What is autonomous cars? Autonomous cars are self-driving vehicles that use artificial intelligence (AI) and sensors to navigate and operate without human intervention, using high-resolution cameras and lidars that detect what happens in the car's immediate surroundings. They have the potential to revolutionize transportation by improving safety, efficiency, and accessibility.
Papers and Code
Dec 09, 2024
Abstract:Machine Learning (ML) models, such as deep neural networks, are widely applied in autonomous systems to perform complex perception tasks. New dependability challenges arise when ML predictions are used in safety-critical applications, like autonomous cars and surgical robots. Thus, the use of fault tolerance mechanisms, such as safety monitors, is essential to ensure the safe behavior of the system despite the occurrence of faults. This paper presents an extensive literature review on safety monitoring of perception functions using ML in a safety-critical context. In this review, we structure the existing literature to highlight key factors to consider when designing such monitors: threat identification, requirements elicitation, detection of failure, reaction, and evaluation. We also highlight the ongoing challenges associated with safety monitoring and suggest directions for future research.
* 25 pages, 2 figures
Via
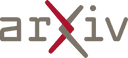
Dec 12, 2024
Abstract:Autonomous racing extends beyond the challenge of controlling a racecar at its physical limits. Professional racers employ strategic maneuvers to outwit other competing opponents to secure victory. While modern control algorithms can achieve human-level performance by computing offline racing lines for single-car scenarios, research on real-time algorithms for multi-car autonomous racing is limited. To bridge this gap, we develop game-theoretic modeling framework that incorporates the competitive aspect of autonomous racing like overtaking and blocking through a novel policy parametrization, while operating the car at its limit. Furthermore, we propose an algorithmic approach to compute the (approximate) Nash equilibrium strategy, which represents the optimal approach in the presence of competing agents. Specifically, we introduce an algorithm inspired by recently introduced framework of dynamic near-potential function, enabling real-time computation of the Nash equilibrium. Our approach comprises two phases: offline and online. During the offline phase, we use simulated racing data to learn a near-potential function that approximates utility changes for agents. This function facilitates the online computation of approximate Nash equilibria by maximizing its value. We evaluate our method in a head-to-head 3-car racing scenario, demonstrating superior performance compared to several existing baselines.
Via
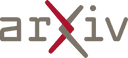
Dec 02, 2024
Abstract:Spatial understanding of the semantics of the surroundings is a key capability needed by autonomous cars to enable safe driving decisions. Recently, purely vision-based solutions have gained increasing research interest. In particular, approaches extracting a bird's eye view (BEV) from multiple cameras have demonstrated great performance for spatial understanding. This paper addresses the dependency on learned positional encodings to correlate image and BEV feature map elements for transformer-based methods. We propose leveraging epipolar geometric constraints to model the relationship between cameras and the BEV by Epipolar Attention Fields. They are incorporated into the attention mechanism as a novel attribution term, serving as an alternative to learned positional encodings. Experiments show that our method EAFormer outperforms previous BEV approaches by 2% mIoU for map semantic segmentation and exhibits superior generalization capabilities compared to implicitly learning the camera configuration.
* Accepted at WACV 2025
Via
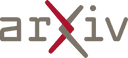
Jan 13, 2025
Abstract:Modeling car-following behavior is essential for traffic simulation, analyzing driving patterns, and understanding complex traffic flows with varying levels of autonomous vehicles. Traditional models like the Safe Distance Model and Intelligent Driver Model (IDM) require precise parameter calibration and often lack generality due to simplified assumptions about driver behavior. While machine learning and deep learning methods capture complex patterns, they require large labeled datasets. Foundation models provide a more efficient alternative. Pre-trained on vast, diverse time series datasets, they can be applied directly to various tasks without the need for extensive re-training. These models generalize well across domains, and with minimal fine-tuning, they can be adapted to specific tasks like car-following behavior prediction. In this paper, we apply Chronos, a state-of-the-art public time series foundation model, to analyze car-following behavior using the Open ACC dataset. Without fine-tuning, Chronos outperforms traditional models like IDM and Exponential smoothing with trend and seasonality (ETS), and achieves similar results to deep learning models such as DeepAR and TFT, with an RMSE of 0.60. After fine-tuning, Chronos reduces the error to an RMSE of 0.53, representing a 33.75% improvement over IDM and a 12-37% reduction compared to machine learning models like ETS and deep learning models including DeepAR, WaveNet, and TFT. This demonstrates the potential of foundation models to significantly advance transportation research, offering a scalable, adaptable, and highly accurate approach to predicting and simulating car-following behaviors.
