Topic:Information Extraction
What is Information Extraction? Information extraction is the process of automatically extracting structured information from unstructured text data.
Papers and Code
Jun 11, 2025
Abstract:Different medical imaging modalities capture diagnostic information at varying spatial resolutions, from coarse global patterns to fine-grained localized structures. However, most existing vision-language frameworks in the medical domain apply a uniform strategy for local feature extraction, overlooking the modality-specific demands. In this work, we present MedMoE, a modular and extensible vision-language processing framework that dynamically adapts visual representation based on the diagnostic context. MedMoE incorporates a Mixture-of-Experts (MoE) module conditioned on the report type, which routes multi-scale image features through specialized expert branches trained to capture modality-specific visual semantics. These experts operate over feature pyramids derived from a Swin Transformer backbone, enabling spatially adaptive attention to clinically relevant regions. This framework produces localized visual representations aligned with textual descriptions, without requiring modality-specific supervision at inference. Empirical results on diverse medical benchmarks demonstrate that MedMoE improves alignment and retrieval performance across imaging modalities, underscoring the value of modality-specialized visual representations in clinical vision-language systems.
Via
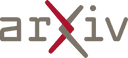
Jun 09, 2025
Abstract:Large language models (LLMs) are increasingly used to extract clinical data from electronic health records (EHRs), offering significant improvements in scalability and efficiency for real-world data (RWD) curation in oncology. However, the adoption of LLMs introduces new challenges in ensuring the reliability, accuracy, and fairness of extracted data, which are essential for research, regulatory, and clinical applications. Existing quality assurance frameworks for RWD and artificial intelligence do not fully address the unique error modes and complexities associated with LLM-extracted data. In this paper, we propose a comprehensive framework for evaluating the quality of clinical data extracted by LLMs. The framework integrates variable-level performance benchmarking against expert human abstraction, automated verification checks for internal consistency and plausibility, and replication analyses comparing LLM-extracted data to human-abstracted datasets or external standards. This multidimensional approach enables the identification of variables most in need of improvement, systematic detection of latent errors, and confirmation of dataset fitness-for-purpose in real-world research. Additionally, the framework supports bias assessment by stratifying metrics across demographic subgroups. By providing a rigorous and transparent method for assessing LLM-extracted RWD, this framework advances industry standards and supports the trustworthy use of AI-powered evidence generation in oncology research and practice.
* 18 pages, 3 tables, 1 figure
Via
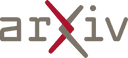
Jun 09, 2025
Abstract:In the context of the digital development of architecture, the automatic segmentation of walls and windows is a key step in improving the efficiency of building information models and computer-aided design. This study proposes an automatic segmentation model for building facade walls and windows based on multimodal semantic guidance, called Segment Any Architectural Facades (SAAF). First, SAAF has a multimodal semantic collaborative feature extraction mechanism. By combining natural language processing technology, it can fuse the semantic information in text descriptions with image features, enhancing the semantic understanding of building facade components. Second, we developed an end-to-end training framework that enables the model to autonomously learn the mapping relationship from text descriptions to image segmentation, reducing the influence of manual intervention on the segmentation results and improving the automation and robustness of the model. Finally, we conducted extensive experiments on multiple facade datasets. The segmentation results of SAAF outperformed existing methods in the mIoU metric, indicating that the SAAF model can maintain high-precision segmentation ability when faced with diverse datasets. Our model has made certain progress in improving the accuracy and generalization ability of the wall and window segmentation task. It is expected to provide a reference for the development of architectural computer vision technology and also explore new ideas and technical paths for the application of multimodal learning in the architectural field.
