Topic:Information Extraction
What is Information Extraction? Information extraction is the process of automatically extracting structured information from unstructured text data.
Papers and Code
Jun 24, 2025
Abstract:This paper provides preliminary results on exploring the task of performing turn-level data augmentation for dialogue system based on different types of commonsense relationships, and the automatic evaluation of the generated synthetic turns. The proposed methodology takes advantage of the extended knowledge and zero-shot capabilities of pretrained Large Language Models (LLMs) to follow instructions, understand contextual information, and their commonsense reasoning capabilities. The approach draws inspiration from methodologies like Chain-of-Thought (CoT), applied more explicitly to the task of prompt-based generation for dialogue-based data augmentation conditioned on commonsense attributes, and the automatic evaluation of the generated dialogues. To assess the effectiveness of the proposed approach, first we extracted 200 randomly selected partial dialogues, from 5 different well-known dialogue datasets, and generate alternative responses conditioned on different event commonsense attributes. This novel dataset allows us to measure the proficiency of LLMs in generating contextually relevant commonsense knowledge, particularly up to 12 different specific ATOMIC [10] database relations. Secondly, we propose an evaluation framework to automatically detect the quality of the generated dataset inspired by the ACCENT [26] metric, which offers a nuanced approach to assess event commonsense. However, our method does not follow ACCENT's complex eventrelation tuple extraction process. Instead, we propose an instruction-based prompt for each commonsense attribute and use state-of-the-art LLMs to automatically detect the original attributes used when creating each augmented turn in the previous step. Preliminary results suggest that our approach effectively harnesses LLMs capabilities for commonsense reasoning and evaluation in dialogue systems.
Via
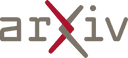
Jun 17, 2025
Abstract:One-shot subset selection serves as an effective tool to reduce deep learning training costs by identifying an informative data subset based on the information extracted by an information extractor (IE). Traditional IEs, typically pre-trained on the target dataset, are inherently dataset-dependent. Foundation models (FMs) offer a promising alternative, potentially mitigating this limitation. This work investigates two key questions: (1) Can FM-based subset selection outperform traditional IE-based methods across diverse datasets? (2) Do all FMs perform equally well as IEs for subset selection? Extensive experiments uncovered surprising insights: FMs consistently outperform traditional IEs on fine-grained datasets, whereas their advantage diminishes on coarse-grained datasets with noisy labels. Motivated by these finding, we propose RAM-APL (RAnking Mean-Accuracy of Pseudo-class Labels), a method tailored for fine-grained image datasets. RAM-APL leverages multiple FMs to enhance subset selection by exploiting their complementary strengths. Our approach achieves state-of-the-art performance on fine-grained datasets, including Oxford-IIIT Pet, Food-101, and Caltech-UCSD Birds-200-2011.
* 18 pages, 10 figures, accepted by ICML 2025
Via
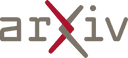
Jun 15, 2025
Abstract:The advent of 6G networks demands unprecedented levels of intelligence, adaptability, and efficiency to address challenges such as ultra-high-speed data transmission, ultra-low latency, and massive connectivity in dynamic environments. Traditional wireless image transmission frameworks, reliant on static configurations and isolated source-channel coding, struggle to balance computational efficiency, robustness, and quality under fluctuating channel conditions. To bridge this gap, this paper proposes an AI-native deep joint source-channel coding (JSCC) framework tailored for resource-constrained 6G networks. Our approach integrates key information extraction and adaptive background synthesis to enable intelligent, semantic-aware transmission. Leveraging AI-driven tools, Mediapipe for human pose detection and Rembg for background removal, the model dynamically isolates foreground features and matches backgrounds from a pre-trained library, reducing data payloads while preserving visual fidelity. Experimental results demonstrate significant improvements in peak signal-to-noise ratio (PSNR) compared with traditional JSCC method, especially under low-SNR conditions. This approach offers a practical solution for multimedia services in resource-constrained mobile communications.
