May 19, 2025
Abstract:Understanding people's preferences and needs is crucial for urban planning decisions, yet current approaches often combine them from multi-cultural and multi-city populations, obscuring important demographic differences and risking amplifying biases. We conducted a large-scale urban visual perception survey of streetscapes worldwide using street view imagery, examining how demographics -- including gender, age, income, education, race and ethnicity, and, for the first time, personality traits -- shape perceptions among 1,000 participants, with balanced demographics, from five countries and 45 nationalities. This dataset, introduced as Street Perception Evaluation Considering Socioeconomics (SPECS), exhibits statistically significant differences in perception scores in six traditionally used indicators (safe, lively, wealthy, beautiful, boring, and depressing) and four new ones we propose (live nearby, walk, cycle, green) among demographics and personalities. We revealed that location-based sentiments are carried over in people's preferences when comparing urban streetscapes with other cities. Further, we compared the perception scores based on where participants and streetscapes are from. We found that an off-the-shelf machine learning model trained on an existing global perception dataset tends to overestimate positive indicators and underestimate negative ones compared to human responses, suggesting that targeted intervention should consider locals' perception. Our study aspires to rectify the myopic treatment of street perception, which rarely considers demographics or personality traits.
* Under review
Via
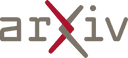