Topic:Text Classification
What is Text Classification? Text classification is the process of categorizing text documents into predefined categories or labels.
Papers and Code
May 08, 2025
Abstract:The concept of sharpness has been successfully applied to traditional architectures like MLPs and CNNs to predict their generalization. For transformers, however, recent work reported weak correlation between flatness and generalization. We argue that existing sharpness measures fail for transformers, because they have much richer symmetries in their attention mechanism that induce directions in parameter space along which the network or its loss remain identical. We posit that sharpness must account fully for these symmetries, and thus we redefine it on a quotient manifold that results from quotienting out the transformer symmetries, thereby removing their ambiguities. Leveraging tools from Riemannian geometry, we propose a fully general notion of sharpness, in terms of a geodesic ball on the symmetry-corrected quotient manifold. In practice, we need to resort to approximating the geodesics. Doing so up to first order yields existing adaptive sharpness measures, and we demonstrate that including higher-order terms is crucial to recover correlation with generalization. We present results on diagonal networks with synthetic data, and show that our geodesic sharpness reveals strong correlation for real-world transformers on both text and image classification tasks.
Via
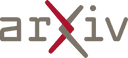
May 16, 2025
Abstract:This paper presents an end-to-end suite for multilingual information extraction and processing from image-based documents. The system uses Optical Character Recognition (Tesseract) to extract text in languages such as English, Hindi, and Tamil, and then a pipeline involving large language model APIs (Gemini) for cross-lingual translation, abstractive summarization, and re-translation into a target language. Additional modules add sentiment analysis (TensorFlow), topic classification (Transformers), and date extraction (Regex) for better document comprehension. Made available in an accessible Gradio interface, the current research shows a real-world application of libraries, models, and APIs to close the language gap and enhance access to information in image media across different linguistic environments
* 8 pages, 7 figures, direct arXiv submission
Via
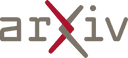
May 18, 2025
Abstract:We introduce the first method for translating text embeddings from one vector space to another without any paired data, encoders, or predefined sets of matches. Our unsupervised approach translates any embedding to and from a universal latent representation (i.e., a universal semantic structure conjectured by the Platonic Representation Hypothesis). Our translations achieve high cosine similarity across model pairs with different architectures, parameter counts, and training datasets. The ability to translate unknown embeddings into a different space while preserving their geometry has serious implications for the security of vector databases. An adversary with access only to embedding vectors can extract sensitive information about the underlying documents, sufficient for classification and attribute inference.
Via
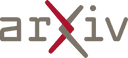
May 19, 2025
Abstract:Despite major advances in surgical brain-to-text (B2T), i.e. transcribing speech from invasive brain recordings, non-invasive alternatives have yet to surpass even chance on standard metrics. This remains a barrier to building a non-invasive brain-computer interface (BCI) capable of restoring communication in paralysed individuals without surgery. Here, we present the first non-invasive B2T result that significantly exceeds these critical baselines, raising BLEU by $1.4\mathrm{-}2.6\times$ over prior work. This result is driven by three contributions: (1) we extend recent word-classification models with LLM-based rescoring, transforming single-word predictors into closed-vocabulary B2T systems; (2) we introduce a predictive in-filling approach to handle out-of-vocabulary (OOV) words, substantially expanding the effective vocabulary; and (3) we demonstrate, for the first time, how to scale non-invasive B2T models across datasets, unlocking deep learning at scale and improving accuracy by $2.1\mathrm{-}2.3\times$. Through these contributions, we offer new insights into the roles of data quality and vocabulary size. Together, our results remove a major obstacle to realising practical non-invasive B2T systems.
* 27 pages, 10 figures, 10 tables. Under review
Via
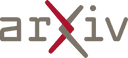
May 16, 2025
Abstract:Recently, large-scale pre-trained speech encoders and Large Language Models (LLMs) have been released, which show state-of-the-art performance on a range of spoken language processing tasks including Automatic Speech Recognition (ASR). To effectively combine both models for better performance, continuous speech prompts, and ASR error correction have been adopted. However, these methods are prone to suboptimal performance or are inflexible. In this paper, we propose a new paradigm, LegoSLM, that bridges speech encoders and LLMs using the ASR posterior matrices. The speech encoder is trained to generate Connectionist Temporal Classification (CTC) posteriors over the LLM vocabulary, which are used to reconstruct pseudo-audio embeddings by computing a weighted sum of the LLM input embeddings. These embeddings are concatenated with text embeddings in the LLM input space. Using the well-performing USM and Gemma models as an example, we demonstrate that our proposed LegoSLM method yields good performance on both ASR and speech translation tasks. By connecting USM with Gemma models, we can get an average of 49% WERR over the USM-CTC baseline on 8 MLS testsets. The trained model also exhibits modularity in a range of settings -- after fine-tuning the Gemma model weights, the speech encoder can be switched and combined with the LLM in a zero-shot fashion. Additionally, we propose to control the decode-time influence of the USM and LLM using a softmax temperature, which shows effectiveness in domain adaptation.
