Topic:Heart Rate Variability
What is Heart Rate Variability? Heart rate variability is the variation in time intervals between heartbeats, which is an important indicator of cardiovascular health.
Papers and Code
May 09, 2025
Abstract:Wearable sensors are widely used to collect physiological data and develop stress detection models. However, most studies focus on a single dataset, rarely evaluating model reproducibility across devices, populations, or study conditions. We previously assessed the reproducibility of stress detection models across multiple studies, testing models trained on one dataset against others using heart rate (with R-R interval) and electrodermal activity (EDA). In this study, we extended our stress detection reproducibility to consumer wearable sensors. We compared validated research-grade devices, to consumer wearables - Biopac MP160, Polar H10, Empatica E4, to the Garmin Forerunner 55s, assessing device-specific stress detection performance by conducting a new stress study on undergraduate students. Thirty-five students completed three standardized stress-induction tasks in a lab setting. Biopac MP160 performed the best, being consistent with our expectations of it as the gold standard, though performance varied across devices and models. Combining heart rate variability (HRV) and EDA enhanced stress prediction across most scenarios. However, Empatica E4 showed variability; while HRV and EDA improved stress detection in leave-one-subject-out (LOSO) evaluations (AUROC up to 0.953), device-specific limitations led to underperformance when tested with our pre-trained stress detection tool (AUROC 0.723), highlighting generalizability challenges related to hardware-model compatibility. Garmin Forerunner 55s demonstrated strong potential for real-world stress monitoring, achieving the best mental arithmetic stress detection performance in LOSO (AUROC up to 0.961) comparable to research-grade devices like Polar H10 (AUROC 0.954), and Empatica E4 (AUROC 0.905 with HRV-only model and AUROC 0.953 with HRV+EDA model), with the added advantage of consumer-friendly wearability for free-living contexts.
* Accepted at IEEE EMBC 2025
Via
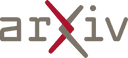
May 07, 2025
Abstract:Human interaction experience plays a crucial role in the effectiveness of human-machine collaboration, especially as interactions in future systems progress towards tighter physical and functional integration. While automation design has been shown to impact task performance, its influence on human experience metrics such as flow, sense of agency (SoA), and embodiment remains underexplored. This study investigates how variations in automation design affect these psychological experience measures and examines correlations between subjective experience and physiological indicators. A user study was conducted in a simulated wood workshop, where participants collaborated with a lightweight robot under four automation levels. The results of the study indicate that medium automation levels enhance flow, SoA and embodiment, striking a balance between support and user autonomy. In contrast, higher automation, despite optimizing task performance, diminishes perceived flow and agency. Furthermore, we observed that grip force might be considered as a real-time proxy of SoA, while correlations with heart rate variability were inconclusive. The findings underscore the necessity for automation strategies that integrate human- centric metrics, aiming to optimize both performance and user experience in collaborative robotic systems
Via
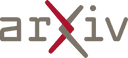
May 05, 2025
Abstract:Sepsis is a life threatening medical condition that occurs when the body has an extreme response to infection, leading to widespread inflammation, organ failure, and potentially death. Because sepsis can worsen rapidly, early detection is critical to saving lives. However, current diagnostic methods often identify sepsis only after significant damage has already occurred. Our project aims to address this challenge by developing a machine learning based system to predict sepsis in its early stages, giving healthcare providers more time to intervene. A major problem with existing models is the wide variability in the patient information or features they use, such as heart rate, temperature, and lab results. This inconsistency makes models difficult to compare and limits their ability to work across different hospitals and settings. To solve this, we propose a method called Feature Aligned Transfer Learning (FATL), which identifies and focuses on the most important and commonly reported features across multiple studies, ensuring the model remains consistent and clinically relevant. Most existing models are trained on narrow patient groups, leading to population bias. FATL addresses this by combining knowledge from models trained on diverse populations, using a weighted approach that reflects each models contribution. This makes the system more generalizable and effective across different patient demographics and clinical environments. FATL offers a practical and scalable solution for early sepsis detection, particularly in hospitals with limited resources, and has the potential to improve patient outcomes, reduce healthcare costs, and support more equitable healthcare delivery.
