Topic:Human Object Interaction Detection
What is Human Object Interaction Detection? Human-object interaction (HOI) detection is a task of identifying a set of interactions in an image, which involves the localization of the subject (i.e., humans) and target (i.e., objects) of interaction, and the classification of the interaction labels.
Papers and Code
Apr 24, 2025
Abstract:We present a framework for perspective-aware reasoning in vision-language models (VLMs) through mental imagery simulation. Perspective-taking, the ability to perceive an environment or situation from an alternative viewpoint, is a key benchmark for human-level visual understanding, essential for environmental interaction and collaboration with autonomous agents. Despite advancements in spatial reasoning within VLMs, recent research has shown that modern VLMs significantly lack perspective-aware reasoning capabilities and exhibit a strong bias toward egocentric interpretations. To bridge the gap between VLMs and human perception, we focus on the role of mental imagery, where humans perceive the world through abstracted representations that facilitate perspective shifts. Motivated by this, we propose a framework for perspective-aware reasoning, named Abstract Perspective Change (APC), that effectively leverages vision foundation models, such as object detection, segmentation, and orientation estimation, to construct scene abstractions and enable perspective transformations. Our experiments on synthetic and real-image benchmarks, compared with various VLMs, demonstrate significant improvements in perspective-aware reasoning with our framework, further outperforming fine-tuned spatial reasoning models and novel-view-synthesis-based approaches.
Via
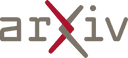
Apr 16, 2025
Abstract:Visual relation detection (VRD) aims to identify relationships (or interactions) between object pairs in an image. Although recent VRD models have achieved impressive performance, they are all restricted to pre-defined relation categories, while failing to consider the semantic ambiguity characteristic of visual relations. Unlike objects, the appearance of visual relations is always subtle and can be described by multiple predicate words from different perspectives, e.g., ``ride'' can be depicted as ``race'' and ``sit on'', from the sports and spatial position views, respectively. To this end, we propose to model visual relations as continuous embeddings, and design diffusion models to achieve generalized VRD in a conditional generative manner, termed Diff-VRD. We model the diffusion process in a latent space and generate all possible relations in the image as an embedding sequence. During the generation, the visual and text embeddings of subject-object pairs serve as conditional signals and are injected via cross-attention. After the generation, we design a subsequent matching stage to assign the relation words to subject-object pairs by considering their semantic similarities. Benefiting from the diffusion-based generative process, our Diff-VRD is able to generate visual relations beyond the pre-defined category labels of datasets. To properly evaluate this generalized VRD task, we introduce two evaluation metrics, i.e., text-to-image retrieval and SPICE PR Curve inspired by image captioning. Extensive experiments in both human-object interaction (HOI) detection and scene graph generation (SGG) benchmarks attest to the superiority and effectiveness of Diff-VRD.
* Under review at IEEE TCSVT. The Appendix is provided additionally
Via
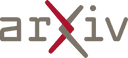
Apr 07, 2025
Abstract:With robots becoming increasingly prevalent in various domains, it has become crucial to equip them with tools to achieve greater fluency in interactions with humans. One of the promising areas for further exploration lies in human trust. A real-time, objective model of human trust could be used to maximize productivity, preserve safety, and mitigate failure. In this work, we attempt to use physiological measures, gaze, and facial expressions to model human trust in a robot partner. We are the first to design an in-person, human-robot supervisory interaction study to create a dedicated trust dataset. Using this dataset, we train machine learning algorithms to identify the objective measures that are most indicative of trust in a robot partner, advancing trust prediction in human-robot interactions. Our findings indicate that a combination of sensor modalities (blood volume pulse, electrodermal activity, skin temperature, and gaze) can enhance the accuracy of detecting human trust in a robot partner. Furthermore, the Extra Trees, Random Forest, and Decision Trees classifiers exhibit consistently better performance in measuring the person's trust in the robot partner. These results lay the groundwork for constructing a real-time trust model for human-robot interaction, which could foster more efficient interactions between humans and robots.
