Topic:Information Extraction
What is Information Extraction? Information extraction is the process of automatically extracting structured information from unstructured text data.
Papers and Code
Jun 18, 2025
Abstract:Automatic lyrics transcription (ALT) remains a challenging task in the field of music information retrieval, despite great advances in automatic speech recognition (ASR) brought about by transformer-based architectures in recent years. One of the major challenges in ALT is the high amplitude of interfering audio signals relative to conventional ASR due to musical accompaniment. Recent advances in music source separation have enabled automatic extraction of high-quality separated vocals, which could potentially improve ALT performance. However, the effect of source separation has not been systematically investigated in order to establish best practices for its use. This work examines the impact of source separation on ALT using Whisper, a state-of-the-art open source ASR model. We evaluate Whisper's performance on original audio, separated vocals, and vocal stems across short-form and long-form transcription tasks. For short-form, we suggest a concatenation method that results in a consistent reduction in Word Error Rate (WER). For long-form, we propose an algorithm using source separation as a vocal activity detector to derive segment boundaries, which results in a consistent reduction in WER relative to Whisper's native long-form algorithm. Our approach achieves state-of-the-art results for an open source system on the Jam-ALT long-form ALT benchmark, without any training or fine-tuning. We also publish MUSDB-ALT, the first dataset of long-form lyric transcripts following the Jam-ALT guidelines for which vocal stems are publicly available.
* Accepted at 2025 ICME Workshop AI for Music
Via
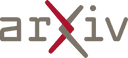
Jun 12, 2025
Abstract:The infrared and visible images fusion (IVIF) is receiving increasing attention from both the research community and industry due to its excellent results in downstream applications. Existing deep learning approaches often utilize convolutional neural networks to extract image features. However, the inherently capacity of convolution operations to capture global context can lead to information loss, thereby restricting fusion performance. To address this limitation, we propose an end-to-end fusion network named the Frequency-Spatial Attention Transformer Fusion Network (FSATFusion). The FSATFusion contains a frequency-spatial attention Transformer (FSAT) module designed to effectively capture discriminate features from source images. This FSAT module includes a frequency-spatial attention mechanism (FSAM) capable of extracting significant features from feature maps. Additionally, we propose an improved Transformer module (ITM) to enhance the ability to extract global context information of vanilla Transformer. We conducted both qualitative and quantitative comparative experiments, demonstrating the superior fusion quality and efficiency of FSATFusion compared to other state-of-the-art methods. Furthermore, our network was tested on two additional tasks without any modifications, to verify the excellent generalization capability of FSATFusion. Finally, the object detection experiment demonstrated the superiority of FSATFusion in downstream visual tasks. Our code is available at https://github.com/Lmmh058/FSATFusion.
Via
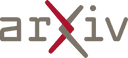
Jun 15, 2025
Abstract:Reconstructing a 3D point cloud from a given conditional sketch is challenging. Existing methods often work directly in 3D space, but domain variability and difficulty in reconstructing accurate 3D structures from 2D sketches remain significant obstacles. Moreover, ideal models should also accept prompts for control, in addition with the sparse sketch, posing challenges in multi-modal fusion. We propose DiffS-NOCS (Diffusion-based Sketch-to-NOCS Map), which leverages ControlNet with a modified multi-view decoder to generate NOCS maps with embedded 3D structure and position information in 2D space from sketches. The 3D point cloud is reconstructed by combining multiple NOCS maps from different views. To enhance sketch understanding, we integrate a viewpoint encoder for extracting viewpoint features. Additionally, we design a feature-level multi-view aggregation network as the denoising module, facilitating cross-view information exchange and improving 3D consistency in NOCS map generation. Experiments on ShapeNet demonstrate that DiffS-NOCS achieves controllable and fine-grained point cloud reconstruction aligned with sketches.
Via
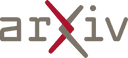
Jun 11, 2025
Abstract:Transparent and reflective objects in everyday environments pose significant challenges for depth sensors due to their unique visual properties, such as specular reflections and light transmission. These characteristics often lead to incomplete or inaccurate depth estimation, which severely impacts downstream geometry-based vision tasks, including object recognition, scene reconstruction, and robotic manipulation. To address the issue of missing depth information in transparent and reflective objects, we propose DCIRNet, a novel multimodal depth completion network that effectively integrates RGB images and depth maps to enhance depth estimation quality. Our approach incorporates an innovative multimodal feature fusion module designed to extract complementary information between RGB images and incomplete depth maps. Furthermore, we introduce a multi-stage supervision and depth refinement strategy that progressively improves depth completion and effectively mitigates the issue of blurred object boundaries. We integrate our depth completion model into dexterous grasping frameworks and achieve a $44\%$ improvement in the grasp success rate for transparent and reflective objects. We conduct extensive experiments on public datasets, where DCIRNet demonstrates superior performance. The experimental results validate the effectiveness of our approach and confirm its strong generalization capability across various transparent and reflective objects.
Via
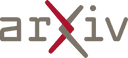
Jun 17, 2025
Abstract:Holographic displays have significant potential in virtual reality and augmented reality owing to their ability to provide all the depth cues. Deep learning-based methods play an important role in computer-generated holograms (CGH). During the diffraction process, each pixel exerts an influence on the reconstructed image. However, previous works face challenges in capturing sufficient information to accurately model this process, primarily due to the inadequacy of their effective receptive field (ERF). Here, we designed complex-valued deformable convolution for integration into network, enabling dynamic adjustment of the convolution kernel's shape to increase flexibility of ERF for better feature extraction. This approach allows us to utilize a single model while achieving state-of-the-art performance in both simulated and optical experiment reconstructions, surpassing existing open-source models. Specifically, our method has a peak signal-to-noise ratio that is 2.04 dB, 5.31 dB, and 9.71 dB higher than that of CCNN-CGH, HoloNet, and Holo-encoder, respectively, when the resolution is 1920$\times$1072. The number of parameters of our model is only about one-eighth of that of CCNN-CGH.
