May 16, 2025
Abstract:Robust machine learning depends on clean data, yet current image data cleaning benchmarks rely on synthetic noise or narrow human studies, limiting comparison and real-world relevance. We introduce CleanPatrick, the first large-scale benchmark for data cleaning in the image domain, built upon the publicly available Fitzpatrick17k dermatology dataset. We collect 496,377 binary annotations from 933 medical crowd workers, identify off-topic samples (4%), near-duplicates (21%), and label errors (22%), and employ an aggregation model inspired by item-response theory followed by expert review to derive high-quality ground truth. CleanPatrick formalizes issue detection as a ranking task and adopts typical ranking metrics mirroring real audit workflows. Benchmarking classical anomaly detectors, perceptual hashing, SSIM, Confident Learning, NoiseRank, and SelfClean, we find that, on CleanPatrick, self-supervised representations excel at near-duplicate detection, classical methods achieve competitive off-topic detection under constrained review budgets, and label-error detection remains an open challenge for fine-grained medical classification. By releasing both the dataset and the evaluation framework, CleanPatrick enables a systematic comparison of image-cleaning strategies and paves the way for more reliable data-centric artificial intelligence.
Via
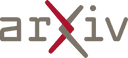