Topic:Information Extraction
What is Information Extraction? Information extraction is the process of automatically extracting structured information from unstructured text data.
Papers and Code
Apr 24, 2025
Abstract:Mutual Reinforcement Effect (MRE) is an emerging subfield at the intersection of information extraction and model interpretability. MRE aims to leverage the mutual understanding between tasks of different granularities, enhancing the performance of both coarse-grained and fine-grained tasks through joint modeling. While MRE has been explored and validated in the textual domain, its applicability to visual and multimodal domains remains unexplored. In this work, we extend MRE to the multimodal information extraction domain for the first time. Specifically, we introduce a new task: Multimodal Mutual Reinforcement Effect (M-MRE), and construct a corresponding dataset to support this task. To address the challenges posed by M-MRE, we further propose a Prompt Format Adapter (PFA) that is fully compatible with various Large Vision-Language Models (LVLMs). Experimental results demonstrate that MRE can also be observed in the M-MRE task, a multimodal text-image understanding scenario. This provides strong evidence that MRE facilitates mutual gains across three interrelated tasks, confirming its generalizability beyond the textual domain.
Via
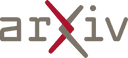
Apr 24, 2025
Abstract:Multimodal click-through rate (CTR) prediction is a key technique in industrial recommender systems. It leverages heterogeneous modalities such as text, images, and behavioral logs to capture high-order feature interactions between users and items, thereby enhancing the system's understanding of user interests and its ability to predict click behavior. The primary challenge in this field lies in effectively utilizing the rich semantic information from multiple modalities while satisfying the low-latency requirements of online inference in real-world applications. To foster progress in this area, the Multimodal CTR Prediction Challenge Track of the WWW 2025 EReL@MIR Workshop formulates the problem into two tasks: (1) Task 1 of Multimodal Item Embedding: this task aims to explore multimodal information extraction and item representation learning methods that enhance recommendation tasks; and (2) Task 2 of Multimodal CTR Prediction: this task aims to explore what multimodal recommendation model can effectively leverage multimodal embedding features and achieve better performance. In this paper, we propose a novel model for Task 2, named Quadratic Interest Network (QIN) for Multimodal CTR Prediction. Specifically, QIN employs adaptive sparse target attention to extract multimodal user behavior features, and leverages Quadratic Neural Networks to capture high-order feature interactions. As a result, QIN achieved an AUC of 0.9798 on the leaderboard and ranked second in the competition. The model code, training logs, hyperparameter configurations, and checkpoints are available at https://github.com/salmon1802/QIN.
Via
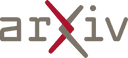
Apr 24, 2025
Abstract:Even in the era of rapid advances in large models, video understanding, particularly long videos, remains highly challenging. Compared with textual or image-based information, videos commonly contain more information with redundancy, requiring large models to strategically allocate attention at a global level for accurate comprehension. To address this, we propose MCAF, an agent-based, training-free framework perform video understanding through Multimodal Coarse-to-fine Attention Focusing. The key innovation lies in its ability to sense and prioritize segments of the video that are highly relevant to the understanding task. First, MCAF hierarchically concentrates on highly relevant frames through multimodal information, enhancing the correlation between the acquired contextual information and the query. Second, it employs a dilated temporal expansion mechanism to mitigate the risk of missing crucial details when extracting information from these concentrated frames. In addition, our framework incorporates a self-reflection mechanism utilizing the confidence level of the model's responses as feedback. By iteratively applying these two creative focusing strategies, it adaptively adjusts attention to capture highly query-connected context and thus improves response accuracy. MCAF outperforms comparable state-of-the-art methods on average. On the EgoSchema dataset, it achieves a remarkable 5% performance gain over the leading approach. Meanwhile, on Next-QA and IntentQA datasets, it outperforms the current state-of-the-art standard by 0.2% and 0.3% respectively. On the Video-MME dataset, which features videos averaging nearly an hour in length, MCAF also outperforms other agent-based methods.
Via
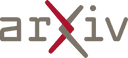
Apr 23, 2025
Abstract:IFC data has become the general building information standard for collaborative work in the construction industry. However, IFC data can be very complicated because it allows for multiple ways to represent the same product information. In this research, we utilise the capabilities of LLMs to parse the IFC data with Graph Retrieval-Augmented Generation (Graph-RAG) technique to retrieve building object properties and their relations. We will show that, despite limitations due to the complex hierarchy of the IFC data, the Graph-RAG parsing enhances generative LLMs like GPT-4o with graph-based knowledge, enabling natural language query-response retrieval without the need for a complex pipeline.
* 2025 European Conference on Computing in Construction
Via
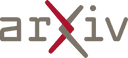
Apr 24, 2025
Abstract:Understanding source code is a topic of great interest in the software engineering community, since it can help programmers in various tasks such as software maintenance and reuse. Recent advances in large language models (LLMs) have demonstrated remarkable program comprehension capabilities, while transformer-based topic modeling techniques offer effective ways to extract semantic information from text. This paper proposes and explores a novel approach that combines these strengths to automatically identify meaningful topics in a corpus of Python programs. Our method consists in applying topic modeling on the descriptions obtained by asking an LLM to summarize the code. To assess the internal consistency of the extracted topics, we compare them against topics inferred from function names alone, and those derived from existing docstrings. Experimental results suggest that leveraging LLM-generated summaries provides interpretable and semantically rich representation of code structure. The promising results suggest that our approach can be fruitfully applied in various software engineering tasks such as automatic documentation and tagging, code search, software reorganization and knowledge discovery in large repositories.