* 8 pages, 9 figures
Via
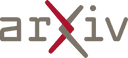
Jun 13, 2025
Abstract:Mitigating entity bias is a critical challenge in Relation Extraction (RE), where models often rely excessively on entities, resulting in poor generalization. This paper presents a novel approach to address this issue by adapting a Variational Information Bottleneck (VIB) framework. Our method compresses entity-specific information while preserving task-relevant features. It achieves state-of-the-art performance on relation extraction datasets across general, financial, and biomedical domains, in both indomain (original test sets) and out-of-domain (modified test sets with type-constrained entity replacements) settings. Our approach offers a robust, interpretable, and theoretically grounded methodology.
* Accepted at ACL 2025 Main
Via
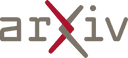
Jun 15, 2025
Abstract:The newly established IEEE 802.11bf Task Group aims to amend the WLAN standard to support advanced sensing applications such as human activity recognition (HAR). Although studies have demonstrated the potential of sub-7 GHz Wi-Fi Channel State Information (CSI) for HAR, no method currently performs reliably in real-world scenarios. This work tackles the poor generalization of Wi-Fi-based HAR by introducing an innovative approach to extracting and utilizing movement-related representations, which makes it robust to noise and static environmental properties. This is achieved by transforming CSI signals into the delay profile space and decomposing them into various Doppler velocities, which serve as informative projections of a mobile point's velocity from different unknown random angles. To mitigate the impact of this randomness, MORIC is introduced as a novel time series classification model based on random convolutional kernels, designed to be invariant to the random order and repetition of input representations, thereby enabling robust Wi-Fi CSI-based activity classification. Experimental results on the collected dataset demonstrate that the proposed method outperforms state-of-the-art approaches in terms of generalization accuracy for hand motion recognition, particularly for challenging gestures. Furthermore, incorporating a small number of calibration samples leads to a significant improvement in accuracy, enhancing the practicality of the method for real-world deployment.
Via
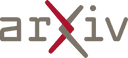
Jun 10, 2025
Abstract:Extracting meaningful latent representations from high-dimensional sequential data is a crucial challenge in machine learning, with applications spanning natural science and engineering. We introduce InfoDPCCA, a dynamic probabilistic Canonical Correlation Analysis (CCA) framework designed to model two interdependent sequences of observations. InfoDPCCA leverages a novel information-theoretic objective to extract a shared latent representation that captures the mutual structure between the data streams and balances representation compression and predictive sufficiency while also learning separate latent components that encode information specific to each sequence. Unlike prior dynamic CCA models, such as DPCCA, our approach explicitly enforces the shared latent space to encode only the mutual information between the sequences, improving interpretability and robustness. We further introduce a two-step training scheme to bridge the gap between information-theoretic representation learning and generative modeling, along with a residual connection mechanism to enhance training stability. Through experiments on synthetic and medical fMRI data, we demonstrate that InfoDPCCA excels as a tool for representation learning. Code of InfoDPCCA is available at https://github.com/marcusstang/InfoDPCCA.
* accepted by UAI-25, code is available at
\url{https://github.com/marcusstang/InfoDPCCA}
Via
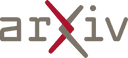
Jun 07, 2025
Abstract:Audio bandwidth extension is the task of reconstructing missing high frequency components of bandwidth-limited audio signals, where bandwidth limitation is a common issue for audio signals due to several reasons, including channel capacity and data constraints. While conventional spectral band replication is a well-established parametric approach to audio bandwidth extension, the SBR usually entails coarse feature extraction and reconstruction techniques, which leads to limitations when processing various types of audio signals. In parallel, numerous deep neural network-based audio bandwidth extension methods have been proposed. These DNN-based methods are usually referred to as blind BWE, as these methods do not rely on prior information extracted from original signals, and only utilize given low frequency band signals to estimate missing high frequency components. In order to replace conventional SBR with DNNs, simply adopting existing DNN-based methodologies results in suboptimal performance due to the blindness of these methods. My proposed research suggests a new approach to parametric non-blind bandwidth extension, as DNN-based side information extraction and DNN-based bandwidth extension are performed only at the front and end of the audio coding pipeline.
* Accepted to Interspeech 2025
Via
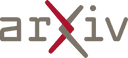
Jun 17, 2025
Abstract:The success of large language models in text processing has inspired their adaptation to speech modeling. However, since speech is continuous and complex, it is often discretized for autoregressive modeling. Speech tokens derived from self-supervised models (known as semantic tokens) typically focus on the linguistic aspects of speech but neglect prosodic information. As a result, models trained on these tokens can generate speech with reduced naturalness. Existing approaches try to fix this by adding pitch features to the semantic tokens. However, pitch alone cannot fully represent the range of paralinguistic attributes, and selecting the right features requires careful hand-engineering. To overcome this, we propose an end-to-end variational approach that automatically learns to encode these continuous speech attributes to enhance the semantic tokens. Our approach eliminates the need for manual extraction and selection of paralinguistic features. Moreover, it produces preferred speech continuations according to human raters. Code, samples and models are available at https://github.com/b04901014/vae-gslm.
* International Conference on Machine Learning (ICML) 2025
Via
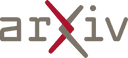