Topic:Time Series Analysis
What is Time Series Analysis? Time series analysis comprises statistical methods for analyzing a sequence of data points collected over an interval of time to identify interesting patterns and trends.
Papers and Code
Apr 08, 2025
Abstract:In exploring Predictive Health Management (PHM) strategies for Proton Exchange Membrane Fuel Cells (PEMFC), the Transformer model, widely used in data-driven approaches, excels in many fields but struggles with time series analysis due to its self-attention mechanism, which yields a complexity of the input sequence squared and low computational efficiency. It also faces challenges in capturing both global long-term dependencies and local details effectively. To tackle this, we propose the Temporal Scale Transformer (TSTransformer), an enhanced version of the inverted Transformer (iTransformer). Unlike traditional Transformers that treat each timestep as an input token, TSTransformer maps sequences of varying lengths into tokens at different stages for inter-sequence modeling, using attention to capture multivariate correlations and feed-forward networks (FFN) to encode sequence representations. By integrating a one-dimensional convolutional layer into the multivariate attention for multi-level scaling of K and V matrices, it improves local feature extraction, captures temporal scale characteristics, and reduces token count and computational costs. Experiments comparing TSTransformer with models like Long Short-Term Memory, iTransformer, and Transformer demonstrate its potential as a powerful tool for advancing PHM in renewable energy, effectively addressing the limitations of pure Transformer models in data-driven time series tasks.
* 7 figs, 10 pages
Via
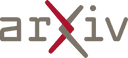
May 03, 2025
Abstract:Behind a set of rules in Deontic Defeasible Logic, there is a mapping process of normative background fragments. This process goes from text to rules and implicitly encompasses an explanation of the coded fragments. In this paper we deliver a methodology for \textit{legal coding} that starts with a fragment and goes onto a set of Deontic Defeasible Logic rules, involving a set of \textit{scenarios} to test the correctness of the coded fragments. The methodology is illustrated by the coding process of an example text. We then show the results of a series of experiments conducted with humans encoding a variety of normative backgrounds and corresponding cases in which we have measured the efforts made in the coding process, as related to some measurable features. To process these examples, a recently developed technology, Houdini, that allows reasoning in Deontic Defeasible Logic, has been employed. Finally we provide a technique to forecast time required in coding, that depends on factors such as knowledge of the legal domain, knowledge of the coding processes, length of the text, and a measure of \textit{depth} that refers to the length of the paths of legal references.
Via
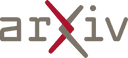
Apr 28, 2025
Abstract:Snow is an essential input for various land surface models. Seasonal snow estimates are available as snow water equivalent (SWE) from process-based reanalysis products or locally from in situ measurements. While the reanalysis products are computationally expensive and available at only fixed spatial and temporal resolutions, the in situ measurements are highly localized and sparse. To address these issues and enable the analysis of the effect of a large suite of physical, morphological, and geological conditions on the presence and amount of snow, we build a Long Short-Term Memory (LSTM) network, which is able to estimate the SWE based on time series input of the various physical/meteorological factors as well static spatial/morphological factors. Specifically, this model breaks down the SWE estimation into two separate tasks: (i) a classification task that indicates the presence/absence of snow on a specific day and (ii) a regression task that indicates the height of the SWE on a specific day in the case of snow presence. The model is trained using physical/in situ SWE measurements from the SNOw TELemetry (SNOTEL) snow pillows in the western United States. We will show that trained LSTM models have a classification accuracy of $\geq 93\%$ for the presence of snow and a coefficient of correlation of $\sim 0.9$ concerning their SWE estimates. We will also demonstrate that the models can generalize both spatially and temporally to previously unseen data.
* Preprint of journal paper in preparation. Details: 24 pages, 8
figures
Via
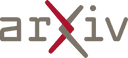
Apr 16, 2025
Abstract:Reservoir computing (RC) represents a class of state-space models (SSMs) characterized by a fixed state transition mechanism (the reservoir) and a flexible readout layer that maps from the state space. It is a paradigm of computational dynamical systems that harnesses the transient dynamics of high-dimensional state spaces for efficient processing of temporal data. Rooted in concepts from recurrent neural networks, RC achieves exceptional computational power by decoupling the training of the dynamic reservoir from the linear readout layer, thereby circumventing the complexities of gradient-based optimization. This work presents a systematic exploration of RC, addressing its foundational properties such as the echo state property, fading memory, and reservoir capacity through the lens of dynamical systems theory. We formalize the interplay between input signals and reservoir states, demonstrating the conditions under which reservoirs exhibit stability and expressive power. Further, we delve into the computational trade-offs and robustness characteristics of RC architectures, extending the discussion to their applications in signal processing, time-series prediction, and control systems. The analysis is complemented by theoretical insights into optimization, training methodologies, and scalability, highlighting open challenges and potential directions for advancing the theoretical underpinnings of RC.
* 100 pages, 17 tables, 41 figures
Via
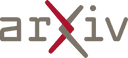
Apr 09, 2025
Abstract:Large Language Models (LLMs) have been applied to time series forecasting tasks, leveraging pre-trained language models as the backbone and incorporating textual data to purportedly enhance the comprehensive capabilities of LLMs for time series. However, are these texts really helpful for interpretation? This study seeks to investigate the actual efficacy and interpretability of such textual incorporations. Through a series of empirical experiments on textual prompts and textual prototypes, our findings reveal that the misalignment between two modalities exists, and the textual information does not significantly improve time series forecasting performance in many cases. Furthermore, visualization analysis indicates that the textual representations learned by existing frameworks lack sufficient interpretability when applied to time series data. We further propose a novel metric named Semantic Matching Index (SMI) to better evaluate the matching degree between time series and texts during our post hoc interpretability investigation. Our analysis reveals the misalignment and limited interpretability of texts in current time-series LLMs, and we hope this study can raise awareness of the interpretability of texts for time series. The code is available at https://github.com/zachysun/TS-Lang-Exp.
