Mar 26, 2025
Abstract:One of the most significant issues as attended a lot in recent years is that of recognizing the sentiments and emotions in social media texts. The analysis of sentiments and emotions is intended to recognize the conceptual information such as the opinions, feelings, attitudes and emotions of people towards the products, services, organizations, people, topics, events and features in the written text. These indicate the greatness of the problem space. In the real world, businesses and organizations are always looking for tools to gather ideas, emotions, and directions of people about their products, services, or events related to their own. This article uses the Twitter social network, one of the most popular social networks with about 420 million active users, to extract data. Using this social network, users can share their information and opinions about personal issues, policies, products, events, etc. It can be used with appropriate classification of emotional states due to the availability of its data. In this study, supervised learning and deep neural network algorithms are used to classify the emotional states of Twitter users. The use of deep learning methods to increase the learning capacity of the model is an advantage due to the large amount of available data. Tweets collected on various topics are classified into four classes using a combination of two Bidirectional Long Short Term Memory network and a Convolutional network. The results obtained from this study with an average accuracy of 93%, show good results extracted from the proposed framework and improved accuracy compared to previous work.
Via
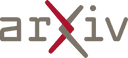