May 15, 2025
Abstract:With the growing demand for healthy foods, agricultural product price forecasting has become increasingly important. Hass avocados, as a high-value crop, exhibit complex price fluctuations influenced by factors such as seasonality, region, and weather. Traditional prediction models often struggle with highly nonlinear and dynamic data. To address this, we propose a hybrid deep learning model, TCN-MLP-Attention Architecture, combining Temporal Convolutional Networks (TCN) for sequential feature extraction, Multi-Layer Perceptrons (MLP) for nonlinear interactions, and an Attention mechanism for dynamic feature weighting. The dataset used covers over 50,000 records of Hass avocado sales across the U.S. from 2015 to 2018, including variables such as sales volume, average price, time, region, weather, and variety type, collected from point-of-sale systems and the Hass Avocado Board. After systematic preprocessing, including missing value imputation and feature normalization, the proposed model was trained and evaluated. Experimental results demonstrate that the TCN-MLP-Attention model achieves excellent predictive performance, with an RMSE of 1.23 and an MSE of 1.51, outperforming traditional methods. This research provides a scalable and effective approach for time series forecasting in agricultural markets and offers valuable insights for intelligent supply chain management and price strategy optimization.
Via
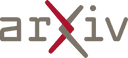