What is facial recognition? Facial recognition is an AI-based technique for identifying or confirming an individual's identity using their face. It maps facial features from an image or video and then compares the information with a collection of known faces to find a match.
Papers and Code
Mar 05, 2025
Abstract:Manual attendance tracking at large-scale events, such as marriage functions or conferences, is often inefficient and prone to human error. To address this challenge, we propose an automated, cloud-based attendance tracking system that uses cameras mounted at the entrance and exit gates. The mounted cameras continuously capture video and send the video data to cloud services to perform real-time face detection and recognition. Unlike existing solutions, our system accurately identifies attendees even when they are not looking directly at the camera, allowing natural movements, such as looking around or talking while walking. To the best of our knowledge, this is the first system to achieve high recognition rates under such dynamic conditions. Our system demonstrates overall 90% accuracy, with each video frame processed in 5 seconds, ensuring real time operation without frame loss. In addition, notifications are sent promptly to security personnel within the same latency. This system achieves 100% accuracy for individuals without facial obstructions and successfully recognizes all attendees appearing within the camera's field of view, providing a robust solution for attendee recognition in large-scale social events.
Via
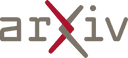
Mar 08, 2025
Abstract:In Neural Networks, there are various methods of feature fusion. Different strategies can significantly affect the effectiveness of feature representation, consequently influencing the ability of model to extract representative and discriminative features. In the field of face recognition, traditional feature fusion methods include feature concatenation and feature addition. Recently, various attention mechanism-based fusion strategies have emerged. However, we found that these methods primarily focus on the important features in the image, referred to as salient features in this paper, while neglecting another equally important set of features for image recognition tasks, which we term differential features. This may cause the model to overlook critical local differences when dealing with complex facial samples. Therefore, in this paper, we propose an efficient convolution module called MSConv (Multiplicative and Subtractive Convolution), designed to balance the learning of model about salient and differential features. Specifically, we employ multi-scale mixed convolution to capture both local and broader contextual information from face images, and then utilize Multiplication Operation (MO) and Subtraction Operation (SO) to extract salient and differential features, respectively. Experimental results demonstrate that by integrating both salient and differential features, MSConv outperforms models that only focus on salient features.
Via
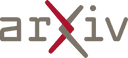
Jan 14, 2025
Abstract:Facial expressions play a crucial role in human communication serving as a powerful and impactful means to express a wide range of emotions. With advancements in artificial intelligence and computer vision, deep neural networks have emerged as effective tools for facial emotion recognition. In this paper, we propose EmoNeXt, a novel deep learning framework for facial expression recognition based on an adapted ConvNeXt architecture network. We integrate a Spatial Transformer Network (STN) to focus on feature-rich regions of the face and Squeeze-and-Excitation blocks to capture channel-wise dependencies. Moreover, we introduce a self-attention regularization term, encouraging the model to generate compact feature vectors. We demonstrate the superiority of our model over existing state-of-the-art deep learning models on the FER2013 dataset regarding emotion classification accuracy.
* 6 pages, 5 figures and 2 tables. 2023 IEEE 25th International
Workshop on Multimedia Signal Processing (MMSP), Poitiers, France
Via
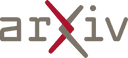
Feb 28, 2025
Abstract:In the age of AI-driven generative technologies, traditional biometric recognition systems face unprecedented challenges, particularly from sophisticated deepfake and face reenactment techniques. In this study, we propose a Two-Stream Spatial-Temporal Transformer Framework for person identification using upper body keypoints visible during online conversations, which we term conversational keypoints. Our framework processes both spatial relationships between keypoints and their temporal evolution through two specialized branches: a Spatial Transformer (STR) that learns distinctive structural patterns in keypoint configurations, and a Temporal Transformer (TTR) that captures sequential motion patterns. Using the state-of-the-art Sapiens pose estimator, we extract 133 keypoints (based on COCO-WholeBody format) representing facial features, head pose, and hand positions. The framework was evaluated on a dataset of 114 individuals engaged in natural conversations, achieving recognition accuracies of 80.12% for the spatial stream, 63.61% for the temporal stream. We then explored two fusion strategies: a shared loss function approach achieving 82.22% accuracy, and a feature-level fusion method that concatenates feature maps from both streams, significantly improving performance to 94.86%. By jointly modeling both static anatomical relationships and dynamic movement patterns, our approach learns comprehensive identity signatures that are more robust to spoofing than traditional appearance-based methods.
Via
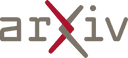
Jan 11, 2025
Abstract:The widespread adoption of facial recognition (FR) models raises serious concerns about their potential misuse, motivating the development of anti-facial recognition (AFR) to protect user facial privacy. In this paper, we argue that the static FR strategy, predominantly adopted in prior literature for evaluating AFR efficacy, cannot faithfully characterize the actual capabilities of determined trackers who aim to track a specific target identity. In particular, we introduce \emph{\ourAttack}, a dynamic FR strategy where the model's gallery database is iteratively updated with newly recognized target identity images. Surprisingly, such a simple approach renders all the existing AFR protections ineffective. To mitigate the privacy threats posed by DynTracker, we advocate for explicitly promoting diversity in the AFR-protected images. We hypothesize that the lack of diversity is the primary cause of the failure of existing AFR methods. Specifically, we develop \emph{DivTrackee}, a novel method for crafting diverse AFR protections that builds upon a text-guided image generation framework and diversity-promoting adversarial losses. Through comprehensive experiments on various facial image benchmarks and feature extractors, we demonstrate DynTracker's strength in breaking existing AFR methods and the superiority of DivTrackee in preventing user facial images from being identified by dynamic FR strategies. We believe our work can act as an important initial step towards developing more effective AFR methods for protecting user facial privacy against determined trackers.
