Jun 17, 2025
Abstract:Speech enhancement, particularly denoising, is vital in improving the intelligibility and quality of speech signals for real-world applications, especially in noisy environments. While prior research has introduced various deep learning models for this purpose, many struggle to balance noise suppression, perceptual quality, and speaker-specific feature preservation, leaving a critical research gap in their comparative performance evaluation. This study benchmarks three state-of-the-art models Wave-U-Net, CMGAN, and U-Net, on diverse datasets such as SpEAR, VPQAD, and Clarkson datasets. These models were chosen due to their relevance in the literature and code accessibility. The evaluation reveals that U-Net achieves high noise suppression with SNR improvements of +71.96% on SpEAR, +64.83% on VPQAD, and +364.2% on the Clarkson dataset. CMGAN outperforms in perceptual quality, attaining the highest PESQ scores of 4.04 on SpEAR and 1.46 on VPQAD, making it well-suited for applications prioritizing natural and intelligible speech. Wave-U-Net balances these attributes with improvements in speaker-specific feature retention, evidenced by VeriSpeak score gains of +10.84% on SpEAR and +27.38% on VPQAD. This research indicates how advanced methods can optimize trade-offs between noise suppression, perceptual quality, and speaker recognition. The findings may contribute to advancing voice biometrics, forensic audio analysis, telecommunication, and speaker verification in challenging acoustic conditions.
Via
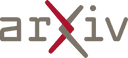