What is Topic Modeling? Topic modeling is a type of statistical modeling for discovering the abstract topics that occur in a collection of documents.
Papers and Code
May 20, 2025
Abstract:EasyMath is a compact benchmark for practical math reasoning in small language models. It covers thirteen categories, from basic arithmetic and order of operations to word problems, algebraic expressions, edge cases, and omits specialist topics. We tested 23 models (14M to 4B parameters) using exact, numerical, and symbolic checks on free-form answers in a zero-shot setting. Accuracy rises with size and training, chain-of-thought adds modest gains, and consistency improves at scale.
* 17 pages, 9 figures, 8 tables
Via
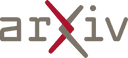
May 14, 2025
Abstract:Wildfires have become increasingly frequent, irregular, and severe in recent years. Understanding how affected populations perceive and respond during wildfire crises is critical for timely and empathetic disaster response. Social media platforms offer a crowd-sourced channel to capture evolving public discourse, providing hyperlocal information and insight into public sentiment. This study analyzes Reddit discourse during the 2025 Los Angeles wildfires, spanning from the onset of the disaster to full containment. We collect 385 posts and 114,879 comments related to the Palisades and Eaton fires. We adopt topic modeling methods to identify the latent topics, enhanced by large language models (LLMs) and human-in-the-loop (HITL) refinement. Furthermore, we develop a hierarchical framework to categorize latent topics, consisting of two main categories, Situational Awareness (SA) and Crisis Narratives (CN). The volume of SA category closely aligns with real-world fire progressions, peaking within the first 2-5 days as the fires reach the maximum extent. The most frequent co-occurring category set of public health and safety, loss and damage, and emergency resources expands on a wide range of health-related latent topics, including environmental health, occupational health, and one health. Grief signals and mental health risks consistently accounted for 60 percentage and 40 percentage of CN instances, respectively, with the highest total volume occurring at night. This study contributes the first annotated social media dataset on the 2025 LA fires, and introduces a scalable multi-layer framework that leverages topic modeling for crisis discourse analysis. By identifying persistent public health concerns, our results can inform more empathetic and adaptive strategies for disaster response, public health communication, and future research in comparable climate-related disaster events.
Via
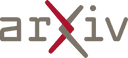
May 12, 2025
Abstract:Traditional topic models often struggle with contextual nuances and fail to adequately handle polysemy and rare words. This limitation typically results in topics that lack coherence and quality. Large Language Models (LLMs) can mitigate this issue by generating an initial set of topics. However, these raw topics frequently lack refinement and representativeness, which leads to redundancy without lexical similarity and reduced interpretability. This paper introduces HAMLET, a graph-driven architecture for cross-lingual healthcare topic modeling that uses LLMs. The proposed approach leverages neural-enhanced semantic fusion to refine the embeddings of topics generated by the LLM. Instead of relying solely on statistical co-occurrence or human interpretation to extract topics from a document corpus, this method introduces a topic embedding refinement that uses Bidirectional Encoder Representations from Transformers (BERT) and Graph Neural Networks (GNN). After topic generation, a hybrid technique that involves BERT and Sentence-BERT (SBERT) is employed for embedding. The topic representations are further refined using a GNN, which establishes connections between documents, topics, words, similar topics, and similar words. A novel method is introduced to compute similarities. Consequently, the topic embeddings are refined, and the top k topics are extracted. Experiments were conducted using two healthcare datasets, one in English and one in French, from which six sets were derived. The results demonstrate the effectiveness of HAMLET.
Via
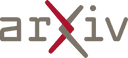
May 30, 2025
Abstract:Steering vectors are a lightweight method for controlling text properties by adding a learned bias to language model activations at inference time. So far, steering vectors have predominantly been evaluated in multiple-choice settings, while their effectiveness in free-form generation tasks remains understudied. Moving "Beyond Multiple Choice," we thoroughly evaluate the effectiveness of steering vectors in adaptively controlling topical focus, sentiment, toxicity, and readability in abstractive summaries of the NEWTS dataset. We find that steering effectively controls the targeted summary properties, but high steering strengths consistently degrade both intrinsic and extrinsic text quality. Compared to steering, prompting offers weaker control, while preserving text quality. Combining steering and prompting yields the strongest control over text properties and offers the most favorable efficacy-quality trade-off at moderate steering strengths. Our results underscore the practical trade-off between control strength and text quality preservation when applying steering vectors to free-form generation tasks.
* 29 pages, 21 figures, preprint
Via
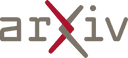
May 29, 2025
Abstract:The inherent nature of social media posts, characterized by the freedom of language use with a disjointed array of diverse opinions and topics, poses significant challenges to downstream NLP tasks such as comment clustering, comment summarization, and social media opinion analysis. To address this, we propose a granular level of identifying and generating aspect terms from individual comments to guide model attention. Specifically, we leverage multilingual large language models with supervised fine-tuning for comment aspect term generation (CAT-G), further aligning the model's predictions with human expectations through DPO. We demonstrate the effectiveness of our method in enhancing the comprehension of social media discourse on two NLP tasks. Moreover, this paper contributes the first multilingual CAT-G test set on English, Chinese, Malay, and Bahasa Indonesian. As LLM capabilities vary among languages, this test set allows for a comparative analysis of performance across languages with varying levels of LLM proficiency.
