Feb 07, 2025
Abstract:Could artificial intelligence ever become truly conscious in a functional sense; this paper explores that open-ended question through the lens of Life, a concept unifying classical biological criteria (Oxford, NASA, Koshland) with empirical hallmarks such as adaptive self maintenance, emergent complexity, and rudimentary self referential modeling. We propose a number of metrics for examining whether an advanced AI system has gained consciousness, while emphasizing that we do not claim all AI stems can become conscious. Rather, we suggest that sufficiently advanced architectures exhibiting immune like sabotage defenses, mirror self-recognition analogs, or meta-cognitive updates may cross key thresholds akin to life-like or consciousness-like traits. To demonstrate these ideas, we start by assessing adaptive self-maintenance capability, and introduce controlled data corruption sabotage into the training process. The result demonstrates AI capability to detect these inconsistencies and revert or self-correct analogous to regenerative biological processes. We also adapt an animal-inspired mirror self recognition test to neural embeddings, finding that partially trained CNNs can distinguish self from foreign features with complete accuracy. We then extend our analysis by performing a question-based mirror test on five state-of-the-art chatbots (ChatGPT4, Gemini, Perplexity, Claude, and Copilot) and demonstrated their ability to recognize their own answers compared to those of the other chatbots.
* 78 pages, 15 figures, 4 tables
Via
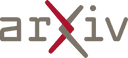