Via
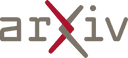
Jun 16, 2025
Abstract:With the advent of digital transformation, organisations are increasingly generating large volumes of data through the execution of various processes across disparate systems. By integrating data from these heterogeneous sources, it becomes possible to derive new insights essential for tasks such as monitoring and analysing process performance. Typically, this information is extracted during a data pre-processing or engineering phase. However, this step is often performed in an ad-hoc manner and is time-consuming and labour-intensive. To streamline this process, we introduce a reference model and a collection of patterns designed to enrich production event data. The reference model provides a standard way for storing and extracting production event data. The patterns describe common information extraction tasks and how such tasks can be automated effectively. The reference model is developed by combining the ISA-95 industry standard with the Event Knowledge Graph formalism. The patterns are developed based on empirical observations from event data sets originating in manufacturing processes and are formalised using the reference model. We evaluate the relevance and applicability of these patterns by demonstrating their application to use cases.
* Extended version of the paper submitted to EDOC 2025
Via
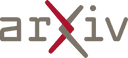
Jun 17, 2025
Abstract:Large Language Models (LLMs) are experiencing rapid advancements in complex reasoning, exhibiting remarkable generalization in mathematics and programming. In contrast, while spatial intelligence is fundamental for Vision-Language Models (VLMs) in real-world interaction, the systematic evaluation of their complex reasoning ability within spatial contexts remains underexplored. To bridge this gap, we introduce SIRI-Bench, a benchmark designed to evaluate VLMs' spatial intelligence through video-based reasoning tasks. SIRI-Bench comprises nearly 1K video-question-answer triplets, where each problem is embedded in a realistic 3D scene and captured by video. By carefully designing questions and corresponding 3D scenes, our benchmark ensures that solving the questions requires both spatial comprehension for extracting information and high-level reasoning for deriving solutions, making it a challenging benchmark for evaluating VLMs. To facilitate large-scale data synthesis, we develop an Automatic Scene Creation Engine. This engine, leveraging multiple specialized LLM agents, can generate realistic 3D scenes from abstract math problems, ensuring faithfulness to the original descriptions. Experimental results reveal that state-of-the-art VLMs struggle significantly on SIRI-Bench, underscoring the challenge of spatial reasoning. We hope that our study will bring researchers' attention to spatially grounded reasoning and advance VLMs in visual problem-solving.
* 16 pages, 9 figures
Via
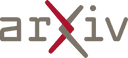
Jun 18, 2025
Abstract:In the ever-expanding domain of 5G-NR wireless cellular networks, over-the-air jamming attacks are prevalent as security attacks, compromising the quality of the received signal. We simulate a jamming environment by incorporating additive white Gaussian noise (AWGN) into the real-world In-phase and Quadrature (I/Q) OFDM datasets. A Convolutional Autoencoder (CAE) is exploited to implement a jamming detection over various characteristics such as heterogenous I/Q datasets; extracting relevant information on Synchronization Signal Blocks (SSBs), and fewer SSB observations with notable class imbalance. Given the characteristics of datasets, balanced datasets are acquired by employing a Conv1D conditional Wasserstein Generative Adversarial Network-Gradient Penalty(CWGAN-GP) on both majority and minority SSB observations. Additionally, we compare the performance and detection ability of the proposed CAE model on augmented datasets with benchmark models: Convolutional Denoising Autoencoder (CDAE) and Convolutional Sparse Autoencoder (CSAE). Despite the complexity of data heterogeneity involved across all datasets, CAE depicts the robustness in detection performance of jammed signal by achieving average values of 97.33% precision, 91.33% recall, 94.08% F1-score, and 94.35% accuracy over CDAE and CSAE.
* 6 pages, 5 figures, Accepted to IEEE International Symposium on
Personal, Indoor and Mobile Radio Communications (PIMRC) 2025
Via
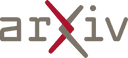
Jun 16, 2025
Abstract:Visual Information Extraction (VIE) converts unstructured document images into structured formats like JSON, critical for medical applications such as report analysis and online consultations. Traditional methods rely on OCR and language models, while end-to-end multimodal models offer direct JSON generation. However, domain-specific schemas and high annotation costs limit their effectiveness in medical VIE. We base our approach on the Reinforcement Learning with Verifiable Rewards (RLVR) framework to address these challenges using only 100 annotated samples. Our approach ensures dataset diversity, a balanced precision-recall reward mechanism to reduce hallucinations and improve field coverage, and innovative sampling strategies to enhance reasoning capabilities. Fine-tuning Qwen2.5-VL-7B with our RLVR method, we achieve state-of-the-art performance on medical VIE tasks, significantly improving F1, precision, and recall. While our models excel on tasks similar to medical datasets, performance drops on dissimilar tasks, highlighting the need for domain-specific optimization. Case studies further demonstrate the value of reasoning during training and inference for VIE.
