What is autonomous cars? Autonomous cars are self-driving vehicles that use artificial intelligence (AI) and sensors to navigate and operate without human intervention, using high-resolution cameras and lidars that detect what happens in the car's immediate surroundings. They have the potential to revolutionize transportation by improving safety, efficiency, and accessibility.
Papers and Code
May 19, 2024
Abstract:This work addresses the inherited limitations in the current state-of-the-art 3D multi-object tracking (MOT) methods that follow the tracking-by-detection paradigm, notably trajectory estimation drift for long-occluded objects in LiDAR point cloud streams acquired by autonomous cars. In addition, the absence of adequate track legitimacy verification results in ghost track accumulation. To tackle these issues, we introduce a two-fold innovation. Firstly, we propose refinement in Kalman filter that enhances trajectory drift noise mitigation, resulting in more robust state estimation for occluded objects. Secondly, we propose a novel online track validity mechanism to distinguish between legitimate and ghost tracks combined with a multi-stage observational gating process for incoming observations. This mechanism substantially reduces ghost tracks by up to 80\% and improves HOTA by 7\%. Accordingly, we propose an online 3D MOT framework, RobMOT, that demonstrates superior performance over the top-performing state-of-the-art methods, including deep learning approaches, across various detectors with up to 3.28\% margin in MOTA and 2.36\% in HOTA. RobMOT excels under challenging conditions, such as prolonged occlusions and the tracking of distant objects, with up to 59\% enhancement in processing latency.
Via
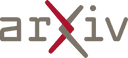
Sep 02, 2024
Abstract:The primary goal of traffic accident anticipation is to foresee potential accidents in real time using dashcam videos, a task that is pivotal for enhancing the safety and reliability of autonomous driving technologies. In this study, we introduce an innovative framework, AccNet, which significantly advances the prediction capabilities beyond the current state-of-the-art (SOTA) 2D-based methods by incorporating monocular depth cues for sophisticated 3D scene modeling. Addressing the prevalent challenge of skewed data distribution in traffic accident datasets, we propose the Binary Adaptive Loss for Early Anticipation (BA-LEA). This novel loss function, together with a multi-task learning strategy, shifts the focus of the predictive model towards the critical moments preceding an accident. {We rigorously evaluate the performance of our framework on three benchmark datasets--Dashcam Accident Dataset (DAD), Car Crash Dataset (CCD), and AnAn Accident Detection (A3D), and DADA-2000 Dataset--demonstrating its superior predictive accuracy through key metrics such as Average Precision (AP) and mean Time-To-Accident (mTTA).
Via
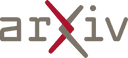
Aug 19, 2024
Abstract:As autonomous vehicle technology advances, the precise assessment of safety in complex traffic scenarios becomes crucial, especially in mixed-vehicle environments where human perception of safety must be taken into account. This paper presents a framework designed for assessing traffic safety in multi-vehicle situations, facilitating the simultaneous utilization of diverse objective safety metrics. Additionally, it allows the integration of subjective perception of safety by adjusting model parameters. The framework was applied to evaluate various model configurations in car-following scenarios on a highway, utilizing naturalistic driving datasets. The evaluation of the model showed an outstanding performance, particularly when integrating multiple objective safety measures. Furthermore, the performance was significantly enhanced when considering all surrounding vehicles.