Via
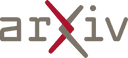
Jun 11, 2025
Abstract:Transformer-based models have achieved strong performance in remote sensing image captioning by capturing long-range dependencies and contextual information. However, their practical deployment is hindered by high computational costs, especially in multi-modal frameworks that employ separate transformer-based encoders and decoders. In addition, existing remote sensing image captioning models primarily focus on high-level semantic extraction while often overlooking fine-grained structural features such as edges, contours, and object boundaries. To address these challenges, a lightweight transformer architecture is proposed by reducing the dimensionality of the encoder layers and employing a distilled version of GPT-2 as the decoder. A knowledge distillation strategy is used to transfer knowledge from a more complex teacher model to improve the performance of the lightweight network. Furthermore, an edge-aware enhancement strategy is incorporated to enhance image representation and object boundary understanding, enabling the model to capture fine-grained spatial details in remote sensing images. Experimental results demonstrate that the proposed approach significantly improves caption quality compared to state-of-the-art methods.
Via
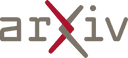
Jun 11, 2025
Abstract:Open-set domain generalization(OSDG) for hyperspectral image classification presents significant challenges due to the presence of unknown classes in target domains and the need for models to generalize across multiple unseen domains without target-specific adaptation. Existing domain adaptation methods assume access to target domain data during training and fail to address the fundamental issue of domain shift when unknown classes are present, leading to negative transfer and reduced classification performance. To address these limitations, we propose a novel open-set domain generalization framework that combines four key components: Spectrum-Invariant Frequency Disentanglement (SIFD) for domain-agnostic feature extraction, Dual-Channel Residual Network (DCRN) for robust spectral-spatial feature learning, Evidential Deep Learning (EDL) for uncertainty quantification, and Spectral-Spatial Uncertainty Disentanglement (SSUD) for reliable open-set classification. The SIFD module extracts domain-invariant spectral features in the frequency domain through attention-weighted frequency analysis and domain-agnostic regularization, while DCRN captures complementary spectral and spatial information via parallel pathways with adaptive fusion. EDL provides principled uncertainty estimation using Dirichlet distributions, enabling the SSUD module to make reliable open-set decisions through uncertainty-aware pathway weighting and adaptive rejection thresholding. Experimental results on three cross-scene hyperspectral classification tasks show that our approach achieves performance comparable to state-of-the-art domain adaptation methods while requiring no access to the target domain during training. The implementation will be made available at https://github.com/amir-khb/SSUDOSDG upon acceptance.
Via
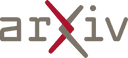
Jun 10, 2025
Abstract:Anomaly detection (AD) is a task that distinguishes normal and abnormal data, which is important for applying automation technologies of the manufacturing facilities. For MVTec dataset that is a representative AD dataset for industrial environment, many recent works have shown remarkable performances. However, the existing anomaly detection works have a limitation of showing good performance for fully-aligned datasets only, unlike real-world industrial environments. To solve this limitation, we propose HomographyAD, a novel deep anomaly detection methodology based on the ImageNet-pretrained network, which is specially designed for actual industrial dataset. Specifically, we first suggest input foreground alignment using the deep homography estimation method. In addition, we fine-tune the model by self homography learning to learn additional shape information from normal samples. Finally, we conduct anomaly detection based on the measure of how far the feature of test sample is from the distribution of the extracted normal features. By applying our proposed method to various existing AD approaches, we show performance enhancement through extensive experiments.
Via
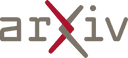
Jun 06, 2025
Abstract:Medical segmentation plays an important role in clinical applications like radiation therapy and surgical guidance, but acquiring clinically acceptable results is difficult. In recent years, progress has been witnessed with the success of utilizing transformer-like models, such as combining the attention mechanism with CNN. In particular, transformer-based segmentation models can extract global information more effectively, compensating for the drawbacks of CNN modules that focus on local features. However, utilizing transformer architecture is not easy, because training transformer-based models can be resource-demanding. Moreover, due to the distinct characteristics in the medical field, especially when encountering mid-sized and small organs with compact regions, their results often seem unsatisfactory. For example, using ViT to segment medical images directly only gives a DSC of less than 50\%, which is far lower than the clinically acceptable score of 80\%. In this paper, we used Mask2Former with deformable attention to reduce computation and proposed offset adjustment strategies to encourage sampling points within the same organs during attention weights computation, thereby integrating compact foreground information better. Additionally, we utilized the 4th feature map in Mask2Former to provide a coarse location of organs, and employed an FCN-based auxiliary head to help train Mask2Former more quickly using Dice loss. We show that our model achieves SOTA (State-of-the-Art) performance on the HaNSeg and SegRap2023 datasets, especially on mid-sized and small organs.Our code is available at link https://github.com/earis/Offsetadjustment\_Background-location\_Decoder\_Mask2former.