Via
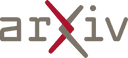
Apr 23, 2025
Abstract:Medical consultation dialogues contain critical clinical information, yet their unstructured nature hinders effective utilization in diagnosis and treatment. Traditional methods, relying on rule-based or shallow machine learning techniques, struggle to capture deep and implicit semantics. Recently, large pre-trained language models and Low-Rank Adaptation (LoRA), a lightweight fine-tuning method, have shown promise for structured information extraction. We propose EMRModel, a novel approach that integrates LoRA-based fine-tuning with code-style prompt design, aiming to efficiently convert medical consultation dialogues into structured electronic medical records (EMRs). Additionally, we construct a high-quality, realistically grounded dataset of medical consultation dialogues with detailed annotations. Furthermore, we introduce a fine-grained evaluation benchmark for medical consultation information extraction and provide a systematic evaluation methodology, advancing the optimization of medical natural language processing (NLP) models. Experimental results show EMRModel achieves an F1 score of 88.1%, improving by49.5% over standard pre-trained models. Compared to traditional LoRA fine-tuning methods, our model shows superior performance, highlighting its effectiveness in structured medical record extraction tasks.
Via
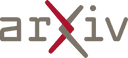
Apr 23, 2025
Abstract:This paper uses the capabilities of latent diffusion models (LDMs) to generate realistic RGB human-object interaction scenes to guide humanoid loco-manipulation planning. To do so, we extract from the generated images both the contact locations and robot configurations that are then used inside a whole-body trajectory optimization (TO) formulation to generate physically consistent trajectories for humanoids. We validate our full pipeline in simulation for different long-horizon loco-manipulation scenarios and perform an extensive analysis of the proposed contact and robot configuration extraction pipeline. Our results show that using the information extracted from LDMs, we can generate physically consistent trajectories that require long-horizon reasoning.
Via
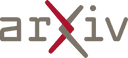
Apr 24, 2025
Abstract:We propose a fully unsupervised algorithm that detects from encephalography (EEG) recordings when a subject actively listens to sound, versus when the sound is ignored. This problem is known as absolute auditory attention decoding (aAAD). We propose an unsupervised discriminative CCA model for feature extraction and combine it with an unsupervised classifier called minimally informed linear discriminant analysis (MILDA) for aAAD classification. Remarkably, the proposed unsupervised algorithm performs significantly better than a state-of-the-art supervised model. A key reason is that the unsupervised algorithm can successfully adapt to the non-stationary test data at a low computational cost. This opens the door to the analysis of the auditory attention of a subject using EEG signals with a model that automatically tunes itself to the subject without requiring an arduous supervised training session beforehand.
Via
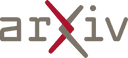
Apr 23, 2025
Abstract:Verifiers are auxiliary models that assess the correctness of outputs generated by base large language models (LLMs). They play a crucial role in many strategies for solving reasoning-intensive problems with LLMs. Typically, verifiers are LLMs themselves, often as large (or larger) than the base model they support, making them computationally expensive. In this work, we introduce a novel lightweight verification approach, LiLaVe, which reliably extracts correctness signals from the hidden states of the base LLM. A key advantage of LiLaVe is its ability to operate with only a small fraction of the computational budget required by traditional LLM-based verifiers. To demonstrate its practicality, we couple LiLaVe with popular meta-generation strategies, like best-of-n or self-consistency. Moreover, we design novel LiLaVe-based approaches, like conditional self-correction or conditional majority voting, that significantly improve both accuracy and efficiency in generation tasks with smaller LLMs. Our work demonstrates the fruitfulness of extracting latent information from the hidden states of LLMs, and opens the door to scalable and resource-efficient solutions for reasoning-intensive applications.
Via
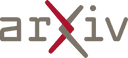
Apr 23, 2025
Abstract:Unstructured clinical data can serve as a unique and rich source of information that can meaningfully inform clinical practice. Extracting the most pertinent context from such data is critical for exploiting its true potential toward optimal and timely decision-making in patient care. While prior research has explored various methods for clinical text summarization, most prior studies either process all input tokens uniformly or rely on heuristic-based filters, which can overlook nuanced clinical cues and fail to prioritize information critical for decision-making. In this study, we propose Contextual, a novel framework that integrates a Context-Preserving Token Filtering method with a Domain-Specific Knowledge Graph (KG) for contextual augmentation. By preserving context-specific important tokens and enriching them with structured knowledge, ConTextual improves both linguistic coherence and clinical fidelity. Our extensive empirical evaluations on two public benchmark datasets demonstrate that ConTextual consistently outperforms other baselines. Our proposed approach highlights the complementary role of token-level filtering and structured retrieval in enhancing both linguistic and clinical integrity, as well as offering a scalable solution for improving precision in clinical text generation.
Via
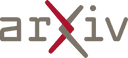