Via
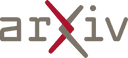
Jan 16, 2025
Abstract:In real-world sequential decision making tasks like autonomous driving, robotics, and healthcare, learning from observed state-action trajectories is critical for tasks like imitation, classification, and clustering. For example, self-driving cars must replicate human driving behaviors, while robots and healthcare systems benefit from modeling decision sequences, whether or not they come from expert data. Existing trajectory encoding methods often focus on specific tasks or rely on reward signals, limiting their ability to generalize across domains and tasks. Inspired by the success of embedding models like CLIP and BERT in static domains, we propose a novel method for embedding state-action trajectories into a latent space that captures the skills and competencies in the dynamic underlying decision-making processes. This method operates without the need for reward labels, enabling better generalization across diverse domains and tasks. Our contributions are threefold: (1) We introduce a trajectory embedding approach that captures multiple abilities from state-action data. (2) The learned embeddings exhibit strong representational power across downstream tasks, including imitation, classification, clustering, and regression. (3) The embeddings demonstrate unique properties, such as controlling agent behaviors in IQ-Learn and an additive structure in the latent space. Experimental results confirm that our method outperforms traditional approaches, offering more flexible and powerful trajectory representations for various applications. Our code is available at https://github.com/Erasmo1015/vte.
* AAMAS 2025
Via
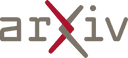
Jan 06, 2025
Abstract:The Lane Keeping Assist (LKA) system has become a standard feature in recent car models. While marketed as providing auto-steering capabilities, the system's operational characteristics and safety performance remain underexplored, primarily due to a lack of real-world testing and comprehensive data. To fill this gap, we extensively tested mainstream LKA systems from leading U.S. automakers in Tampa, Florida. Using an innovative method, we collected a comprehensive dataset that includes full Controller Area Network (CAN) messages with LKA attributes, as well as video, perception, and lateral trajectory data from a high-quality front-facing camera equipped with advanced vision detection and trajectory planning algorithms. Our tests spanned diverse, challenging conditions, including complex road geometry, adverse weather, degraded lane markings, and their combinations. A vision language model (VLM) further annotated the videos to capture weather, lighting, and traffic features. Based on this dataset, we present an empirical overview of LKA's operational features and safety performance. Key findings indicate: (i) LKA is vulnerable to faint markings and low pavement contrast; (ii) it struggles in lane transitions (merges, diverges, intersections), often causing unintended departures or disengagements; (iii) steering torque limitations lead to frequent deviations on sharp turns, posing safety risks; and (iv) LKA systems consistently maintain rigid lane-centering, lacking adaptability on tight curves or near large vehicles such as trucks. We conclude by demonstrating how this dataset can guide both infrastructure planning and self-driving technology. In view of LKA's limitations, we recommend improvements in road geometry and pavement maintenance. Additionally, we illustrate how the dataset supports the development of human-like LKA systems via VLM fine-tuning and Chain of Thought reasoning.
Via
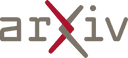
Nov 18, 2024
Abstract:Autonomous driving evaluation requires simulation environments that closely replicate actual road conditions, including real-world sensory data and responsive feedback loops. However, many existing simulations need to predict waypoints along fixed routes on public datasets or synthetic photorealistic data, \ie, open-loop simulation usually lacks the ability to assess dynamic decision-making. While the recent efforts of closed-loop simulation offer feedback-driven environments, they cannot process visual sensor inputs or produce outputs that differ from real-world data. To address these challenges, we propose DrivingSphere, a realistic and closed-loop simulation framework. Its core idea is to build 4D world representation and generate real-life and controllable driving scenarios. In specific, our framework includes a Dynamic Environment Composition module that constructs a detailed 4D driving world with a format of occupancy equipping with static backgrounds and dynamic objects, and a Visual Scene Synthesis module that transforms this data into high-fidelity, multi-view video outputs, ensuring spatial and temporal consistency. By providing a dynamic and realistic simulation environment, DrivingSphere enables comprehensive testing and validation of autonomous driving algorithms, ultimately advancing the development of more reliable autonomous cars. The benchmark will be publicly released.
