Jun 14, 2025
Abstract:Navigating the vast and rapidly growing body of scientific literature is a formidable challenge for aspiring researchers. Current approaches to supporting research idea generation often rely on generic large language models (LLMs). While LLMs are effective at aiding comprehension and summarization, they often fall short in guiding users toward practical research ideas due to their limitations. In this study, we present a novel structural framework for research ideation. Our framework, The Budget AI Researcher, uses retrieval-augmented generation (RAG) chains, vector databases, and topic-guided pairing to recombine concepts from hundreds of machine learning papers. The system ingests papers from nine major AI conferences, which collectively span the vast subfields of machine learning, and organizes them into a hierarchical topic tree. It uses the tree to identify distant topic pairs, generate novel research abstracts, and refine them through iterative self-evaluation against relevant literature and peer reviews, generating and refining abstracts that are both grounded in real-world research and demonstrably interesting. Experiments using LLM-based metrics indicate that our method significantly improves the concreteness of generated research ideas relative to standard prompting approaches. Human evaluations further demonstrate a substantial enhancement in the perceived interestingness of the outputs. By bridging the gap between academic data and creative generation, the Budget AI Researcher offers a practical, free tool for accelerating scientific discovery and lowering the barrier for aspiring researchers. Beyond research ideation, this approach inspires solutions to the broader challenge of generating personalized, context-aware outputs grounded in evolving real-world knowledge.
* Intended for AAAI's AI4Research Workshop
Via
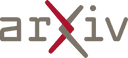