Via
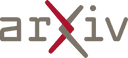
Feb 27, 2025
Abstract:In this paper, a novel dataset is introduced, designed to assess student attention within in-person classroom settings. This dataset encompasses RGB camera data, featuring multiple cameras per student to capture both posture and facial expressions, in addition to smartwatch sensor data for each individual. This dataset allows machine learning algorithms to be trained to predict attention and correlate it with emotion. A comprehensive suite of attention and emotion labels for each student is provided, generated through self-reporting as well as evaluations by four different experts. Our dataset uniquely combines facial and environmental camera data, smartwatch metrics, and includes underrepresented ethnicities in similar datasets, all within in-the-wild, in-person settings, making it the most comprehensive dataset of its kind currently available. The dataset presented offers an extensive and diverse collection of data pertaining to student interactions across different educational contexts, augmented with additional metadata from other tools. This initiative addresses existing deficiencies by offering a valuable resource for the analysis of student attention and emotion in face-to-face lessons.
Via
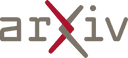
Jan 30, 2025
Abstract:Under Display Camera (UDC) is an advanced imaging system that places a digital camera lens underneath a display panel, effectively concealing the camera. However, the display panel significantly degrades captured images or videos, introducing low transmittance, blur, noise, and flare issues. Tackling such issues is challenging because of the complex degradation of UDCs, including diverse flare patterns. Despite extensive research on UDC images and their restoration models, studies on videos have yet to be significantly explored. While two UDC video datasets exist, they primarily focus on unrealistic or synthetic UDC degradation rather than real-world UDC degradation. In this paper, we propose a real-world UDC video dataset called UDC-VIT. Unlike existing datasets, only UDC-VIT exclusively includes human motions that target facial recognition. We propose a video-capturing system to simultaneously acquire non-degraded and UDC-degraded videos of the same scene. Then, we align a pair of captured videos frame by frame, using discrete Fourier transform (DFT). We compare UDC-VIT with six representative UDC still image datasets and two existing UDC video datasets. Using six deep-learning models, we compare UDC-VIT and an existing synthetic UDC video dataset. The results indicate the ineffectiveness of models trained on earlier synthetic UDC video datasets, as they do not reflect the actual characteristics of UDC-degraded videos. We also demonstrate the importance of effective UDC restoration by evaluating face recognition accuracy concerning PSNR, SSIM, and LPIPS scores. UDC-VIT enables further exploration in the UDC video restoration and offers better insights into the challenge. UDC-VIT is available at our project site.
* Main body (10 pages, 9 Figures, 3 Tables), References (4 pages),
Appendix (15 pages, 11 Figures, 6 Tables)
Via
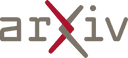
Jan 15, 2025
Abstract:Facial brightness is a key image quality factor impacting face recognition accuracy differentials across demographic groups. In this work, we aim to decrease the accuracy gap between the similarity score distributions for Caucasian and African American female mated image pairs, as measured by d' between distributions. To balance brightness across demographic groups, we conduct three experiments, interpreting brightness in the face skin region either as median pixel value or as the distribution of pixel values. Balancing based on median brightness alone yields up to a 46.8% decrease in d', while balancing based on brightness distribution yields up to a 57.6% decrease. In all three cases, the similarity scores of the individual distributions improve, with mean scores maximally improving 5.9% for Caucasian females and 3.7% for African American females.
* 14 pages, 11 figures, Conference submission
Via
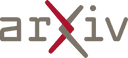
Jan 25, 2025
Abstract:In human-centric scenes, the ability to simultaneously understand visual and auditory information is crucial. While recent omni models can process multiple modalities, they generally lack effectiveness in human-centric scenes due to the absence of large-scale, specialized datasets and non-targeted architectures. In this work, we developed HumanOmni, the industry's first human-centric Omni-multimodal large language model. We constructed a dataset containing over 2.4 million human-centric video clips with detailed captions and more than 14 million instructions, facilitating the understanding of diverse human-centric scenes. HumanOmni includes three specialized branches for understanding different types of scenes. It adaptively fuses features from these branches based on user instructions, significantly enhancing visual understanding in scenes centered around individuals. Moreover, HumanOmni integrates audio features to ensure a comprehensive understanding of environments and individuals. Our experiments validate HumanOmni's advanced capabilities in handling human-centric scenes across a variety of tasks, including emotion recognition, facial expression description, and action understanding. Our model will be open-sourced to facilitate further development and collaboration within both academia and industry.
Via
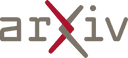
Jan 30, 2025
Abstract:Facial Emotion Recognition has emerged as increasingly pivotal in the domain of User Experience, notably within modern usability testing, as it facilitates a deeper comprehension of user satisfaction and engagement. This study aims to extend the ResEmoteNet model by employing a knowledge distillation framework to develop Mini-ResEmoteNet models - lightweight student models - tailored for usability testing. Experiments were conducted on the FER2013 and RAF-DB datasets to assess the efficacy of three student model architectures: Student Model A, Student Model B, and Student Model C. Their development involves reducing the number of feature channels in each layer of the teacher model by approximately 50%, 75%, and 87.5%. Demonstrating exceptional performance on the FER2013 dataset, Student Model A (E1) achieved a test accuracy of 76.33%, marking a 0.21% absolute improvement over EmoNeXt. Moreover, the results exhibit absolute improvements in terms of inference speed and memory usage during inference compared to the ResEmoteNet model. The findings indicate that the proposed methods surpass other state-of-the-art approaches.
* 5 pages with 4 figures
Via
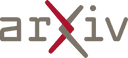