Via
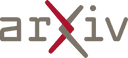
May 21, 2025
Abstract:Effective cross-lingual transfer remains a critical challenge in scaling the benefits of large language models from high-resource to low-resource languages. Towards this goal, prior studies have explored many approaches to combine task knowledge from task-specific data in a (high-resource) source language and language knowledge from unlabeled text in a (low-resource) target language. One notable approach proposed composable sparse fine-tuning (SFT) for cross-lingual transfer that learns task-specific and language-specific sparse masks to select a subset of the pretrained model's parameters that are further fine-tuned. These sparse fine-tuned vectors (SFTs) are subsequently composed with the pretrained model to facilitate zero-shot cross-lingual transfer to a task in a target language, using only task-specific data from a source language. These sparse masks for SFTs were identified using a simple magnitude-based pruning. In our work, we introduce DeFT-X, a novel composable SFT approach that denoises the weight matrices of a pretrained model before magnitude pruning using singular value decomposition, thus yielding more robust SFTs. We evaluate DeFT-X on a diverse set of extremely low-resource languages for sentiment classification (NusaX) and natural language inference (AmericasNLI) and demonstrate that it performs at par or outperforms SFT and other prominent cross-lingual transfer baselines.
Via
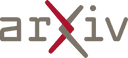
May 13, 2025
Abstract:Metabolic syndrome (MetS) is a medication condition characterized by abdominal obesity, insulin resistance, hypertension and hyperlipidemia. It increases the risk of majority of chronic diseases, including type 2 diabetes mellitus, and affects about one quarter of the global population. Therefore, early detection and timely intervention for MetS are crucial. Standard diagnosis for MetS components requires blood tests conducted within medical institutions. However, it is frequently underestimated, leading to unmet need for care for MetS population. This study aims to use the least physiological data and free texts about exercises related activities, which are obtained easily in daily life, to diagnosis MetS. We collected the data from 40 volunteers in a nursing home and used data augmentation to reduce the imbalance. We propose a deep learning framework for classifying MetS that integrates natural language processing (NLP) and exercise monitoring. The results showed that the best model reported a high positive result (AUROC=0.806 and REC=76.3%) through 3-fold cross-validation. Feature importance analysis revealed that text and minimum heart rate on a daily basis contribute the most in the classification of MetS. This study demonstrates the potential application of data that are easily measurable in daily life for the early diagnosis of MetS, which could contribute to reducing the cost of screening and management for MetS population.
Via
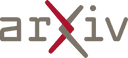
May 14, 2025
Abstract:Granite-speech LLMs are compact and efficient speech language models specifically designed for English ASR and automatic speech translation (AST). The models were trained by modality aligning the 2B and 8B parameter variants of granite-3.3-instruct to speech on publicly available open-source corpora containing audio inputs and text targets consisting of either human transcripts for ASR or automatically generated translations for AST. Comprehensive benchmarking shows that on English ASR, which was our primary focus, they outperform several competitors' models that were trained on orders of magnitude more proprietary data, and they keep pace on English-to-X AST for major European languages, Japanese, and Chinese. The speech-specific components are: a conformer acoustic encoder using block attention and self-conditioning trained with connectionist temporal classification, a windowed query-transformer speech modality adapter used to do temporal downsampling of the acoustic embeddings and map them to the LLM text embedding space, and LoRA adapters to further fine-tune the text LLM. Granite-speech-3.3 operates in two modes: in speech mode, it performs ASR and AST by activating the encoder, projector, and LoRA adapters; in text mode, it calls the underlying granite-3.3-instruct model directly (without LoRA), essentially preserving all the text LLM capabilities and safety. Both models are freely available on HuggingFace (https://huggingface.co/ibm-granite/granite-speech-3.3-2b and https://huggingface.co/ibm-granite/granite-speech-3.3-8b) and can be used for both research and commercial purposes under a permissive Apache 2.0 license.
* 7 pages, 9 figures
Via
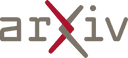
May 14, 2025
Abstract:The success of deep learning in computer vision over the past decade has hinged on large labeled datasets and strong pretrained models. In data-scarce settings, the quality of these pretrained models becomes crucial for effective transfer learning. Image classification and self-supervised learning have traditionally been the primary methods for pretraining CNNs and transformer-based architectures. Recently, the rise of text-to-image generative models, particularly those using denoising diffusion in a latent space, has introduced a new class of foundational models trained on massive, captioned image datasets. These models' ability to generate realistic images of unseen content suggests they possess a deep understanding of the visual world. In this work, we present Marigold, a family of conditional generative models and a fine-tuning protocol that extracts the knowledge from pretrained latent diffusion models like Stable Diffusion and adapts them for dense image analysis tasks, including monocular depth estimation, surface normals prediction, and intrinsic decomposition. Marigold requires minimal modification of the pre-trained latent diffusion model's architecture, trains with small synthetic datasets on a single GPU over a few days, and demonstrates state-of-the-art zero-shot generalization. Project page: https://marigoldcomputervision.github.io
* Journal extension of our CVPR 2024 paper, featuring new tasks,
improved efficiency, high-resolution capabilities, and enhanced accessibility
Via
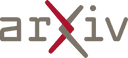
May 16, 2025
Abstract:Minimally invasive surgery (MIS) presents significant visual and technical challenges, including surgical instrument classification and understanding surgical action involving instruments, verbs, and anatomical targets. While many machine learning-based methods have been developed for surgical understanding, they typically rely on procedure- and task-specific models trained on small, manually annotated datasets. In contrast, the recent success of vision-language models (VLMs) trained on large volumes of raw image-text pairs has demonstrated strong adaptability to diverse visual data and a range of downstream tasks. This opens meaningful research questions: how well do these general-purpose VLMs perform in the surgical domain? In this work, we explore those questions by benchmarking several VLMs across diverse surgical datasets, including general laparoscopic procedures and endoscopic submucosal dissection, to assess their current capabilities and limitations. Our benchmark reveals key gaps in the models' ability to consistently link language to the correct regions in surgical scenes.
Via
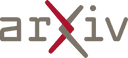