What is Resnet? ResNet (Residual Neural Network) is a deep-learning architecture that uses residual connections to enable training of very deep neural networks.
Papers and Code
Apr 24, 2025
Abstract:As state of the art neural networks (NNs) continue to grow in size, their resource-efficient implementation becomes ever more important. In this paper, we introduce a compression scheme that reduces the number of computations required for NN inference on reconfigurable hardware such as FPGAs. This is achieved by combining pruning via regularized training, weight sharing and linear computation coding (LCC). Contrary to common NN compression techniques, where the objective is to reduce the memory used for storing the weights of the NNs, our approach is optimized to reduce the number of additions required for inference in a hardware-friendly manner. The proposed scheme achieves competitive performance for simple multilayer perceptrons, as well as for large scale deep NNs such as ResNet-34.
* Accepted at the 2025 IEEE Statistical Signal Processing (SSP)
Workshop, Edinburgh
Via
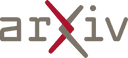
Apr 24, 2025
Abstract:This paper presents a comprehensive empirical analysis of conformal prediction methods on a challenging aerial image dataset featuring diverse events in unconstrained environments. Conformal prediction is a powerful post-hoc technique that takes the output of any classifier and transforms it into a set of likely labels, providing a statistical guarantee on the coverage of the true label. Unlike evaluations on standard benchmarks, our study addresses the complexities of data-scarce and highly variable real-world settings. We investigate the effectiveness of leveraging pretrained models (MobileNet, DenseNet, and ResNet), fine-tuned with limited labeled data, to generate informative prediction sets. To further evaluate the impact of calibration, we consider two parallel pipelines (with and without temperature scaling) and assess performance using two key metrics: empirical coverage and average prediction set size. This setup allows us to systematically examine how calibration choices influence the trade-off between reliability and efficiency. Our findings demonstrate that even with relatively small labeled samples and simple nonconformity scores, conformal prediction can yield valuable uncertainty estimates for complex tasks. Moreover, our analysis reveals that while temperature scaling is often employed for calibration, it does not consistently lead to smaller prediction sets, underscoring the importance of careful consideration in its application. Furthermore, our results highlight the significant potential of model compression techniques within the conformal prediction pipeline for deployment in resource-constrained environments. Based on our observations, we advocate for future research to delve into the impact of noisy or ambiguous labels on conformal prediction performance and to explore effective model reduction strategies.
* 17 pages, 5 figures, and 2 tables
Via
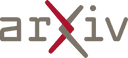
Apr 24, 2025
Abstract:We introduce the Overfitting-Underfitting Indicator (OUI), a novel tool for monitoring the training dynamics of Deep Neural Networks (DNNs) and identifying optimal regularization hyperparameters. Specifically, we validate that OUI can effectively guide the selection of the Weight Decay (WD) hyperparameter by indicating whether a model is overfitting or underfitting during training without requiring validation data. Through experiments on DenseNet-BC-100 with CIFAR- 100, EfficientNet-B0 with TinyImageNet and ResNet-34 with ImageNet-1K, we show that maintaining OUI within a prescribed interval correlates strongly with improved generalization and validation scores. Notably, OUI converges significantly faster than traditional metrics such as loss or accuracy, enabling practitioners to identify optimal WD (hyperparameter) values within the early stages of training. By leveraging OUI as a reliable indicator, we can determine early in training whether the chosen WD value leads the model to underfit the training data, overfit, or strike a well-balanced trade-off that maximizes validation scores. This enables more precise WD tuning for optimal performance on the tested datasets and DNNs. All code for reproducing these experiments is available at https://github.com/AlbertoFdezHdez/OUI.
