Topic:Time Series Analysis
What is Time Series Analysis? Time series analysis comprises statistical methods for analyzing a sequence of data points collected over an interval of time to identify interesting patterns and trends.
Papers and Code
Feb 26, 2025
Abstract:Time series data, defined by equally spaced points over time, is essential in fields like medicine, telecommunications, and energy. Analyzing it involves tasks such as classification, clustering, prototyping, and regression. Classification identifies normal vs. abnormal movements in skeleton-based motion sequences, clustering detects stock market behavior patterns, prototyping expands physical therapy datasets, and regression predicts patient recovery. Deep learning has recently gained traction in time series analysis due to its success in other domains. This thesis leverages deep learning to enhance classification with feature engineering, introduce foundation models, and develop a compact yet state-of-the-art architecture. We also address limited labeled data with self-supervised learning. Our contributions apply to real-world tasks, including human motion analysis for action recognition and rehabilitation. We introduce a generative model for human motion data, valuable for cinematic production and gaming. For prototyping, we propose a shape-based synthetic sample generation method to support regression models when data is scarce. Lastly, we critically evaluate discriminative and generative models, identifying limitations in current methodologies and advocating for a robust, standardized evaluation framework. Our experiments on public datasets provide novel insights and methodologies, advancing time series analysis with practical applications.
Via
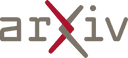
Mar 19, 2025
Abstract:With the prevalence of sensor failures, imputation--the process of estimating missing values--has emerged as the cornerstone of time series data preparation. While numerous imputation algorithms have been developed to address these data gaps, existing libraries provide limited support. Furthermore, they often lack the ability to simulate realistic patterns of time series missing data and fail to account for the impact of imputation on subsequent downstream analysis. This paper introduces ImputeGAP, a comprehensive library for time series imputation that supports a diverse range of imputation methods and modular missing data simulation catering to datasets with varying characteristics. The library includes extensive customization options, such as automated hyperparameter tuning, benchmarking, explainability, downstream evaluation, and compatibility with popular time series frameworks.
Via
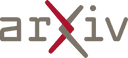
Mar 13, 2025
Abstract:Time-series analysis is critical for a wide range of fields such as healthcare, finance, transportation, and energy, among many others. The practical applications often involve analyzing time-series data alongside contextual information in the form of natural language to support informed decisions. However, current time-series models are limited in their ability to perform reasoning that involves both time-series and their textual content. In this work, we address this gap by introducing \textit{Chat-TS}, a large language model (LLM) based framework, designed to support reasoning over time series and textual data. Unlike traditional models, Chat-TS integrates time-series tokens into LLMs' vocabulary, enhancing its reasoning ability over both modalities without compromising the core natural language capabilities, enabling practical analysis and reasoning across modalities. To support learning and evaluation in this setup, we contribute new datasets: the \textit{TS Instruct Training Dataset} which pairs diverse time-series data with relevant text instructions and responses for instruction tuning, the \textit{TS Instruct Question and Answer (QA) Gold Dataset} which provides multiple-choice questions designed to evaluate multimodal reasoning, and a \textit{TS Instruct Quantitative Probing Set} which contains a small subset of the TS Instruct QA tasks alongside math and decision-making questions for LLM evaluation. We designed a training strategy to preserve the inherent reasoning capabilities of LLMs while augmenting them for time-series reasoning. Experiments show that Chat-TS achieves state-of-the-art performance in multi-modal reasoning tasks by maintaining strong natural language proficiency while improving time-series reasoning. ~\footnote{To ensure replicability and facilitate future research, all models, datasets, and code will be available at [\texttt{Github-URL}].}
Via
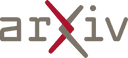
Apr 04, 2025
Abstract:Given the growing environmental challenges, accurate monitoring and prediction of changes in water bodies are essential for sustainable management and conservation. The Continuous Monitoring of Land Disturbance (COLD) algorithm provides a valuable tool for real-time analysis of land changes, such as deforestation, urban expansion, agricultural activities, and natural disasters. This capability enables timely interventions and more informed decision-making. This paper assesses the effectiveness of the algorithm to estimate water bodies and track pixel-level water trends over time. Our findings indicate that COLD-derived data can reliably estimate estimate water frequency during stable periods and delineate water bodies. Furthermore, it enables the evaluation of trends in water areas after disturbances, allowing for the determination of whether water frequency increases, decreases, or remains constant.
Via
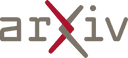
Mar 10, 2025
Abstract:With the exponential growth of time series data across diverse domains, there is a pressing need for effective analysis tools. Time series clustering is important for identifying patterns in these datasets. However, prevailing methods often encounter obstacles in maintaining data relationships and ensuring interpretability. We present Graphint, an innovative system based on the $k$-Graph methodology that addresses these challenges. Graphint integrates a robust time series clustering algorithm with an interactive tool for comparison and interpretation. More precisely, our system allows users to compare results against competing approaches, identify discriminative subsequences within specified datasets, and visualize the critical information utilized by $k$-Graph to generate outputs. Overall, Graphint offers a comprehensive solution for extracting actionable insights from complex temporal datasets.
