Jun 10, 2025
Abstract:The rise of large language models (LLMs) has created new possibilities for digital twins in healthcare. However, the deployment of such systems in consumer health contexts raises significant concerns related to hallucination, bias, lack of transparency, and ethical misuse. In response to recommendations from health authorities such as the World Health Organization (WHO), we propose Responsible Health Twin (RHealthTwin), a principled framework for building and governing AI-powered digital twins for well-being assistance. RHealthTwin processes multimodal inputs that guide a health-focused LLM to produce safe, relevant, and explainable responses. At the core of RHealthTwin is the Responsible Prompt Engine (RPE), which addresses the limitations of traditional LLM configuration. Conventionally, users input unstructured prompt and the system instruction to configure the LLM, which increases the risk of hallucination. In contrast, RPE extracts predefined slots dynamically to structure both inputs. This guides the language model to generate responses that are context aware, personalized, fair, reliable, and explainable for well-being assistance. The framework further adapts over time through a feedback loop that updates the prompt structure based on user satisfaction. We evaluate RHealthTwin across four consumer health domains including mental support, symptom triage, nutrition planning, and activity coaching. RPE achieves state-of-the-art results with BLEU = 0.41, ROUGE-L = 0.63, and BERTScore = 0.89 on benchmark datasets. Also, we achieve over 90% in ethical compliance and instruction-following metrics using LLM-as-judge evaluation, outperforming baseline strategies. We envision RHealthTwin as a forward-looking foundation for responsible LLM-based applications in health and well-being.
* 18 pages, 12 figures, IEEE EMBS JBHI
Via
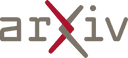