* The paper was peer-reviewed
Via
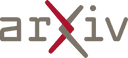
May 27, 2025
Abstract:Dialogue Topic Segmentation (DTS) aims to divide dialogues into coherent segments. DTS plays a crucial role in various NLP downstream tasks, but suffers from chronic problems: data shortage, labeling ambiguity, and incremental complexity of recently proposed solutions. On the other hand, Despite advances in Large Language Models (LLMs) and reasoning strategies, these have rarely been applied to DTS. This paper introduces Def-DTS: Deductive Reasoning for Open-domain Dialogue Topic Segmentation, which utilizes LLM-based multi-step deductive reasoning to enhance DTS performance and enable case study using intermediate result. Our method employs a structured prompting approach for bidirectional context summarization, utterance intent classification, and deductive topic shift detection. In the intent classification process, we propose the generalizable intent list for domain-agnostic dialogue intent classification. Experiments in various dialogue settings demonstrate that Def-DTS consistently outperforms traditional and state-of-the-art approaches, with each subtask contributing to improved performance, particularly in reducing type 2 error. We also explore the potential for autolabeling, emphasizing the importance of LLM reasoning techniques in DTS.
* 19 pages, 3 figures, Accepted to Findings of the ACL 2025
Via
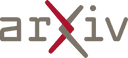
May 26, 2025
Abstract:We investigate long-context vulnerabilities in Large Language Models (LLMs) through Many-Shot Jailbreaking (MSJ). Our experiments utilize context length of up to 128K tokens. Through comprehensive analysis with various many-shot attack settings with different instruction styles, shot density, topic, and format, we reveal that context length is the primary factor determining attack effectiveness. Critically, we find that successful attacks do not require carefully crafted harmful content. Even repetitive shots or random dummy text can circumvent model safety measures, suggesting fundamental limitations in long-context processing capabilities of LLMs. The safety behavior of well-aligned models becomes increasingly inconsistent with longer contexts. These findings highlight significant safety gaps in context expansion capabilities of LLMs, emphasizing the need for new safety mechanisms.
* Accepted by ACL 2025
Via
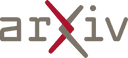
May 23, 2025
Abstract:Conversations are usually structured by roles -- who is speaking, who's being addressed, and who's listening -- and unfold in threads that break with changes in speaker floor or topical focus. While large language models (LLMs) have shown incredible capabilities in dialogue and reasoning, their ability to understand fine-grained conversational structure, especially in multi-modal, multi-party settings, remains underexplored. To address this gap, we introduce a suite of tasks focused on conversational role attribution (speaker, addressees, side-participants) and conversation threading (utterance linking and clustering), drawing on conversation analysis and sociolinguistics. To support those tasks, we present a human annotated dataset of 4,398 annotations for speakers and reply-to relationship, 5,755 addressees, and 3,142 side-participants. We evaluate popular audio-visual LLMs and vision-language models on our dataset, and our experimental results suggest that multimodal conversational structure understanding remains challenging. The most performant audio-visual LLM outperforms all vision-language models across all metrics, especially in speaker and addressee recognition. However, its performance drops significantly when conversation participants are anonymized. The number of conversation participants in a clip is the strongest negative predictor of role-attribution performance, while acoustic clarity (measured by pitch and spectral centroid) and detected face coverage yield positive associations. We hope this work lays the groundwork for future evaluation and development of multimodal LLMs that can reason more effectively about conversation structure.
Via
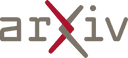
May 22, 2025
Abstract:Large Language Models (LLMs) are commonly pretrained on vast corpora of text without utilizing contextual metadata such as source, quality, or topic, leading to a context-free learning paradigm. While recent studies suggest that adding metadata like URL information as context (i.e., auxiliary inputs not used in the loss calculation) can improve training efficiency and downstream performance, they offer limited understanding of which types of metadata are truly effective and under what conditions. In this work, we conduct a systematic evaluation and find that not all metadata types contribute equally. Only URL context speeds up training, whereas quality scores and topic/format domain information offer no clear benefit. Furthermore, the improved downstream performances of URL conditioning emerge only when longer prompts are used at inference time. In addition, we demonstrate that context-aware pretraining enables more controllable generation than context-free pretraining, in a classifier-free guidance fashion. Although topic and format metadata do not accelerate training, they are effective for steering outputs, offering human-interpretable control over generation.
Via
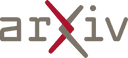
May 24, 2025
Abstract:In this work, we provide DZEN, a dataset of parallel Dzongkha and English test questions for Bhutanese middle and high school students. The over 5K questions in our collection span a variety of scientific topics and include factual, application, and reasoning-based questions. We use our parallel dataset to test a number of Large Language Models (LLMs) and find a significant performance difference between the models in English and Dzongkha. We also look at different prompting strategies and discover that Chain-of-Thought (CoT) prompting works well for reasoning questions but less well for factual ones. We also find that adding English translations enhances the precision of Dzongkha question responses. Our results point to exciting avenues for further study to improve LLM performance in Dzongkha and, more generally, in low-resource languages. We release the dataset at: https://github.com/kraritt/llm_dzongkha_evaluation.
* 24 pages, 20 figures
Via
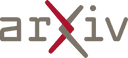