Via
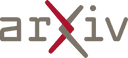
Apr 29, 2025
Abstract:Self driving cars has been the biggest innovation in the automotive industry, but to achieve human level accuracy or near human level accuracy is the biggest challenge that research scientists are facing today. Unlike humans autonomous vehicles do not work on instincts rather they make a decision based on the training data that has been fed to them using machine learning models using which they can make decisions in different conditions they face in the real world. With the advancements in machine learning especially deep learning the self driving car research skyrocketed. In this project we have presented multiple ways to predict acceleration of the autonomous vehicle using Waymo's open dataset. Our main approach was to using CNN to mimic human action and LSTM to treat this as a time series problem.
* This work contributed to research acknowledged in
https://doi.org/10.3390/app10062046
Via
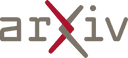
May 02, 2025
Abstract:We study the problem of learning to stabilize (LTS) a linear time-invariant (LTI) system. Policy gradient (PG) methods for control assume access to an initial stabilizing policy. However, designing such a policy for an unknown system is one of the most fundamental problems in control, and it may be as hard as learning the optimal policy itself. Existing work on the LTS problem requires large data as it scales quadratically with the ambient dimension. We propose a two-phase approach that first learns the left unstable subspace of the system and then solves a series of discounted linear quadratic regulator (LQR) problems on the learned unstable subspace, targeting to stabilize only the system's unstable dynamics and reduce the effective dimension of the control space. We provide non-asymptotic guarantees for both phases and demonstrate that operating on the unstable subspace reduces sample complexity. In particular, when the number of unstable modes is much smaller than the state dimension, our analysis reveals that LTS on the unstable subspace substantially speeds up the stabilization process. Numerical experiments are provided to support this sample complexity reduction achieved by our approach.
Via
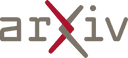
Apr 07, 2025
Abstract:In recent years, modeling and analysis of interval-valued time series have garnered increasing attention in econometrics, finance, and statistics. However, these studies have predominantly focused on statistical inference in the forecasting of univariate and multivariate interval-valued time series, overlooking another important aspect: classification. In this paper, we introduce a classification approach that treats intervals as unified entities, applicable to both univariate and multivariate interval-valued time series. Specifically, we first extend the point-valued time series imaging methods to interval-valued scenarios using the $D_K$-distance, enabling the imaging of interval-valued time series. Then, we employ suitable deep learning model for classification on the obtained imaging dataset, aiming to achieve classification for interval-valued time series. In theory, we derived a sharper excess risk bound for deep multiclassifiers based on offset Rademacher complexity. Finally, we validate the superiority of the proposed method through comparisons with various existing point-valued time series classification methods in both simulation studies and real data applications.
Via
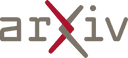
Apr 09, 2025
Abstract:Missing instances in time series data impose a significant challenge to deep learning models, particularly in regression tasks. In the Earth Observation field, satellite failure or cloud occlusion frequently results in missing time-steps, introducing uncertainties in the predicted output and causing a decline in predictive performance. While many studies address missing time-steps through data augmentation to improve model robustness, the uncertainty arising at the input level is commonly overlooked. To address this gap, we introduce Monte Carlo Temporal Dropout (MC-TD), a method that explicitly accounts for input-level uncertainty by randomly dropping time-steps during inference using a predefined dropout ratio, thereby simulating the effect of missing data. To bypass the need for costly searches for the optimal dropout ratio, we extend this approach with Monte Carlo Concrete Temporal Dropout (MC-ConcTD), a method that learns the optimal dropout distribution directly. Both MC-TD and MC-ConcTD are applied during inference, leveraging Monte Carlo sampling for uncertainty quantification. Experiments on three EO time-series datasets demonstrate that MC-ConcTD improves predictive performance and uncertainty calibration compared to existing approaches. Additionally, we highlight the advantages of adaptive dropout tuning over manual selection, making uncertainty quantification more robust and accessible for EO applications.
* Accepted at Symposium on Intelligent Data Analysis (IDA 2025)
Via
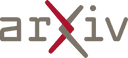
Apr 04, 2025
Abstract:In recent years, the modeling and analysis of interval-valued time series have garnered significant attention in the fields of econometrics and statistics. However, the existing literature primarily focuses on regression tasks while neglecting classification aspects. In this paper, we propose an adaptive approach for interval-valued time series classification. Specifically, we represent interval-valued time series using convex combinations of upper and lower bounds of intervals and transform these representations into images based on point-valued time series imaging methods. We utilize a fine-grained image classification neural network to classify these images, to achieve the goal of classifying the original interval-valued time series. This proposed method is applicable to both univariate and multivariate interval-valued time series. On the optimization front, we treat the convex combination coefficients as learnable parameters similar to the parameters of the neural network and provide an efficient estimation method based on the alternating direction method of multipliers (ADMM). On the theoretical front, under specific conditions, we establish a margin-based multiclass generalization bound for generic CNNs composed of basic blocks involving convolution, pooling, and fully connected layers. Through simulation studies and real data applications, we validate the effectiveness of the proposed method and compare its performance against a wide range of point-valued time series classification methods.
Via
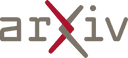