* 10 pages, 3 figures
Via
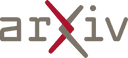
Apr 23, 2025
Abstract:An ongoing research challenge within several domains in computer vision is how to increase model generalization capabilities. Several attempts to improve model generalization performance are heavily inspired by human perceptual intelligence, which is remarkable in both its performance and efficiency to generalize to unknown samples. Many of these methods attempt to force portions of the network to be orthogonal, following some observation within neuroscience related to early vision processes. In this paper, we propose a loss component that regularizes the filtering kernels in the first convolutional layer of a network to make them nearly orthogonal. Deviating from previous works, we give the network flexibility in which pairs of kernels it makes orthogonal, allowing the network to navigate to a better solution space, imposing harsh penalties. Without architectural modifications, we report substantial gains in generalization performance using the proposed loss against previous works (including orthogonalization- and saliency-based regularization methods) across three different architectures (ResNet-50, DenseNet-121, ViT-b-16) and two difficult open-set recognition tasks: presentation attack detection in iris biometrics, and anomaly detection in chest X-ray images.
* 8 pages, 1 figure, 3 tables
Via
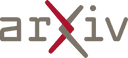
Apr 21, 2025
Abstract:Raman spectroscopy serves as a powerful and reliable tool for analyzing the chemical information of substances. The integration of Raman spectroscopy with deep learning methods enables rapid qualitative and quantitative analysis of materials. Most existing approaches adopt supervised learning methods. Although supervised learning has achieved satisfactory accuracy in spectral analysis, it is still constrained by costly and limited well-annotated spectral datasets for training. When spectral annotation is challenging or the amount of annotated data is insufficient, the performance of supervised learning in spectral material identification declines. In order to address the challenge of feature extraction from unannotated spectra, we propose a self-supervised learning paradigm for Raman Spectroscopy based on a Masked AutoEncoder, termed SMAE. SMAE does not require any spectral annotations during pre-training. By randomly masking and then reconstructing the spectral information, the model learns essential spectral features. The reconstructed spectra exhibit certain denoising properties, improving the signal-to-noise ratio (SNR) by more than twofold. Utilizing the network weights obtained from masked pre-training, SMAE achieves clustering accuracy of over 80% for 30 classes of isolated bacteria in a pathogenic bacterial dataset, demonstrating significant improvements compared to classical unsupervised methods and other state-of-the-art deep clustering methods. After fine-tuning the network with a limited amount of annotated data, SMAE achieves an identification accuracy of 83.90% on the test set, presenting competitive performance against the supervised ResNet (83.40%).
* 15 pages, 10 figures
Via
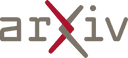
Apr 21, 2025
Abstract:Automated diagnosis of eczema using images acquired from digital camera can enable individuals to self-monitor their recovery. The process entails first segmenting out the eczema region from the image and then measuring the severity of eczema in the segmented region. The state-of-the-art methods for automated eczema diagnosis rely on deep neural networks such as convolutional neural network (CNN) and have shown impressive performance in accurately measuring the severity of eczema. However, these methods require massive volume of annotated data to train which can be hard to obtain. In this paper, we propose a self-supervised learning framework for automated eczema diagnosis under limited training data regime. Our framework consists of two stages: i) Segmentation, where we use an in-context learning based algorithm called SegGPT for few-shot segmentation of eczema region from the image; ii) Feature extraction and classification, where we extract DINO features from the segmented regions and feed it to a multi-layered perceptron (MLP) for 4-class classification of eczema severity. When evaluated on a dataset of annotated "in-the-wild" eczema images, we show that our method outperforms (Weighted F1: 0.67 $\pm$ 0.01) the state-of-the-art deep learning methods such as finetuned Resnet-18 (Weighted F1: 0.44 $\pm$ 0.16) and Vision Transformer (Weighted F1: 0.40 $\pm$ 0.22). Our results show that self-supervised learning can be a viable solution for automated skin diagnosis where labeled data is scarce.
