Jan 27, 2025
Abstract:With the rapid advancement of Artificial Intelligence, particularly in Natural Language Processing, Large Language Models (LLMs) have become pivotal in educational question-answering systems, especially university admission chatbots. Concepts such as Retrieval-Augmented Generation (RAG) and other advanced techniques have been developed to enhance these systems by integrating specific university data, enabling LLMs to provide informed responses on admissions and academic counseling. However, these enhanced RAG techniques often involve high operational costs and require the training of complex, specialized modules, which poses challenges for practical deployment. Additionally, in the educational context, it is crucial to provide accurate answers to prevent misinformation, a task that LLM-based systems find challenging without appropriate strategies and methods. In this paper, we introduce the Unified RAG (URAG) Framework, a hybrid approach that significantly improves the accuracy of responses, particularly for critical queries. Experimental results demonstrate that URAG enhances our in-house, lightweight model to perform comparably to state-of-the-art commercial models. Moreover, to validate its practical applicability, we conducted a case study at our educational institution, which received positive feedback and acclaim. This study not only proves the effectiveness of URAG but also highlights its feasibility for real-world implementation in educational settings.
* Under review at SoICT'24
Via
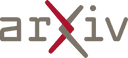