What is facial recognition? Facial recognition is an AI-based technique for identifying or confirming an individual's identity using their face. It maps facial features from an image or video and then compares the information with a collection of known faces to find a match.
Papers and Code
Nov 01, 2024
Abstract:Current face anonymization techniques often depend on identity loss calculated by face recognition models, which can be inaccurate and unreliable. Additionally, many methods require supplementary data such as facial landmarks and masks to guide the synthesis process. In contrast, our approach uses diffusion models with only a reconstruction loss, eliminating the need for facial landmarks or masks while still producing images with intricate, fine-grained details. We validated our results on two public benchmarks through both quantitative and qualitative evaluations. Our model achieves state-of-the-art performance in three key areas: identity anonymization, facial attribute preservation, and image quality. Beyond its primary function of anonymization, our model can also perform face swapping tasks by incorporating an additional facial image as input, demonstrating its versatility and potential for diverse applications. Our code and models are available at https://github.com/hanweikung/face_anon_simple .
Via
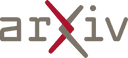
Nov 02, 2024
Abstract:In this paper, we propose a novel deep inductive transfer learning framework, named feature distribution adaptation network, to tackle the challenging multi-modal speech emotion recognition problem. Our method aims to use deep transfer learning strategies to align visual and audio feature distributions to obtain consistent representation of emotion, thereby improving the performance of speech emotion recognition. In our model, the pre-trained ResNet-34 is utilized for feature extraction for facial expression images and acoustic Mel spectrograms, respectively. Then, the cross-attention mechanism is introduced to model the intrinsic similarity relationships of multi-modal features. Finally, the multi-modal feature distribution adaptation is performed efficiently with feed-forward network, which is extended using the local maximum mean discrepancy loss. Experiments are carried out on two benchmark datasets, and the results demonstrate that our model can achieve excellent performance compared with existing ones.
Via
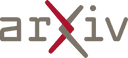
Oct 29, 2024
Abstract:In this paper, we propose a novel deep inductive transfer learning framework, named feature distribution adaptation network, to tackle the challenging multi-modal speech emotion recognition problem. Our method aims to use deep transfer learning strategies to align visual and audio feature distributions to obtain consistent representation of emotion, thereby improving the performance of speech emotion recognition. In our model, the pre-trained ResNet-34 is utilized for feature extraction for facial expression images and acoustic Mel spectrograms, respectively. Then, the cross-attention mechanism is introduced to model the intrinsic similarity relationships of multi-modal features. Finally, the multi-modal feature distribution adaptation is performed efficiently with feed-forward network, which is extended using the local maximum mean discrepancy loss. Experiments are carried out on two benchmark datasets, and the results demonstrate that our model can achieve excellent performance compared with existing ones.Our code is available at https://github.com/shaokai1209/FDAN.
Via
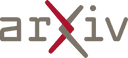
Oct 03, 2024
Abstract:Vision Large Language Models (VLLMs) are transforming the intersection of computer vision and natural language processing. Nonetheless, the potential of using visual prompts for emotion recognition in these models remains largely unexplored and untapped. Traditional methods in VLLMs struggle with spatial localization and often discard valuable global context. To address this problem, we propose a Set-of-Vision prompting (SoV) approach that enhances zero-shot emotion recognition by using spatial information, such as bounding boxes and facial landmarks, to mark targets precisely. SoV improves accuracy in face count and emotion categorization while preserving the enriched image context. Through a battery of experimentation and analysis of recent commercial or open-source VLLMs, we evaluate the SoV model's ability to comprehend facial expressions in natural environments. Our findings demonstrate the effectiveness of integrating spatial visual prompts into VLLMs for improving emotion recognition performance.
* Accepted by EMNLP2024 (Main, Long paper)
Via
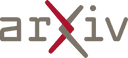
Oct 10, 2024
Abstract:Face recognition systems (FRS) can be compromised by face morphing attacks, which blend textural and geometric information from multiple facial images. The rapid evolution of generative AI, especially Generative Adversarial Networks (GAN) or Diffusion models, where encoded images are interpolated to generate high-quality face morphing images. In this work, we present a novel method for the automatic face morphing generation method \textit{MorCode}, which leverages a contemporary encoder-decoder architecture conditioned on codebook learning to generate high-quality morphing images. Extensive experiments were performed on the newly constructed morphing dataset using five state-of-the-art morphing generation techniques using both digital and print-scan data. The attack potential of the proposed morphing generation technique, \textit{MorCode}, was benchmarked using three different face recognition systems. The obtained results indicate the highest attack potential of the proposed \textit{MorCode} when compared with five state-of-the-art morphing generation methods on both digital and print scan data.
Via
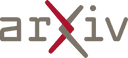
Sep 26, 2024
Abstract:In this paper, we introduce Behavior4All, a comprehensive, open-source toolkit for in-the-wild facial behavior analysis, integrating Face Localization, Valence-Arousal Estimation, Basic Expression Recognition and Action Unit Detection, all within a single framework. Available in both CPU-only and GPU-accelerated versions, Behavior4All leverages 12 large-scale, in-the-wild datasets consisting of over 5 million images from diverse demographic groups. It introduces a novel framework that leverages distribution matching and label co-annotation to address tasks with non-overlapping annotations, encoding prior knowledge of their relatedness. In the largest study of its kind, Behavior4All outperforms both state-of-the-art and toolkits in overall performance as well as fairness across all databases and tasks. It also demonstrates superior generalizability on unseen databases and on compound expression recognition. Finally, Behavior4All is way times faster than other toolkits.