* A project implemented for MACHINE LEARNING IN HEALTH AND BIOMEDICAL
SCIENCE
Via
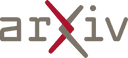
May 03, 2025
Abstract:Biosignal monitoring, in particular heart activity through heart rate (HR) and heart rate variability (HRV) tracking, is vital in enabling continuous, non-invasive tracking of physiological and cognitive states. Recent studies have explored compact, head-worn devices for HR and HRV monitoring to improve usability and reduce stigma. However, this approach is challenged by the current reliance on wet electrodes, which limits usability, the weakness of ear-derived signals, making HR/HRV extraction more complex, and the incompatibility of current algorithms for embedded deployment. This work introduces a single-ear wearable system for real-time ECG (Electrocardiogram) parameter estimation, which directly runs on BioGAP, an energy-efficient device for biosignal acquisition and processing. By combining SoA in-ear electrode technology, an optimized DeepMF algorithm, and BioGAP, our proposed subject-independent approach allows for robust extraction of HR/HRV parameters directly on the device with just 36.7 uJ/inference at comparable performance with respect to the current state-of-the-art architecture, achieving 0.49 bpm and 25.82 ms for HR/HRV mean errors, respectively and an estimated battery life of 36h with a total system power consumption of 7.6 mW. Clinical relevance: The ability to reconstruct ECG signals and extract HR and HRV paves the way for continuous, unobtrusive cardiovascular monitoring with head-worn devices. In particular, the integration of cardiovascular measurements in everyday-use devices (such as earbuds) has potential in continuous at-home monitoring to enable early detection of cardiovascular irregularities.
* 7 pages, 7 figures, to be published in IEEE EMBC conference
Via
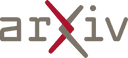
Apr 03, 2025
Abstract:Photoplethysmography (PPG) is a widely used non-invasive technique for monitoring cardiovascular health and various physiological parameters on consumer and medical devices. While motion artifacts are well-known challenges in dynamic settings, suboptimal skin-sensor contact in sedentary conditions - a critical issue often overlooked in existing literature - can distort PPG signal morphology, leading to the loss or shift of essential waveform features and therefore degrading sensing performance. In this work, we propose CP-PPG, a novel approach that transforms Contact Pressure-distorted PPG signals into ones with the ideal morphology. CP-PPG incorporates a novel data collection approach, a well-crafted signal processing pipeline, and an advanced deep adversarial model trained with a custom PPG-aware loss function. We validated CP-PPG through comprehensive evaluations, including 1) morphology transformation performance on our self-collected dataset, 2) downstream physiological monitoring performance on public datasets, and 3) in-the-wild performance. Extensive experiments demonstrate substantial and consistent improvements in signal fidelity (Mean Absolute Error: 0.09, 40% improvement over the original signal) as well as downstream performance across all evaluations in Heart Rate (HR), Heart Rate Variability (HRV), Respiration Rate (RR), and Blood Pressure (BP) estimation (on average, 21% improvement in HR; 41-46% in HRV; 6% in RR; and 4-5% in BP). These findings highlight the critical importance of addressing skin-sensor contact issues for accurate and dependable PPG-based physiological monitoring. Furthermore, CP-PPG can serve as a generic, plug-in API to enhance PPG signal quality.
Via
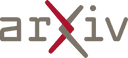
Apr 01, 2025
Abstract:Remote photoplethysmography (rPPG) offers a novel approach to noninvasive monitoring of vital signs, such as respiratory rate, utilizing a camera. Although several supervised and self-supervised methods have been proposed, they often fail to accurately reconstruct the PPG signal, particularly in distinguishing between systolic and diastolic components. Their primary focus tends to be solely on extracting heart rate, which may not accurately represent the complete PPG signal. To address this limitation, this paper proposes a novel deep learning architecture using Generative Adversarial Networks by introducing multi-discriminators to extract rPPG signals from facial videos. These discriminators focus on the time domain, the frequency domain, and the second derivative of the original time domain signal. The discriminator integrates four loss functions: variance loss to mitigate local minima caused by noise; dynamic time warping loss to address local minima induced by alignment and sequences of variable lengths; Sparsity Loss for heart rate adjustment, and Variance Loss to ensure a uniform distribution across the desired frequency domain and time interval between systolic and diastolic phases of the PPG signal.