Via
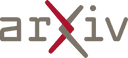
Jul 01, 2024
Abstract:With the rapid advancement of autonomous driving technology, self-driving cars have become a central focus in the development of future transportation systems. Scenario generation technology has emerged as a crucial tool for testing and verifying the safety performance of autonomous driving systems. Current research in scenario generation primarily focuses on open roads such as highways, with relatively limited studies on underground parking garages. The unique structural constraints, insufficient lighting, and high-density obstacles in underground parking garages impose greater demands on the perception systems, which are critical to autonomous driving technology. This study proposes an accelerated generation method for perception failure scenarios tailored to the underground parking garage environment, aimed at testing and improving the safety performance of autonomous vehicle (AV) perception algorithms in such settings. The method presented in this paper generates an intelligent testing environment with a high density of perception failure scenarios by learning the interactions between background vehicles (BVs) and autonomous vehicles (AVs) within perception failure scenarios. Furthermore, this method edits the Markov process within the perception failure scenario data to increase the density of critical information in the training data, thereby optimizing the learning and generation of perception failure scenarios. A simulation environment for an underground parking garage was developed using the Carla and Vissim platforms, with Bevfusion employed as the perception algorithm for testing. The study demonstrates that this method can generate an intelligent testing environment with a high density of perception failure scenarios and enhance the safety performance of perception algorithms within this experimental setup.
Via
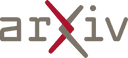
Sep 05, 2024
Abstract:In the evolving landscape of urban mobility, the prospective integration of Connected and Automated Vehicles (CAVs) with Human-Driven Vehicles (HDVs) presents a complex array of challenges and opportunities for autonomous driving systems. While recent advancements in robotics have yielded Multi-Agent Path Finding (MAPF) algorithms tailored for agent coordination task characterized by simplified kinematics and complete control over agent behaviors, these solutions are inapplicable in mixed-traffic environments where uncontrollable HDVs must coexist and interact with CAVs. Addressing this gap, we propose the Behavior Prediction Kinematic Priority Based Search (BK-PBS), which leverages an offline-trained conditional prediction model to forecast HDV responses to CAV maneuvers, integrating these insights into a Priority Based Search (PBS) where the A* search proceeds over motion primitives to accommodate kinematic constraints. We compare BK-PBS with CAV planning algorithms derived by rule-based car-following models, and reinforcement learning. Through comprehensive simulation on a highway merging scenario across diverse scenarios of CAV penetration rate and traffic density, BK-PBS outperforms these baselines in reducing collision rates and enhancing system-level travel delay. Our work is directly applicable to many scenarios of multi-human multi-robot coordination.
Via
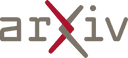
Aug 28, 2024
Abstract:Velocity estimation is of great importance in autonomous racing. Still, existing solutions are characterized by limited accuracy, especially in the case of aggressive driving or poor generalization to unseen road conditions. To address these issues, we propose to utilize Unscented Kalman Filter (UKF) with a learned dynamics model that is optimized directly for the state estimation task. Moreover, we propose to aid this model with the online-estimated friction coefficient, which increases the estimation accuracy and enables zero-shot adaptation to the new road conditions. To evaluate the UKF-based velocity estimator with the proposed dynamics model, we introduced a publicly available dataset of aggressive manoeuvres performed by an F1TENTH car, with sideslip angles reaching 40{\deg}. Using this dataset, we show that learning the dynamics model through UKF leads to improved estimation performance and that the proposed solution outperforms state-of-the-art learning-based state estimators by 17% in the nominal scenario. Moreover, we present unseen zero-shot adaptation abilities of the proposed method to the new road surface thanks to the use of the proposed learning-based tire dynamics model with online friction estimation.
Via
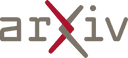
Aug 03, 2024
Abstract:Maps are essential for diverse applications, such as vehicle navigation and autonomous robotics. Both require spatial models for effective route planning and localization. This paper addresses the challenge of road graph construction for autonomous vehicles. Despite recent advances, creating a road graph remains labor-intensive and has yet to achieve full automation. The goal of this paper is to generate such graphs automatically and accurately. Modern cars are equipped with onboard sensors used for today's advanced driver assistance systems like lane keeping. We propose using global navigation satellite system (GNSS) traces and basic image data acquired from these standard sensors in consumer vehicles to estimate road-level maps with minimal effort. We exploit the spatial information in the data by framing the problem as a road centerline semantic segmentation task using a convolutional neural network. We also utilize the data's time series nature to refine the neural network's output by using map matching. We implemented and evaluated our method using a fleet of real consumer vehicles, only using the deployed onboard sensors. Our evaluation demonstrates that our approach not only matches existing methods on simpler road configurations but also significantly outperforms them on more complex road geometries and topologies. This work received the 2023 Woven by Toyota Invention Award.