Via
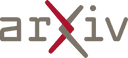
Jun 17, 2025
Abstract:This paper introduces ORD-CC32 , an open research dataset derived from the 1932 Cairo Congress of Arab Music recordings, a historically significant collection representing diverse Arab musical traditions. The dataset includes structured metadata, melodic and rhythmic mode tags (maqam and iqa), manually labeled tonic information, and acoustic features extracted using state-of-the-art pitch detection methods. These resources support computational studies of tuning, temperament, and regional variations in Arab music. A case study using pitch histograms demonstrates the potential for data-driven analysis of microtonal differences across regions. By making this dataset openly available, we aim to enable interdisciplinary research in computational ethnomusicology, music information retrieval (MIR), cultural studies, and digital heritage preservation. ORD-CC32 is shared on Zenodo with tools for feature extraction and metadata retrieval.
* 14 pages, 4 figures, 4 tables
Via
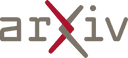
Jun 17, 2025
Abstract:Depth estimation is crucial for intelligent systems, enabling applications from autonomous navigation to augmented reality. While traditional stereo and active depth sensors have limitations in cost, power, and robustness, dual-pixel (DP) technology, ubiquitous in modern cameras, offers a compelling alternative. This paper introduces DiFuse-Net, a novel modality decoupled network design for disentangled RGB and DP based depth estimation. DiFuse-Net features a window bi-directional parallax attention mechanism (WBiPAM) specifically designed to capture the subtle DP disparity cues unique to smartphone cameras with small aperture. A separate encoder extracts contextual information from the RGB image, and these features are fused to enhance depth prediction. We also propose a Cross-modal Transfer Learning (CmTL) mechanism to utilize large-scale RGB-D datasets in the literature to cope with the limitations of obtaining large-scale RGB-DP-D dataset. Our evaluation and comparison of the proposed method demonstrates its superiority over the DP and stereo-based baseline methods. Additionally, we contribute a new, high-quality, real-world RGB-DP-D training dataset, named Dual-Camera Dual-Pixel (DCDP) dataset, created using our novel symmetric stereo camera hardware setup, stereo calibration and rectification protocol, and AI stereo disparity estimation method.
* Accepted in IROS 2025
Via
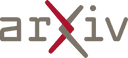
Jun 17, 2025
Abstract:In recirculating aquaculture systems, accurate and effective assessment of fish feeding intensity is crucial for reducing feed costs and calculating optimal feeding times. However, current studies have limitations in modality selection, feature extraction and fusion, and co-inference for decision making, which restrict further improvement in the accuracy, applicability and reliability of multimodal fusion models. To address this problem, this study proposes a Multi-stage Augmented Multimodal Interaction Network (MAINet) for quantifying fish feeding intensity. Firstly, a general feature extraction framework is proposed to efficiently extract feature information from input image, audio and water wave datas. Second, an Auxiliary-modality Reinforcement Primary-modality Mechanism (ARPM) is designed for inter-modal interaction and generate enhanced features, which consists of a Channel Attention Fusion Network (CAFN) and a Dual-mode Attention Fusion Network (DAFN). Finally, an Evidence Reasoning (ER) rule is introduced to fuse the output results of each modality and make decisions, thereby completing the quantification of fish feeding intensity. The experimental results show that the constructed MAINet reaches 96.76%, 96.78%, 96.79% and 96.79% in accuracy, precision, recall and F1-Score respectively, and its performance is significantly higher than the comparison models. Compared with models that adopt single-modality, dual-modality fusion and different decision-making fusion methods, it also has obvious advantages. Meanwhile, the ablation experiments further verified the key role of the proposed improvement strategy in improving the robustness and feature utilization efficiency of model, which can effectively improve the accuracy of the quantitative results of fish feeding intensity.
Via
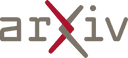