What is Recommendation? Recommendation is the task of providing personalized suggestions to users based on their preferences and behavior.
Papers and Code
Jun 14, 2025
Abstract:Recent work has questioned the reliability of graph learning benchmarks, citing concerns around task design, methodological rigor, and data suitability. In this extended abstract, we contribute to this discussion by focusing on evaluation strategies in Temporal Link Prediction (TLP). We observe that current evaluation protocols are often affected by one or more of the following issues: (1) inconsistent sampled metrics, (2) reliance on hard negative sampling often introduced as a means to improve robustness, and (3) metrics that implicitly assume equal base probabilities across source nodes by combining predictions. We support these claims through illustrative examples and connections to longstanding concerns in the recommender systems community. Our ongoing work aims to systematically characterize these problems and explore alternatives that can lead to more robust and interpretable evaluation. We conclude with a discussion of potential directions for improving the reliability of TLP benchmarks.
Via
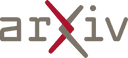
Jun 14, 2025
Abstract:In this work we focus on improving language models' understanding for asset maintenance to guide the engineer's decisions and minimize asset downtime. Given a set of tasks expressed in natural language for Industry 4.0 domain, each associated with queries related to a specific asset, we want to recommend relevant items and generalize to queries of similar assets. A task may involve identifying relevant sensors given a query about an asset's failure mode. Our approach begins with gathering a qualitative, expert-vetted knowledge base to construct nine asset-specific task datasets. To create more contextually informed embeddings, we augment the input tasks using Large Language Models (LLMs), providing concise descriptions of the entities involved in the queries. This embedding model is then integrated with a Reasoning and Acting agent (ReAct), which serves as a powerful tool for answering complex user queries that require multi-step reasoning, planning, and knowledge inference. Through ablation studies, we demonstrate that: (a) LLM query augmentation improves the quality of embeddings, (b) Contrastive loss and other methods that avoid in-batch negatives are superior for datasets with queries related to many items, and (c) It is crucial to balance positive and negative in-batch samples. After training and testing on our dataset, we observe a substantial improvement: HIT@1 increases by +54.2%, MAP@100 by +50.1%, and NDCG@10 by +54.7%, averaged across all tasks and models. Additionally, we empirically demonstrate the model's planning and tool invocation capabilities when answering complex questions related to industrial asset maintenance, showcasing its effectiveness in supporting Subject Matter Experts (SMEs) in their day-to-day operations.
Via
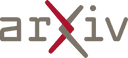
Jun 13, 2025
Abstract:Fleets of networked manufacturing machines of the same type, that are collocated or geographically distributed, are growing in popularity. An excellent example is the rise of 3D printing farms, which consist of multiple networked 3D printers operating in parallel, enabling faster production and efficient mass customization. However, optimizing process parameters across a fleet of manufacturing machines, even of the same type, remains a challenge due to machine-to-machine variability. Traditional trial-and-error approaches are inefficient, requiring extensive testing to determine optimal process parameters for an entire fleet. In this work, we introduce a machine learning-based collaborative recommender system that optimizes process parameters for each machine in a fleet by modeling the problem as a sequential matrix completion task. Our approach leverages spectral clustering and alternating least squares to iteratively refine parameter predictions, enabling real-time collaboration among the machines in a fleet while minimizing the number of experimental trials. We validate our method using a mini 3D printing farm consisting of ten 3D printers for which we optimize acceleration and speed settings to maximize print quality and productivity. Our approach achieves significantly faster convergence to optimal process parameters compared to non-collaborative matrix completion.
* 26 pages, 6 figures
Via
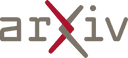
Jun 13, 2025
Abstract:With the rapid growth of Internet services, recommendation systems play a central role in delivering personalized content. Faced with massive user requests and complex model architectures, the key challenge for real-time recommendation systems is how to reduce inference latency and increase system throughput without sacrificing recommendation quality. This paper addresses the high computational cost and resource bottlenecks of deep learning models in real-time settings by proposing a combined set of modeling- and system-level acceleration and optimization strategies. At the model level, we dramatically reduce parameter counts and compute requirements through lightweight network design, structured pruning, and weight quantization. At the system level, we integrate multiple heterogeneous compute platforms and high-performance inference libraries, and we design elastic inference scheduling and load-balancing mechanisms based on real-time load characteristics. Experiments show that, while maintaining the original recommendation accuracy, our methods cut latency to less than 30% of the baseline and more than double system throughput, offering a practical solution for deploying large-scale online recommendation services.
