F] DCFPyL PET/CT scans of patients diagnosed with biochemical recurrence metastatic prostate cancer. We trained two 3D convolutional neural networks, Attention U-Net and SegResNet, and concatenated the PET and CT volumes channel-wise as input. The performance of our custom loss function was evaluated against the Dice and Dice Focal Loss functions. For clinical significance, we considered a detected region of interest (ROI) as a true positive if at least the voxel with the maximum standardized uptake value falls within the ROI. We assessed the models' performance based on the number of lesions in an image, tumour volume, activity, and extent of spread. The L1DFL outperformed the comparative loss functions by at least 13% on the test set. In addition, the F1 scores of the Dice Loss and the Dice Focal Loss were lower than that of L1DFL by at least 6% and 34%, respectively. The Dice Focal Loss yielded more false positives, whereas the Dice Loss was more sensitive to smaller volumes and struggled to segment larger lesions accurately. They also exhibited network-specific variations and yielded declines in segmentation accuracy with increased tumour spread. Our results demonstrate the potential of L1DFL to yield robust segmentation of metastatic prostate cancer lesions in PSMA PET/CT images. The results further highlight potential complexities arising from the variations in lesion characteristics that may influence automated prostate cancer tumour detection and segmentation. The code is publicly available at: https://github.com/ObedDzik/pca_segment.git.
* 29 pages, 7 figures, 1 table
Via
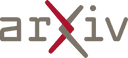
Mar 17, 2025
Abstract:Recently, histopathology vision-language foundation models (VLMs) have gained popularity due to their enhanced performance and generalizability across different downstream tasks. However, most existing histopathology benchmarks are either unimodal or limited in terms of diversity of clinical tasks, organs, and acquisition instruments, as well as their partial availability to the public due to patient data privacy. As a consequence, there is a lack of comprehensive evaluation of existing histopathology VLMs on a unified benchmark setting that better reflects a wide range of clinical scenarios. To address this gap, we introduce HistoVL, a fully open-source comprehensive benchmark comprising images acquired using up to 11 various acquisition tools that are paired with specifically crafted captions by incorporating class names and diverse pathology descriptions. Our Histo-VL includes 26 organs, 31 cancer types, and a wide variety of tissue obtained from 14 heterogeneous patient cohorts, totaling more than 5 million patches obtained from over 41K WSIs viewed under various magnification levels. We systematically evaluate existing histopathology VLMs on Histo-VL to simulate diverse tasks performed by experts in real-world clinical scenarios. Our analysis reveals interesting findings, including large sensitivity of most existing histopathology VLMs to textual changes with a drop in balanced accuracy of up to 25% in tasks such as Metastasis detection, low robustness to adversarial attacks, as well as improper calibration of models evident through high ECE values and low model prediction confidence, all of which can affect their clinical implementation.
Via
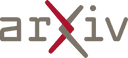
Jan 21, 2025
Abstract:Breast cancer is one of the deadliest cancers causing about massive number of patients to die annually all over the world according to the WHO. It is a kind of cancer that develops when the tissues of the breast grow rapidly and unboundly. This fatality rate can be prevented if the cancer is detected before it gets malignant. Using automation for early-age detection of breast cancer, Artificial Intelligence and Machine Learning technologies can be implemented for the best outcome. In this study, we are using the Breast Cancer Image Classification dataset collected from the Kaggle depository, which comprises 9248 Breast Ultrasound Images and is classified into three categories: Benign, Malignant, and Normal which refers to non-cancerous, cancerous, and normal images.This research introduces three pretrained model featuring custom classifiers that includes ResNet50, MobileNet, and VGG16, along with a custom CNN model utilizing the ReLU activation function.The models ResNet50, MobileNet, VGG16, and a custom CNN recorded accuracies of 98.41%, 97.91%, 98.19%, and 92.94% on the dataset, correspondingly, with ResNet50 achieving the highest accuracy of 98.41%.This model, with its deep and powerful architecture, is particularly successful in detecting aberrant cells as well as cancerous or non-cancerous tumors. These accuracies show that the Machine Learning methods are more compatible for the classification and early detection of breast cancer.
Via
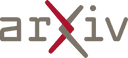