Via
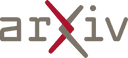
Apr 21, 2025
Abstract:This study demonstrates the capabilities of several methods for analyzing the sensitivity of neural networks to perturbations of the input data and interpreting their underlying mechanisms. The investigated approaches include the Sobol global sensitivity analysis, the local sensitivity method for input pixel perturbations and the activation maximization technique. As examples, in this study we consider a small feedforward neural network for analyzing an open tabular dataset of clinical diabetes data, as well as two classical convolutional architectures, VGG-16 and ResNet-18, which are widely used in image processing and classification. Utilization of the global sensitivity analysis allows us to identify the leading input parameters of the chosen tiny neural network and reduce their number without significant loss of the accuracy. As far as global sensitivity analysis is not applicable to larger models we try the local sensitivity analysis and activation maximization method in application to the convolutional neural networks. These methods show interesting patterns for the convolutional models solving the image classification problem. All in all, we compare the results of the activation maximization method with popular Grad-CAM technique in the context of ultrasound data analysis.
* 11 pages, 16 figures, 32 references
Via
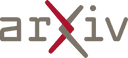
Apr 20, 2025
Abstract:In this paper, we introduce ResNetVLLM (ResNet Vision LLM), a novel cross-modal framework for zero-shot video understanding that integrates a ResNet-based visual encoder with a Large Language Model (LLM. ResNetVLLM addresses the challenges associated with zero-shot video models by avoiding reliance on pre-trained video understanding models and instead employing a non-pretrained ResNet to extract visual features. This design ensures the model learns visual and semantic representations within a unified architecture, enhancing its ability to generate accurate and contextually relevant textual descriptions from video inputs. Our experimental results demonstrate that ResNetVLLM achieves state-of-the-art performance in zero-shot video understanding (ZSVU) on several benchmarks, including MSRVTT-QA, MSVD-QA, TGIF-QA FrameQA, and ActivityNet-QA.
Via
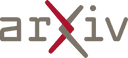
Apr 20, 2025
Abstract:Large Language Models (LLMs) have transformed natural language processing (NLP) tasks, but they suffer from hallucination, generating plausible yet factually incorrect content. This issue extends to Video-Language Models (VideoLLMs), where textual descriptions may inaccurately represent visual content, resulting in multi-modal hallucinations. In this paper, we address hallucination in ResNetVLLM, a video-language model combining ResNet visual encoders with LLMs. We introduce a two-step protocol: (1) a faithfulness detection strategy that uses a modified Lynx model to assess semantic alignment between generated captions and ground-truth video references, and (2) a hallucination mitigation strategy using Retrieval-Augmented Generation (RAG) with an ad-hoc knowledge base dynamically constructed during inference. Our enhanced model, ResNetVLLM-2, reduces multi-modal hallucinations by cross-verifying generated content against external knowledge, improving factual consistency. Evaluation on the ActivityNet-QA benchmark demonstrates a substantial accuracy increase from 54.8% to 65.3%, highlighting the effectiveness of our hallucination detection and mitigation strategies in enhancing video-language model reliability.
Via
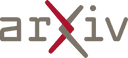
Apr 18, 2025
Abstract:Machine unlearning poses the challenge of ``how to eliminate the influence of specific data from a pretrained model'' in regard to privacy concerns. While prior research on approximated unlearning has demonstrated accuracy and efficiency in time complexity, we claim that it falls short of achieving exact unlearning, and we are the first to focus on fairness and robustness in machine unlearning algorithms. Our study presents fairness Conjectures for a well-trained model, based on the variance-bias trade-off characteristic, and considers their relevance to robustness. Our Conjectures are supported by experiments conducted on the two most widely used model architectures, ResNet and ViT, demonstrating the correlation between fairness and robustness: \textit{the higher fairness-gap is, the more the model is sensitive and vulnerable}. In addition, our experiments demonstrate the vulnerability of current state-of-the-art approximated unlearning algorithms to adversarial attacks, where their unlearned models suffer a significant drop in accuracy compared to the exact-unlearned models. We claim that our fairness-gap measurement and robustness metric should be used to evaluate the unlearning algorithm. Furthermore, we demonstrate that unlearning in the intermediate and last layers is sufficient and cost-effective for time and memory complexity.
* 5 pages
Via
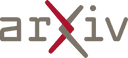