What is facial recognition? Facial recognition is an AI-based technique for identifying or confirming an individual's identity using their face. It maps facial features from an image or video and then compares the information with a collection of known faces to find a match.
Papers and Code
Jan 16, 2025
Abstract:Understanding emotions accurately is essential for fields like human-computer interaction. Due to the complexity of emotions and their multi-modal nature (e.g., emotions are influenced by facial expressions and audio), researchers have turned to using multi-modal models to understand human emotions rather than single-modality. However, current video multi-modal large language models (MLLMs) encounter difficulties in effectively integrating audio and identifying subtle facial micro-expressions. Furthermore, the lack of detailed emotion analysis datasets also limits the development of multimodal emotion analysis. To address these issues, we introduce a self-reviewed dataset and a human-reviewed dataset, comprising 24,137 coarse-grained samples and 3,500 manually annotated samples with detailed emotion annotations, respectively. These datasets allow models to learn from diverse scenarios and better generalize to real-world applications. Moreover, in addition to the audio modeling, we propose to explicitly integrate facial encoding models into the existing advanced Video MLLM, enabling the MLLM to effectively unify audio and the subtle facial cues for emotion understanding. By aligning these features within a unified space and employing instruction tuning in our proposed datasets, our Omni-Emotion achieves state-of-the-art performance in both emotion recognition and reasoning tasks.
Via
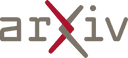
Dec 06, 2024
Abstract:The integration of conversational agents into our daily lives has become increasingly common, yet many of these agents cannot engage in deep interactions with humans. Despite this, there is a noticeable shortage of datasets that capture multimodal information from human-robot interaction dialogues. To address this gap, we have developed a Personal Emotional Robotic Conversational sYstem (PERCY) and recorded a novel multimodal dataset that encompasses rich embodied interaction data. The process involved asking participants to complete a questionnaire and gathering their profiles on ten topics, such as hobbies and favourite music. Subsequently, we initiated conversations between the robot and the participants, leveraging GPT-4 to generate contextually appropriate responses based on the participant's profile and emotional state, as determined by facial expression recognition and sentiment analysis. Automatic and user evaluations were conducted to assess the overall quality of the collected data. The results of both evaluations indicated a high level of naturalness, engagement, fluency, consistency, and relevance in the conversation, as well as the robot's ability to provide empathetic responses. It is worth noting that the dataset is derived from genuine interactions with the robot, involving participants who provided personal information and conveyed actual emotions.
* 9 pages, 5 Figures, Rejected from International Conference of Human
Robot Interaction 2025, Melbourne, Australia
Via
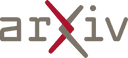
Nov 25, 2024
Abstract:Recent advancements in diffusion models have made generative image editing more accessible, enabling creative edits but raising ethical concerns, particularly regarding malicious edits to human portraits that threaten privacy and identity security. Existing protection methods primarily rely on adversarial perturbations to nullify edits but often fail against diverse editing requests. We propose FaceLock, a novel approach to portrait protection that optimizes adversarial perturbations to destroy or significantly alter biometric information, rendering edited outputs biometrically unrecognizable. FaceLock integrates facial recognition and visual perception into perturbation optimization to provide robust protection against various editing attempts. We also highlight flaws in commonly used evaluation metrics and reveal how they can be manipulated, emphasizing the need for reliable assessments of protection. Experiments show FaceLock outperforms baselines in defending against malicious edits and is robust against purification techniques. Ablation studies confirm its stability and broad applicability across diffusion-based editing algorithms. Our work advances biometric defense and sets the foundation for privacy-preserving practices in image editing. The code is available at: https://github.com/taco-group/FaceLock.