* IEEE International Conference on Robotics and Automation (ICRA),
2025
* Accepted at the IEEE International Conference on Robotics and
Automation (ICRA), 2025
Via
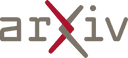
Mar 28, 2025
Abstract:Force estimation in human-object interactions is crucial for various fields like ergonomics, physical therapy, and sports science. Traditional methods depend on specialized equipment such as force plates and sensors, which makes accurate assessments both expensive and restricted to laboratory settings. In this paper, we introduce ForcePose, a novel deep learning framework that estimates applied forces by combining human pose estimation with object detection. Our approach leverages MediaPipe for skeletal tracking and SSD MobileNet for object recognition to create a unified representation of human-object interaction. We've developed a specialized neural network that processes both spatial and temporal features to predict force magnitude and direction without needing any physical sensors. After training on our dataset of 850 annotated videos with corresponding force measurements, our model achieves a mean absolute error of 5.83 N in force magnitude and 7.4 degrees in force direction. When compared to existing computer vision approaches, our method performs 27.5% better while still offering real-time performance on standard computing hardware. ForcePose opens up new possibilities for force analysis in diverse real-world scenarios where traditional measurement tools are impractical or intrusive. This paper discusses our methodology, the dataset creation process, evaluation metrics, and potential applications across rehabilitation, ergonomics assessment, and athletic performance analysis.
Via
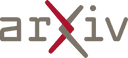
Apr 01, 2025
Abstract:Mobile robots increasingly operate alongside humans but are often out of sight, so that humans need to rely on the sounds of the robots to recognize their presence. For successful human-robot interaction (HRI), it is therefore crucial to understand how humans perceive robots by their consequential sounds, i.e., operating noise. Prior research suggests that the sound of a quadruped Go1 is more detectable than that of a wheeled Turtlebot. This study builds on this and examines the human ability to localize consequential sounds of three robots (quadruped Go1, wheeled Turtlebot 2i, wheeled HSR) in Virtual Reality. In a within-subjects design, we assessed participants' localization performance for the robots with and without an acoustic vehicle alerting system (AVAS) for two velocities (0.3, 0.8 m/s) and two trajectories (head-on, radial). In each trial, participants were presented with the sound of a moving robot for 3~s and were tasked to point at its final position (localization task). Localization errors were measured as the absolute angular difference between the participants' estimated and the actual robot position. Results showed that the robot type significantly influenced the localization accuracy and precision, with the sound of the wheeled HSR (especially without AVAS) performing worst under all experimental conditions. Surprisingly, participants rated the HSR sound as more positive, less annoying, and more trustworthy than the Turtlebot and Go1 sound. This reveals a tension between subjective evaluation and objective auditory localization performance. Our findings highlight consequential robot sounds as a critical factor for designing intuitive and effective HRI, with implications for human-centered robot design and social navigation.
Via
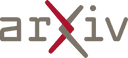
Mar 13, 2025
Abstract:In the domain of Image Anomaly Detection (IAD), Existing methods frequently exhibit a paucity of fine-grained, interpretable semantic information, resulting in the detection of anomalous entities or activities that are susceptible to machine illusions. This deficiency often leads to the detection of anomalous entities or actions that are susceptible to machine illusions and lack sufficient explanation. In this thesis, we propose a novel approach to anomaly detection, termed Hoi2Anomaly, which aims to achieve precise discrimination and localization of anomalies. The proposed methodology involves the construction of a multi-modal instruction tuning dataset comprising human-object interaction (HOI) pairs in anomalous scenarios. Second, we have trained an HOI extractor in threat scenarios to localize and match anomalous actions and entities. Finally, explanatory content is generated for the detected anomalous HOI by fine-tuning the visual language pretraining (VLP) framework. The experimental results demonstrate that Hoi2Anomaly surpasses existing generative approaches in terms of precision and explainability. We will release Hoi2Anomaly for the advancement of the field of anomaly detection.
Via
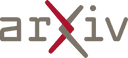
Mar 20, 2025
Abstract:Robotics has shown significant potential in assisting people with disabilities to enhance their independence and involvement in daily activities. Indeed, a societal long-term impact is expected in home-care assistance with the deployment of intelligent robotic interfaces. This work presents a human-robot interface developed to help people with upper limbs impairments, such as those affected by stroke injuries, in activities of everyday life. The proposed interface leverages on a visual servoing guidance component, which utilizes an inexpensive but effective laser emitter device. By projecting the laser on a surface within the workspace of the robot, the user is able to guide the robotic manipulator to desired locations, to reach, grasp and manipulate objects. Considering the targeted users, the laser emitter is worn on the head, enabling to intuitively control the robot motions with head movements that point the laser in the environment, which projection is detected with a neural network based perception module. The interface implements two control modalities: the first allows the user to select specific locations directly, commanding the robot to reach those points; the second employs a paper keyboard with buttons that can be virtually pressed by pointing the laser at them. These buttons enable a more direct control of the Cartesian velocity of the end-effector and provides additional functionalities such as commanding the action of the gripper. The proposed interface is evaluated in a series of manipulation tasks involving a 6DOF assistive robot manipulator equipped with 1DOF beak-like gripper. The two interface modalities are combined to successfully accomplish tasks requiring bimanual capacity that is usually affected in people with upper limbs impairments.