Via
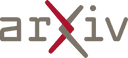
Jun 13, 2025
Abstract:Disentangling user intentions from implicit feedback has become a promising strategy to enhance recommendation accuracy and interpretability. Prior methods often model intentions independently and lack explicit supervision, thus failing to capture the joint semantics that drive user-item interactions. To address these limitations, we propose DMICF, a unified framework that explicitly models interaction-level intent alignment while leveraging structural signals from both user and item perspectives. DMICF adopts a dual-view architecture that jointly encodes user-item interaction graphs from both sides, enabling bidirectional information fusion. This design enhances robustness under data sparsity by allowing the structural redundancy of one view to compensate for the limitations of the other. To model fine-grained user-item compatibility, DMICF introduces an intent interaction encoder that performs sub-intent alignment within each view, uncovering shared semantic structures that underlie user decisions. This localized alignment enables adaptive refinement of intent embeddings based on interaction context, thus improving the model's generalization and expressiveness, particularly in long-tail scenarios. Furthermore, DMICF integrates an intent-aware scoring mechanism that aggregates compatibility signals from matched intent pairs across user and item subspaces, enabling personalized prediction grounded in semantic congruence rather than entangled representations. To facilitate semantic disentanglement, we design a discriminative training signal via multi-negative sampling and softmax normalization, which pulls together semantically aligned intent pairs while pushing apart irrelevant or noisy ones. Extensive experiments demonstrate that DMICF consistently delivers robust performance across datasets with diverse interaction distributions.
* 26 pages, 11 figures
Via
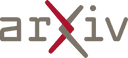
Jun 13, 2025
Abstract:The incidence rate of early-onset colorectal cancer (EoCRC, age < 45) has increased every year, but this population is younger than the recommended age established by national guidelines for cancer screening. In this paper, we applied 10 different machine learning models to predict EoCRC, and compared their performance with advanced large language models (LLM), using patient conditions, lab results, and observations within 6 months of patient journey prior to the CRC diagnoses. We retrospectively identified 1,953 CRC patients from multiple health systems across the United States. The results demonstrated that the fine-tuned LLM achieved an average of 73% sensitivity and 91% specificity.
* Paper accepted for the proceedings of the 2025 American Medical
Informatics Association Annual Symposium (AMIA)
Via
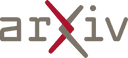
Jun 13, 2025
Abstract:A core learning challenge for existed Foundation Models (FM) is striking the tradeoff between generalization with personalization, which is a dilemma that has been highlighted by various parameter-efficient adaptation techniques. Federated foundation models (FFM) provide a structural means to decouple shared knowledge from individual specific adaptations via decentralized processes. Recommendation systems offer a perfect testbed for FFMs, given their reliance on rich implicit feedback reflecting unique user characteristics. This position paper discusses a novel learning paradigm where FFMs not only harness their generalization capabilities but are specifically designed to preserve the integrity of user personality, illustrated thoroughly within the recommendation contexts. We envision future personal agents, powered by personalized adaptive FMs, guiding user decisions on content. Such an architecture promises a user centric, decentralized system where individuals maintain control over their personalized agents.