Via
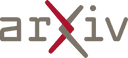
Mar 21, 2025
Abstract:Remote estimation of vital signs enables health monitoring for situations in which contact-based devices are either not available, too intrusive, or too expensive. In this paper, we present a modular, interpretable pipeline for pulse signal estimation from video of the face that achieves state-of-the-art results on publicly available datasets.Our imaging photoplethysmography (iPPG) system consists of three modules: face and landmark detection, time-series extraction, and pulse signal/pulse rate estimation. Unlike many deep learning methods that make use of a single black-box model that maps directly from input video to output signal or heart rate, our modular approach enables each of the three parts of the pipeline to be interpreted individually. The pulse signal estimation module, which we call TURNIP (Time-Series U-Net with Recurrence for Noise-Robust Imaging Photoplethysmography), allows the system to faithfully reconstruct the underlying pulse signal waveform and uses it to measure heart rate and pulse rate variability metrics, even in the presence of motion. When parts of the face are occluded due to extreme head poses, our system explicitly detects such "self-occluded" regions and maintains estimation robustness despite the missing information. Our algorithm provides reliable heart rate estimates without the need for specialized sensors or contact with the skin, outperforming previous iPPG methods on both color (RGB) and near-infrared (NIR) datasets.
* 14 Pages, 8 figures
Via
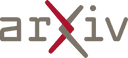
Mar 13, 2025
Abstract:Photoplethysmography (PPG) sensors have been widely used in consumer wearable devices to monitor heart rates (HR) and heart rate variability (HRV). Despite the prevalence, PPG signals can be contaminated by motion artifacts induced from daily activities. Existing approaches mainly use the amplitude information to perform PPG peak detection. However, these approaches cannot accurately identify peaks, since motion artifacts may bring random and significant amplitude variations. To improve the performance of PPG peak detection, the time information can be used. Specifically, heart rates exhibit temporal consistency that consecutive heartbeat intervals in a normal person can have limited variations. To leverage the temporal consistency, we propose the Temporal Attentive U-Net, i.e., TAU, to accurately detect peaks from PPG signals. In TAU, we design a time module that encodes temporal consistency in temporal embeddings. We integrate the amplitude information with temporal embeddings using the attention mechanism to estimate peak labels. Our experimental results show that TAU outperforms eleven baselines on heart rate estimation by more than 22.4%. Our TAU model achieves the best performance across various Signal-to-Noise Ratio (SNR) levels. Moreover, we achieve Pearson correlation coefficients higher than 0.9 (p < 0.01) on estimating HRV features from low-noise-level PPG signals.
* 27 pages, submitted to a journal
Via
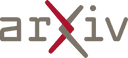
Feb 20, 2025
Abstract:Electrocardiograms (ECGs) are essential for monitoring cardiac health, allowing clinicians to analyze heart rate variability (HRV), detect abnormal rhythms, and diagnose cardiovascular diseases. However, ECG signals, especially those from wearable devices, are often affected by noise artifacts caused by motion, muscle activity, or device-related interference. These artifacts distort R-peaks and the characteristic QRS complex, making HRV analysis unreliable and increasing the risk of misdiagnosis. Despite this, the few existing studies on ECG noise detection have primarily focused on a single dataset, limiting the understanding of how well noise detection models generalize across different datasets. In this paper, we investigate the generalizability of noise detection in ECG using a novel HRV-based approach through cross-dataset experiments on four datasets. Our results show that machine learning achieves an average accuracy of over 90\% and an AUPRC of more than 0.9. These findings suggest that regardless of the ECG data source or the type of noise, the proposed method maintains high accuracy even on unseen datasets, demonstrating the feasibility of generalizability.
Via
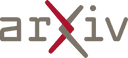
Feb 11, 2025
Abstract:Heart rate variability (HRV) is widely recognized as a valuable biomarker for assessing autonomic cardiac regulation. Pulse rate variability (PRV) is a common surrogate of HRV given the wide usability of PPG in commercially available devices. However, there is no clear conclusion on whether PRV can replace HRV given their different physiological mechanisms. This study evaluates the interchangeability of young adults HRV and PRV during supine-to-stand (STS) tests which are known as common posture transitions in daily life monitoring. Fifteen features from time, frequency and nonlinear domains were extracted from both electrocardiography and PPG signals. Paired t-tests and Wilcoxon signed-rank tests examined the difference between the extracted HRV and PRV features during supine, transition and standing phases separately. One feature showed significant difference in the supine phase, and this discrepancy increased to four in the transition and standing phases. These findings suggested that PRV is different from HRV in the STS tests, despite the fact that both metrics can reflect the sympathetic activation triggered by the posture changes.
Via
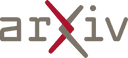