* This work will be presented at IROS 2024. Supplementary website:
https://bazs.github.io/probe2road/
Via
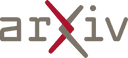
Jul 08, 2024
Abstract:This paper presents a study on autonomous robot navigation, focusing on three key behaviors: Odometry, Target Tracking, and Obstacle Avoidance. Each behavior is described in detail, along with experimental setups for simulated and real-world environments. Odometry utilizes wheel encoder data for precise navigation along predefined paths, validated through experiments with a Pioneer robot. Target Tracking employs vision-based techniques for pursuing designated targets while avoiding obstacles, demonstrated on the same platform. Obstacle Avoidance utilizes ultrasonic sensors to navigate cluttered environments safely, validated in both simulated and real-world scenarios. Additionally, the paper extends the project to include an Elegoo robot car, leveraging its features for enhanced experimentation. Through advanced algorithms and experimental validations, this study provides insights into developing robust navigation systems for autonomous robots.
Via
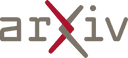
Jul 09, 2024
Abstract:Trajectory planners of autonomous vehicles usually rely on physical models to predict the vehicle behavior. However, despite their suitability, physical models have some shortcomings. On the one hand, simple models suffer from larger model errors and more restrictive assumptions. On the other hand, complex models are computationally more demanding and depend on environmental and operational parameters. In each case, the drawbacks can be associated to a certain degree to the physical modeling of the yaw rate dynamics. Therefore, this paper investigates the yaw rate prediction based on conditional neural processes (CNP), a data-driven meta-learning approach, to simultaneously achieve low errors, adequate complexity and robustness to varying parameters. Thus, physical models can be enhanced in a targeted manner to provide accurate and computationally efficient predictions to enable safe planning in autonomous vehicles. High fidelity simulations for a variety of driving scenarios and different types of cars show that CNP makes it possible to employ and transfer knowledge about the yaw rate based on current driving dynamics in a human-like manner, yielding robustness against changing environmental and operational conditions.
* 2023 62nd IEEE IEEE Conference on Decision and Control (CDC),
Singapore, Singapore, December 13 - 15, 2023, pp. 322--327
* Published in 2023 62nd IEEE IEEE Conference on Decision and Control
(CDC), Singapore, Singapore, December 13 - 15, 2023
Via
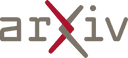
Aug 08, 2024
Abstract:This paper presents a 1/10th scale mini-city platform used as a testing bed for evaluating autonomous and connected vehicles. Using the mini-city platform, we can evaluate different driving scenarios including human-driven and autonomous driving. We provide a unique, visual feature-rich environment for evaluating computer vision methods. The conducted experiments utilize onboard sensors mounted on a robotic platform we built, allowing them to navigate in a controlled real-world urban environment. The designed city is occupied by cars, stop signs, a variety of residential and business buildings, and complex intersections mimicking an urban area. Furthermore, We have designed an intelligent infrastructure at one of the intersections in the city which helps safer and more efficient navigation in the presence of multiple cars and pedestrians. We have used the mini-city platform for the analysis of three different applications: city mapping, depth estimation in challenging occluded environments, and smart infrastructure for connected vehicles. Our smart infrastructure is among the first to develop and evaluate Vehicle-to-Infrastructure (V2I) communication at intersections. The intersection-related result shows how inaccuracy in perception, including mapping and localization, can affect safety. The proposed mini-city platform can be considered as a baseline environment for developing research and education in intelligent transportation systems.
* 8 pages, 9 figures, Presented at 2024 IEEE ITSC Conference, 23
Citations
Via
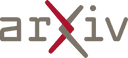