Via
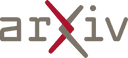
Jun 06, 2025
Abstract:Although deep learning has demonstrated remarkable capabilities in learning from unstructured data, modern tree-based ensemble models remain superior in extracting relevant information and learning from structured datasets. While several efforts have been made to accelerate tree-based models, the inherent characteristics of the models pose significant challenges for conventional accelerators. Recent research leveraging content-addressable memory (CAM) offers a promising solution for accelerating tree-based models, yet existing designs suffer from excessive memory consumption and low utilization. This work addresses these challenges by introducing RETENTION, an end-to-end framework that significantly reduces CAM capacity requirement for tree-based model inference. We propose an iterative pruning algorithm with a novel pruning criterion tailored for bagging-based models (e.g., Random Forest), which minimizes model complexity while ensuring controlled accuracy degradation. Additionally, we present a tree mapping scheme that incorporates two innovative data placement strategies to alleviate the memory redundancy caused by the widespread use of don't care states in CAM. Experimental results show that implementing the tree mapping scheme alone achieves $1.46\times$ to $21.30 \times$ better space efficiency, while the full RETENTION framework yields $4.35\times$ to $207.12\times$ improvement with less than 3% accuracy loss. These results demonstrate that RETENTION is highly effective in reducing CAM capacity requirement, providing a resource-efficient direction for tree-based model acceleration.
Via
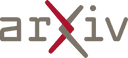
Jun 10, 2025
Abstract:Multivariate long-term and efficient time series forecasting is a key requirement for a variety of practical applications, and there are complex interleaving time dynamics in time series data that require decomposition modeling. Traditional time series decomposition methods are single and rely on fixed rules, which are insufficient for mining the potential information of the series and adapting to the dynamic characteristics of complex series. On the other hand, the Transformer-based models for time series forecasting struggle to effectively model long sequences and intricate dynamic relationships due to their high computational complexity. To overcome these limitations, we introduce KARMA, with an Adaptive Time Channel Decomposition module (ATCD) to dynamically extract trend and seasonal components. It further integrates a Hybrid Frequency-Time Decomposition module (HFTD) to further decompose Series into frequency-domain and time-domain. These components are coupled with multi-scale Mamba-based KarmaBlock to efficiently process global and local information in a coordinated manner. Experiments on eight real-world datasets from diverse domains well demonstrated that KARMA significantly outperforms mainstream baseline methods in both predictive accuracy and computational efficiency. Code and full results are available at this repository: https://github.com/yedadasd/KARMA
* 10 pages,3 figures, published to WASA2025
Via
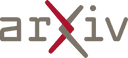
Jun 05, 2025
Abstract:Most of the current top-down multi-person pose estimation lightweight methods are based on multi-branch parallel pure CNN network architecture, which often struggle to capture the global context required for detecting semantically complex keypoints and are hindered by high latency due to their intricate and redundant structures. In this article, an approximate single-branch lightweight global modeling network (LGM-Pose) is proposed to address these challenges. In the network, a lightweight MobileViM Block is designed with a proposed Lightweight Attentional Representation Module (LARM), which integrates information within and between patches using the Non-Parametric Transformation Operation(NPT-Op) to extract global information. Additionally, a novel Shuffle-Integrated Fusion Module (SFusion) is introduced to effectively integrate multi-scale information, mitigating performance degradation often observed in single-branch structures. Experimental evaluations on the COCO and MPII datasets demonstrate that our approach not only reduces the number of parameters compared to existing mainstream lightweight methods but also achieves superior performance and faster processing speeds.
Via
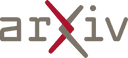