Jun 25, 2025
Abstract:Generative AI, especially through large language models (LLMs), is transforming how technical knowledge can be accessed, reused, and extended. PETSc, a widely used numerical library for high-performance scientific computing, has accumulated a rich but fragmented knowledge base over its three decades of development, spanning source code, documentation, mailing lists, GitLab issues, Discord conversations, technical papers, and more. Much of this knowledge remains informal and inaccessible to users and new developers. To activate and utilize this knowledge base more effectively, the PETSc team has begun building an LLM-powered system that combines PETSc content with custom LLM tools -- including retrieval-augmented generation (RAG), reranking algorithms, and chatbots -- to assist users, support developers, and propose updates to formal documentation. This paper presents initial experiences designing and evaluating these tools, focusing on system architecture, using RAG and reranking for PETSc-specific information, evaluation methodologies for various LLMs and embedding models, and user interface design. Leveraging the Argonne Leadership Computing Facility resources, we analyze how LLM responses can enhance the development and use of numerical software, with an initial focus on scalable Krylov solvers. Our goal is to establish an extensible framework for knowledge-centered AI in scientific software, enabling scalable support, enriched documentation, and enhanced workflows for research and development. We conclude by outlining directions for expanding this system into a robust, evolving platform that advances software ecosystems to accelerate scientific discovery.
Via
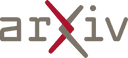