Jun 06, 2025
Abstract:Multimodal Large Language Models (MLLMs) have demonstrated remarkable capabilities in automated front-end engineering, e.g., generating UI code from visual designs. However, existing front-end UI code generation benchmarks have the following limitations: (1) While framework-based development becomes predominant in modern front-end programming, current benchmarks fail to incorporate mainstream development frameworks. (2) Existing evaluations focus solely on the UI code generation task, whereas practical UI development involves several iterations, including refining editing, and repairing issues. (3) Current benchmarks employ unidimensional evaluation, lacking investigation into influencing factors like task difficulty, input context variations, and in-depth code-level analysis. To bridge these gaps, we introduce DesignBench, a multi-framework, multi-task evaluation benchmark for assessing MLLMs' capabilities in automated front-end engineering. DesignBench encompasses three widely-used UI frameworks (React, Vue, and Angular) alongside vanilla HTML/CSS, and evaluates on three essential front-end tasks (generation, edit, and repair) in real-world development workflows. DesignBench contains 900 webpage samples spanning over 11 topics, 9 edit types, and 6 issue categories, enabling detailed analysis of MLLM performance across multiple dimensions. Our systematic evaluation reveals critical insights into MLLMs' framework-specific limitations, task-related bottlenecks, and performance variations under different conditions, providing guidance for future research in automated front-end development. Our code and data are available at https://github.com/WebPAI/DesignBench.
Via
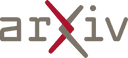