* 14 pages, 3 figures, conference, position paper
Via
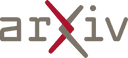
Jun 13, 2025
Abstract:Recent advances in Large Language Models (LLMs) have enabled unprecedented capabilities for time-series reasoning in diverse real-world applications, including medical, financial, and spatio-temporal domains. However, existing approaches typically focus on task-specific model customization, such as forecasting and anomaly detection, while overlooking the data itself, referred to as time-series primitives, which are essential for in-depth reasoning. This position paper advocates a fundamental shift in approaching time-series reasoning with LLMs: prioritizing alignment paradigms grounded in the intrinsic primitives of time series data over task-specific model customization. This realignment addresses the core limitations of current time-series reasoning approaches, which are often costly, inflexible, and inefficient, by systematically accounting for intrinsic structure of data before task engineering. To this end, we propose three alignment paradigms: Injective Alignment, Bridging Alignment, and Internal Alignment, which are emphasized by prioritizing different aspects of time-series primitives: domain, characteristic, and representation, respectively, to activate time-series reasoning capabilities of LLMs to enable economical, flexible, and efficient reasoning. We further recommend that practitioners adopt an alignment-oriented method to avail this instruction to select an appropriate alignment paradigm. Additionally, we categorize relevant literature into these alignment paradigms and outline promising research directions.
Via
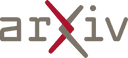
Jun 12, 2025
Abstract:Recently, Graph Neural Networks (GNNs) have become the dominant approach for Knowledge Graph-aware Recommender Systems (KGRSs) due to their proven effectiveness. Building upon GNN-based KGRSs, Self-Supervised Learning (SSL) has been incorporated to address the sparity issue, leading to longer training time. However, through extensive experiments, we reveal that: (1)compared to other KGRSs, the existing GNN-based KGRSs fail to keep their superior performance under sparse interactions even with SSL. (2) More complex models tend to perform worse in sparse interaction scenarios and complex mechanisms, like attention mechanism, can be detrimental as they often increase learning difficulty. Inspired by these findings, we propose LightKG, a simple yet powerful GNN-based KGRS to address sparsity issues. LightKG includes a simplified GNN layer that encodes directed relations as scalar pairs rather than dense embeddings and employs a linear aggregation framework, greatly reducing the complexity of GNNs. Additionally, LightKG incorporates an efficient contrastive layer to implement SSL. It directly minimizes the node similarity in original graph, avoiding the time-consuming subgraph generation and comparison required in previous SSL methods. Experiments on four benchmark datasets show that LightKG outperforms 12 competitive KGRSs in both sparse and dense scenarios while significantly reducing training time. Specifically, it surpasses the best baselines by an average of 5.8\% in recommendation accuracy and saves 84.3\% of training time compared to KGRSs with SSL. Our code is available at https://github.com/1371149/LightKG.
* Proceedings of the 31st ACM SIGKDD Conference on Knowledge Discovery
and Data Mining
Via
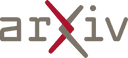
Jun 12, 2025
Abstract:Next Point-of-Interest (POI) recommendation is a critical task in location-based services, aiming to predict users' next visits based on their check-in histories. While many existing methods leverage Graph Neural Networks (GNNs) to incorporate collaborative information and improve recommendation accuracy, most of them model each type of context using separate graphs, treating different factors in isolation. This limits their ability to model the co-influence of multiple contextual factors on user transitions during message propagation, resulting in suboptimal attention weights and recommendation performance. Furthermore, they often prioritize sequential components as the primary predictor, potentially undermining the semantic and structural information encoded in the POI embeddings learned by GNNs. To address these limitations, we propose a Context-Adaptive Graph Neural Networks (CAGNN) for next POI recommendation, which dynamically adjusts attention weights using edge-specific contextual factors and enables mutual enhancement between graph-based and sequential components. Specifically, CAGNN introduces (1) a context-adaptive attention mechanism that jointly incorporates different types of contextual factors into the attention computation during graph propagation, enabling the model to dynamically capture collaborative and context-dependent transition patterns; (2) a graph-sequential mutual enhancement module, which aligns the outputs of the graph- and sequential-based modules via the KL divergence, enabling mutual enhancement of both components. Experimental results on three real-world datasets demonstrate that CAGNN consistently outperforms state-of-the-art methods. Meanwhile, theoretical guarantees are provided that our context-adaptive attention mechanism improves the expressiveness of POI representations.
* 12 pages, 6 figures
Via
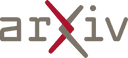