May 24, 2025
Abstract:To capture individual gait patterns, excluding identity-irrelevant cues in walking videos, such as clothing texture and color, remains a persistent challenge for vision-based gait recognition. Traditional silhouette- and pose-based methods, though theoretically effective at removing such distractions, often fall short of high accuracy due to their sparse and less informative inputs. Emerging end-to-end methods address this by directly denoising RGB videos using human priors. Building on this trend, we propose DenoisingGait, a novel gait denoising method. Inspired by the philosophy that "what I cannot create, I do not understand", we turn to generative diffusion models, uncovering how they partially filter out irrelevant factors for gait understanding. Additionally, we introduce a geometry-driven Feature Matching module, which, combined with background removal via human silhouettes, condenses the multi-channel diffusion features at each foreground pixel into a two-channel direction vector. Specifically, the proposed within- and cross-frame matching respectively capture the local vectorized structures of gait appearance and motion, producing a novel flow-like gait representation termed Gait Feature Field, which further reduces residual noise in diffusion features. Experiments on the CCPG, CASIA-B*, and SUSTech1K datasets demonstrate that DenoisingGait achieves a new SoTA performance in most cases for both within- and cross-domain evaluations. Code is available at https://github.com/ShiqiYu/OpenGait.
* 8pages, 4 figures
Via
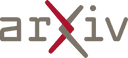