Via
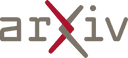
Nov 19, 2024
Abstract:3D semantic occupancy prediction, which seeks to provide accurate and comprehensive representations of environment scenes, is important to autonomous driving systems. For autonomous cars equipped with multi-camera and LiDAR, it is critical to aggregate multi-sensor information into a unified 3D space for accurate and robust predictions. Recent methods are mainly built on the 2D-to-3D transformation that relies on sensor calibration to project the 2D image information into the 3D space. These methods, however, suffer from two major limitations: First, they rely on accurate sensor calibration and are sensitive to the calibration noise, which limits their application in real complex environments. Second, the spatial transformation layers are computationally expensive and limit their running on an autonomous vehicle. In this work, we attempt to exploit a Robust and Efficient 3D semantic Occupancy (REO) prediction scheme. To this end, we propose a calibration-free spatial transformation based on vanilla attention to implicitly model the spatial correspondence. In this way, we robustly project the 2D features to a predefined BEV plane without using sensor calibration as input. Then, we introduce 2D and 3D auxiliary training tasks to enhance the discrimination power of 2D backbones on spatial, semantic, and texture features. Last, we propose a query-based prediction scheme to efficiently generate large-scale fine-grained occupancy predictions. By fusing point clouds that provide complementary spatial information, our REO surpasses the existing methods by a large margin on three benchmarks, including OpenOccupancy, Occ3D-nuScenes, and SemanticKITTI Scene Completion. For instance, our REO achieves 19.8$\times$ speedup compared to Co-Occ, with 1.1 improvements in geometry IoU on OpenOccupancy. Our code will be available at https://github.com/ICEORY/REO.
* 13 pages, 11 figures, 18 tables
Via
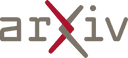
Dec 10, 2024
Abstract:Trajectory prediction of agents is crucial for the safety of autonomous vehicles, whereas previous approaches usually rely on sufficiently long-observed trajectory to predict the future trajectory of the agents. However, in real-world scenarios, it is not realistic to collect adequate observed locations for moving agents, leading to the collapse of most prediction models. For instance, when a moving car suddenly appears and is very close to an autonomous vehicle because of the obstruction, it is quite necessary for the autonomous vehicle to quickly and accurately predict the future trajectories of the car with limited observed trajectory locations. In light of this, we focus on investigating the task of instantaneous trajectory prediction, i.e., two observed locations are available during inference. To this end, we propose a general and plug-and-play instantaneous trajectory prediction approach, called ITPNet. Specifically, we propose a backward forecasting mechanism to reversely predict the latent feature representations of unobserved historical trajectories of the agent based on its two observed locations and then leverage them as complementary information for future trajectory prediction. Meanwhile, due to the inevitable existence of noise and redundancy in the predicted latent feature representations, we further devise a Noise Redundancy Reduction Former, aiming at to filter out noise and redundancy from unobserved trajectories and integrate the filtered features and observed features into a compact query for future trajectory predictions. In essence, ITPNet can be naturally compatible with existing trajectory prediction models, enabling them to gracefully handle the case of instantaneous trajectory prediction. Extensive experiments on the Argoverse and nuScenes datasets demonstrate ITPNet outperforms the baselines, and its efficacy with different trajectory prediction models.
* In Proceedings of the 30th ACM SIGKDD Conference on Knowledge
Discovery and Data Mining (KDD 2024)
Via
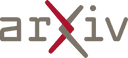
Jan 24, 2025
Abstract:This article proposes an active-learning-based adaptive trajectory tracking control method for autonomous ground vehicles to compensate for modeling errors and unmodeled dynamics. The nominal vehicle model is decoupled into lateral and longitudinal subsystems, which are augmented with online Gaussian Processes (GPs), using measurement data. The estimated mean functions of the GPs are used to construct a feedback compensator, which, together with an LPV state feedback controller designed for the nominal system, gives the adaptive control structure. To assist exploration of the dynamics, the paper proposes a new, dynamic active learning method to collect the most informative samples to accelerate the training process. To analyze the performance of the overall learning tool-chain provided controller, a novel iterative, counterexample-based algorithm is proposed for calculating the induced L2 gain between the reference trajectory and the tracking error. The analysis can be executed for a set of possible realizations of the to-be-controlled system, giving robust performance certificate of the learning method under variation of the vehicle dynamics. The efficiency of the proposed control approach is shown on a high-fidelity physics simulator and in real experiments using a 1/10 scale F1TENTH electric car.
* Submitted to IEEE Transactions on Control Systems Technology
Via
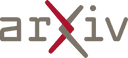