Via
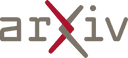
Jan 07, 2025
Abstract:The assessment of face image quality is crucial to ensure reliable face recognition. In order to provide data subjects and operators with explainable and actionable feedback regarding captured face images, relevant quality components have to be measured. Quality components that are known to negatively impact the utility of face images include JPEG and JPEG 2000 compression artefacts, among others. Compression can result in a loss of important image details which may impair the recognition performance. In this work, deep neural networks are trained to detect the compression artefacts in a face images. For this purpose, artefact-free facial images are compressed with the JPEG and JPEG 2000 compression algorithms. Subsequently, the PSNR and SSIM metrics are employed to obtain training labels based on which neural networks are trained using a single network to detect JPEG and JPEG 2000 artefacts, respectively. The evaluation of the proposed method shows promising results: in terms of detection accuracy, error rates of 2-3% are obtained for utilizing PSNR labels during training. In addition, we show that error rates of different open-source and commercial face recognition systems can be significantly reduced by discarding face images exhibiting severe compression artefacts. To minimize resource consumption, EfficientNetV2 serves as basis for the presented algorithm, which is available as part of the OFIQ software.
* 2nd Workshop on Fairness in Biometric Systems (FAIRBIO) at
International Conference on Pattern Recognition (ICPR) 2024
Via
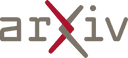
Nov 19, 2024
Abstract:Emotion Recognition (ER) is the process of identifying human emotions from given data. Currently, the field heavily relies on facial expression recognition (FER) because facial expressions contain rich emotional cues. However, it is important to note that facial expressions may not always precisely reflect genuine emotions and FER-based results may yield misleading ER. To understand and bridge this gap between FER and ER, we introduce eye behaviors as an important emotional cues for the creation of a new Eye-behavior-aided Multimodal Emotion Recognition (EMER) dataset. Different from existing multimodal ER datasets, the EMER dataset employs a stimulus material-induced spontaneous emotion generation method to integrate non-invasive eye behavior data, like eye movements and eye fixation maps, with facial videos, aiming to obtain natural and accurate human emotions. Notably, for the first time, we provide annotations for both ER and FER in the EMER, enabling a comprehensive analysis to better illustrate the gap between both tasks. Furthermore, we specifically design a new EMERT architecture to concurrently enhance performance in both ER and FER by efficiently identifying and bridging the emotion gap between the two.Specifically, our EMERT employs modality-adversarial feature decoupling and multi-task Transformer to augment the modeling of eye behaviors, thus providing an effective complement to facial expressions. In the experiment, we introduce seven multimodal benchmark protocols for a variety of comprehensive evaluations of the EMER dataset. The results show that the EMERT outperforms other state-of-the-art multimodal methods by a great margin, revealing the importance of modeling eye behaviors for robust ER. To sum up, we provide a comprehensive analysis of the importance of eye behaviors in ER, advancing the study on addressing the gap between FER and ER for more robust ER performance.
* The paper is part of ongoing work and we request to withdraw it from
arXiv to revise it further. And The paper was submitted without agreement
from all co-authors
Via
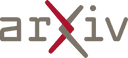
Nov 07, 2024
Abstract:In today's high-pressure and isolated society, the demand for emotional support has surged, necessitating innovative solutions. Socially Assistive Robots (SARs) offer a technological approach to providing emotional assistance by leveraging advanced robotics, artificial intelligence, and sensor technologies. This study explores the development of an emotional support robot designed to detect and respond to human emotions, particularly sadness, through facial recognition and gesture analysis. Utilising the Lego Mindstorms Robotic Kit, Raspberry Pi 4, and various Python libraries, the robot is capable of delivering empathetic interactions, including comforting hugs and AI-generated conversations. Experimental findings highlight the robot's effective facial recognition accuracy, user interaction, and hug feedback mechanisms. These results demonstrate the feasibility of using SARs for emotional support, showcasing their potential features and functions. This research underscores the promise of SARs in providing innovative emotional assistance and enhancing human-robot interaction.