Via
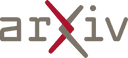
Oct 26, 2024
Abstract:Recognising intent in collaborative human robot tasks can improve team performance and human perception of robots. Intent can differ from the observed outcome in the presence of mistakes which are likely in physically dynamic tasks. We created a dataset of 1227 throws of a ball at a target from 10 participants and observed that 47% of throws were mistakes with 16% completely missing the target. Our research leverages facial images capturing the person's reaction to the outcome of a throw to predict when the resulting throw is a mistake and then we determine the actual intent of the throw. The approach we propose for outcome prediction performs 38% better than the two-stream architecture used previously for this task on front-on videos. In addition, we propose a 1-D CNN model which is used in conjunction with priors learned from the frequency of mistakes to provide an end-to-end pipeline for outcome and intent recognition in this throwing task.
* Auton Robot 47, 249-265 (2023)
* Accepted October 2022 in Autonomous Robots. Published December 2022
Via
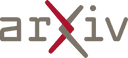
Sep 27, 2024
Abstract:Privacy issue is a main concern in developing face recognition techniques. Although synthetic face images can partially mitigate potential legal risks while maintaining effective face recognition (FR) performance, FR models trained by face images synthesized by existing generative approaches frequently suffer from performance degradation problems due to the insufficient discriminative quality of these synthesized samples. In this paper, we systematically investigate what contributes to solid face recognition model training, and reveal that face images with certain degree of similarities to their identity centers show great effectiveness in the performance of trained FR models. Inspired by this, we propose a novel diffusion-based approach (namely Center-based Semi-hard Synthetic Face Generation (CemiFace)) which produces facial samples with various levels of similarity to the subject center, thus allowing to generate face datasets containing effective discriminative samples for training face recognition. Experimental results show that with a modest degree of similarity, training on the generated dataset can produce competitive performance compared to previous generation methods.
* accepted to NeurIPS 2024. We are preparing the camera-ready version
according to the reviews
Via
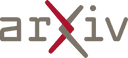
Oct 31, 2024
Abstract:Face recognition technologies are increasingly used in various applications, yet they are vulnerable to face spoofing attacks. These spoofing attacks often involve unique 3D structures, such as printed papers or mobile device screens. Although stereo-depth cameras can detect such attacks effectively, their high-cost limits their widespread adoption. Conversely, two-sensor systems without extrinsic calibration offer a cost-effective alternative but are unable to calculate depth using stereo techniques. In this work, we propose a method to overcome this challenge by leveraging facial attributes to derive disparity information and estimate relative depth for anti-spoofing purposes, using non-calibrated systems. We introduce a multi-modal anti-spoofing model, coined Disparity Model, that incorporates created disparity maps as a third modality alongside the two original sensor modalities. We demonstrate the effectiveness of the Disparity Model in countering various spoof attacks using a comprehensive dataset collected from the Intel RealSense ID Solution F455. Our method outperformed existing methods in the literature, achieving an Equal Error Rate (EER) of 1.71% and a False Negative Rate (FNR) of 2.77% at a False Positive Rate (FPR) of 1%. These errors are lower by 2.45% and 7.94% than the errors of the best comparison method, respectively. Additionally, we introduce a model ensemble that addresses 3D spoof attacks as well, achieving an EER of 2.04% and an FNR of 3.83% at an FPR of 1%. Overall, our work provides a state-of-the-art solution for the challenging task of anti-spoofing in non-calibrated systems that lack depth information.
Via
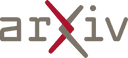
Oct 13, 2024
Abstract:Hypomimia is a non-motor symptom of Parkinson's disease that manifests as delayed facial movements and expressions, along with challenges in articulation and emotion. Currently, subjective evaluation by neurologists is the primary method for hypomimia detection, and conventional rehabilitation approaches heavily rely on verbal prompts from rehabilitation physicians. There remains a deficiency in accessible, user-friendly and scientifically rigorous assistive tools for hypomimia treatments. To investigate this, we developed HypomimaCoach, an Action Unit (AU)-based digital therapy system for hypomimia detection and rehabilitation in Parkinson's disease. The HypomimaCoach system was designed to facilitate engagement through the incorporation of both relaxed and controlled rehabilitation exercises, while also stimulating initiative through the integration of digital therapies that incorporated traditional face training methods. We extract action unit(AU) features and their relationship for hypomimia detection. In order to facilitate rehabilitation, a series of training programmes have been devised based on the Action Units (AUs) and patients are provided with real-time feedback through an additional AU recognition model, which guides them through their training routines. A pilot study was conducted with seven participants in China, all of whom exhibited symptoms of Parkinson's disease hypomimia. The results of the pilot study demonstrated a positive impact on participants' self-efficacy, with favourable feedback received. Furthermore, physician evaluations validated the system's applicability in a therapeutic setting for patients with Parkinson's disease, as well as its potential value in clinical applications.
Via
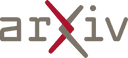