* IEEE Robotics and Automation Letters, vol. 9, no. 9, pp.
7653-7660, Sept. 2024
* 8 pages, 12 figures
Via
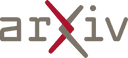
Mar 06, 2025
Abstract:Humans detect real-world object anomalies by perceiving, interacting, and reasoning based on object-conditioned physical knowledge. The long-term goal of Industrial Anomaly Detection (IAD) is to enable machines to autonomously replicate this skill. However, current IAD algorithms are largely developed and tested on static, semantically simple datasets, which diverge from real-world scenarios where physical understanding and reasoning are essential. To bridge this gap, we introduce the Physics Anomaly Detection (Phys-AD) dataset, the first large-scale, real-world, physics-grounded video dataset for industrial anomaly detection. Collected using a real robot arm and motor, Phys-AD provides a diverse set of dynamic, semantically rich scenarios. The dataset includes more than 6400 videos across 22 real-world object categories, interacting with robot arms and motors, and exhibits 47 types of anomalies. Anomaly detection in Phys-AD requires visual reasoning, combining both physical knowledge and video content to determine object abnormality. We benchmark state-of-the-art anomaly detection methods under three settings: unsupervised AD, weakly-supervised AD, and video-understanding AD, highlighting their limitations in handling physics-grounded anomalies. Additionally, we introduce the Physics Anomaly Explanation (PAEval) metric, designed to assess the ability of visual-language foundation models to not only detect anomalies but also provide accurate explanations for their underlying physical causes. Our dataset and benchmark will be publicly available.
* Accepted by CVPR 2025
Via
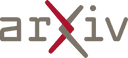
Mar 04, 2025
Abstract:In this paper, we present a touch technology to achieve tactile interactivity for human-robot interaction (HRI) in soft robotics. By combining a capacitive touch sensor with an online solid mechanics simulation provided by the SOFA framework, contact detection is achieved for arbitrary shapes. Furthermore, the implementation of the capacitive touch technology presented here is selectively sensitive to human touch (conductive objects), while it is largely unaffected by the deformations created by the pneumatic actuation of our soft robot. Multi-touch interactions are also possible. We evaluated our approach with an organic soft robotics sculpture that was created by a visual artist. In particular, we evaluate that the touch localization capabilities are robust under the deformation of the device. We discuss the potential this approach has for the arts and entertainment as well as other domains.
* IEEE Robotics and Automation Letters, 2025
* 8 pages, 17 figures. Accepted at IEEE Robotics and Automation Letters
(RA-L) 2025
Via
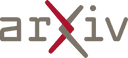
Mar 11, 2025
Abstract:Tactile sensors can significantly enhance the perception of humanoid robotics systems by providing contact information that facilitates human-like interactions. However, existing commercial tactile sensors focus on improving the resolution and sensitivity of single-modal detection with high-cost components and densely integrated design, incurring complex manufacturing processes and unaffordable prices. In this work, we present Bio-Skin, a cost-effective multi-modal tactile sensor that utilizes single-axis Hall-effect sensors for planar normal force measurement and bar-shape piezo resistors for 2D shear force measurement. A thermistor coupling with a heating wire is integrated into a silicone body to achieve temperature sensation and thermostatic function analogous to human skin. We also present a cross-reference framework to validate the two modalities of the force sensing signal, improving the sensing fidelity in a complex electromagnetic environment. Bio-Skin has a multi-layer design, and each layer is manufactured sequentially and subsequently integrated, thereby offering a fast production pathway. After calibration, Bio-Skin demonstrates performance metrics-including signal-to-range ratio, sampling rate, and measurement range-comparable to current commercial products, with one-tenth of the cost. The sensor's real-world performance is evaluated using an Allegro hand in object grasping tasks, while its temperature regulation functionality was assessed in a material detection task.
* This work has been submitted to the IEEE for possible publication
Via
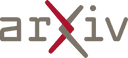