* Part of proceedings of 6th International Conference AsiaHaptics 2024
Via
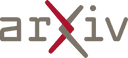
Oct 23, 2024
Abstract:In this paper, we study the effect of introducing channel and spatial attention mechanisms, namely SEN-Net, ECA-Net, and CBAM, to existing CNN vision-based models such as VGGNet, ResNet, and ResNetV2 to perform the Facial Emotion Recognition task. We show that not only attention can significantly improve the performance of these models but also that combining them with a different activation function can further help increase the performance of these models.
Via
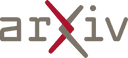
Nov 04, 2024
Abstract:On facial expression datasets with complex and numerous feature types, where the significance and dominance of labeled features are difficult to predict, facial expression recognition(FER) encounters the challenges of inter-class similarity and intra-class variances, making it difficult to mine effective features. We aim to solely leverage the feature similarity among facial samples to address this. We introduce the Cross Similarity Attention (CSA), an input-output position-sensitive attention mechanism that harnesses feature similarity across different images to compute the corresponding global spatial attention. Based on this, we propose a four-branch circular framework, called Quadruplet Cross Similarity (QCS), to extract discriminative features from the same class and eliminate redundant ones from different classes synchronously to refine cleaner features. The symmetry of the network ensures balanced and stable training and reduces the amount of CSA interaction matrix. Contrastive residual distillation is utilized to transfer the information learned in the cross module back to the base network. The cross-attention module exists during training, and only one base branch is retained during inference. our proposed QCS model outperforms state-of-the-art methods on several popular FER datasets, without requiring additional landmark information or other extra training data. The code is available at https://github.com/birdwcp/QCS.
Via
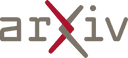
Nov 15, 2024
Abstract:Adversarial attacks in the physical world pose a significant threat to the security of vision-based systems, such as facial recognition and autonomous driving. Existing adversarial patch methods primarily focus on improving attack performance, but they often produce patches that are easily detectable by humans and struggle to achieve environmental consistency, i.e., blending patches into the environment. This paper introduces a novel approach for generating adversarial patches, which addresses both the visual naturalness and environmental consistency of the patches. We propose Prompt-Guided Environmentally Consistent Adversarial Patch (PG-ECAP), a method that aligns the patch with the environment to ensure seamless integration into the environment. The approach leverages diffusion models to generate patches that are both environmental consistency and effective in evading detection. To further enhance the naturalness and consistency, we introduce two alignment losses: Prompt Alignment Loss and Latent Space Alignment Loss, ensuring that the generated patch maintains its adversarial properties while fitting naturally within its environment. Extensive experiments in both digital and physical domains demonstrate that PG-ECAP outperforms existing methods in attack success rate and environmental consistency.
Via
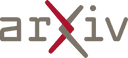
Nov 27, 2024
Abstract:With the advancement of face reconstruction (FR) systems, privacy-preserving face recognition (PPFR) has gained popularity for its secure face recognition, enhanced facial privacy protection, and robustness to various attacks. Besides, specific models and algorithms are proposed for face embedding protection by mapping embeddings to a secure space. However, there is a lack of studies on investigating and evaluating the possibility of extracting face images from embeddings of those systems, especially for PPFR. In this work, we introduce the first approach to exploit Kolmogorov-Arnold Network (KAN) for conducting embedding-to-face attacks against state-of-the-art (SOTA) FR and PPFR systems. Face embedding mapping (FEM) models are proposed to learn the distribution mapping relation between the embeddings from the initial domain and target domain. In comparison with Multi-Layer Perceptrons (MLP), we provide two variants, FEM-KAN and FEM-MLP, for efficient non-linear embedding-to-embedding mapping in order to reconstruct realistic face images from the corresponding face embedding. To verify our methods, we conduct extensive experiments with various PPFR and FR models. We also measure reconstructed face images with different metrics to evaluate the image quality. Through comprehensive experiments, we demonstrate the effectiveness of FEMs in accurate embedding mapping and face reconstruction.
* 16 pages, 8 figures
Via
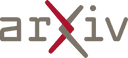