Jun 26, 2025
Abstract:Everyday, a vast stream of research documents is submitted to conferences, anthologies, journals, newsletters, annual reports, daily papers, and various periodicals. Many such publications use independent external specialists to review submissions. This process is called peer review, and the reviewers are called referees. However, it is not always possible to pick the best referee for reviewing. Moreover, new research fields are emerging in every sector, and the number of research papers is increasing dramatically. To review all these papers, every journal assigns a small team of referees who may not be experts in all areas. For example, a research paper in communication technology should be reviewed by an expert from the same field. Thus, efficiently selecting the best reviewer or referee for a research paper is a big challenge. In this research, we propose and implement program that uses a new strategy to automatically select the best reviewers for a research paper. Every research paper contains references at the end, usually from the same area. First, we collect the references and count authors who have at least one paper in the references. Then, we automatically browse the web to extract research topic keywords. Next, we search for top researchers in the specific topic and count their h-index, i10-index, and citations for the first n authors. Afterward, we rank the top n authors based on a score and automatically browse their homepages to retrieve email addresses. We also check their co-authors and colleagues online and discard them from the list. The remaining top n authors, generally professors, are likely the best referees for reviewing the research paper.
* 2018 4th International Conference on Computing, Communication and
Automation (ICCCA), Greater Noida, India, 2018, pp. 1-5
* IEEE Conference Proceedings (5 Pages)
Via
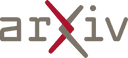