Via
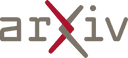
Mar 10, 2025
Abstract:Time series analysis is crucial in fields like finance, transportation, and industry. However, traditional models often focus solely on temporal features, limiting their ability to capture underlying information. This paper proposes a novel time series multitask framework, called LTM, which integrates temporal features with textual descriptions to enhance analytical and predictive capabilities. LTM combines pre-trained time series model, large language model (LLM), and knowledge graph to tackle time series tasks, including forecasting, imputation, and anomaly detection. LTM achieves improved performance with a few trainable parameters. It is very efficient and practical. LTM encodes time series data into patches and enriches user-provided prompts using knowledge graphs to generate enhanced prompts. A novel feature fusion method embeds prompts into each patch encoding, which is processed by a frozen LLM, followed by a feature enhancement module and a time decoder module. During fine-tuning stage, cosine similarity between prompts and temporal patches is integrated into the loss function to boost performance. Experiments on benchmark datasets show that LTM significantly outperforms existing methods. It provides a robust and versatile solution for time series tasks.
Via
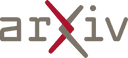
Feb 28, 2025
Abstract:The success of foundation models in natural language processing and computer vision has motivated similar approaches for general time series analysis. While these models are effective for a variety of tasks, their applicability in medical domains with limited data remains largely unexplored. To address this, we investigate the effectiveness of foundation models in medical time series analysis involving electroencephalography (EEG). Through extensive experiments on tasks such as age prediction, seizure detection, and the classification of clinically relevant EEG events, we compare their diagnostic accuracy with that of specialised EEG models. Our analysis shows that foundation models extract meaningful EEG features, outperform specialised models even without domain adaptation, and localise task-specific biomarkers. Moreover, we demonstrate that diagnostic accuracy is substantially influenced by architectural choices such as context length. Overall, our study reveals that foundation models with general time series understanding eliminate the dependency on large domain-specific datasets, making them valuable tools for clinical practice.
Via
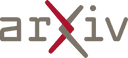
Mar 03, 2025
Abstract:In continual time series analysis using neural networks, catastrophic forgetting (CF) of previously learned models when training on new data domains has always been a significant challenge. This problem is especially challenging in vehicle estimation and control, where new information is sequentially introduced to the model. Unfortunately, existing work on continual learning has not sufficiently addressed the adverse effects of catastrophic forgetting in time series analysis, particularly in multivariate output environments. In this paper, we present EM-ReSeleCT (Efficient Multivariate Representative Selection for Continual Learning in Time Series Tasks), an enhanced approach designed to handle continual learning in multivariate environments. Our approach strategically selects representative subsets from old and historical data and incorporates memory-based continual learning techniques with an improved optimization algorithm to adapt the pre-trained model on new information while preserving previously acquired information. Additionally, we develop a sequence-to-sequence transformer model (autoregressive model) specifically designed for vehicle state estimation. Moreover, we propose an uncertainty quantification framework using conformal prediction to assess the sensitivity of the memory size and to showcase the robustness of the proposed method. Experimental results from tests on an electric Equinox vehicle highlight the superiority of our method in continually learning new information while retaining prior knowledge, outperforming state-of-the-art continual learning methods. Furthermore, EM-ReSeleCT significantly reduces training time, a critical advantage in continual learning applications.
Via
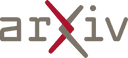
Feb 21, 2025
Abstract:Foundation models for time series analysis (TSA) have attracted significant attention. However, challenges such as data scarcity and data imbalance continue to hinder their development. To address this, we consider modeling complex systems through symbolic expressions that serve as semantic descriptors of time series. Building on this concept, we introduce a series-symbol (S2) dual-modulity data generation mechanism, enabling the unrestricted creation of high-quality time series data paired with corresponding symbolic representations. Leveraging the S2 dataset, we develop SymTime, a pre-trained foundation model for TSA. SymTime demonstrates competitive performance across five major TSA tasks when fine-tuned with downstream task, rivaling foundation models pre-trained on real-world datasets. This approach underscores the potential of dual-modality data generation and pretraining mechanisms in overcoming data scarcity and enhancing task performance.
Via
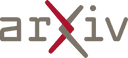
Mar 21, 2025
Abstract:Understanding the relationship between textual news and time-series evolution is a critical yet under-explored challenge in applied data science. While multimodal learning has gained traction, existing multimodal time-series datasets fall short in evaluating cross-modal reasoning and complex question answering, which are essential for capturing complex interactions between narrative information and temporal patterns. To bridge this gap, we introduce Multimodal Time Series Benchmark (MTBench), a large-scale benchmark designed to evaluate large language models (LLMs) on time series and text understanding across financial and weather domains. MTbench comprises paired time series and textual data, including financial news with corresponding stock price movements and weather reports aligned with historical temperature records. Unlike existing benchmarks that focus on isolated modalities, MTbench provides a comprehensive testbed for models to jointly reason over structured numerical trends and unstructured textual narratives. The richness of MTbench enables formulation of diverse tasks that require a deep understanding of both text and time-series data, including time-series forecasting, semantic and technical trend analysis, and news-driven question answering (QA). These tasks target the model's ability to capture temporal dependencies, extract key insights from textual context, and integrate cross-modal information. We evaluate state-of-the-art LLMs on MTbench, analyzing their effectiveness in modeling the complex relationships between news narratives and temporal patterns. Our findings reveal significant challenges in current models, including difficulties in capturing long-term dependencies, interpreting causality in financial and weather trends, and effectively fusing multimodal information.
* 14 pages
Via
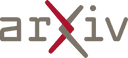