May 10, 2025
Abstract:Named Entity Recognition NER is very crucial for various natural language processing applications, including information extraction, machine translation, and sentiment analysis. Despite the ever-increasing interest in African languages within computational linguistics, existing NER systems focus mainly on English, European, and a few other global languages, leaving a significant gap for under-resourced languages. This research presents the development of a WAZOBIA-NER system tailored for the three most prominent Nigerian languages: Hausa, Yoruba, and Igbo. This research begins with a comprehensive compilation of annotated datasets for each language, addressing data scarcity and linguistic diversity challenges. Exploring the state-of-the-art machine learning technique, Conditional Random Fields (CRF) and deep learning models such as Bidirectional Long Short-Term Memory (BiLSTM), Bidirectional Encoder Representation from Transformers (Bert) and fine-tune with a Recurrent Neural Network (RNN), the study evaluates the effectiveness of these approaches in recognizing three entities: persons, organizations, and locations. The system utilizes optical character recognition (OCR) technology to convert textual images into machine-readable text, thereby enabling the Wazobia system to accept both input text and textual images for extraction purposes. The system achieved a performance of 0.9511 in precision, 0.9400 in recall, 0.9564 in F1-score, and 0.9301 in accuracy. The model's evaluation was conducted across three languages, with precision, recall, F1-score, and accuracy as key assessment metrics. The Wazobia-NER system demonstrates that it is feasible to build robust NER tools for under-resourced African languages using current NLP frameworks and transfer learning.
* 6 pages, 3 figures, 1 table
Via
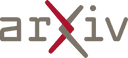