What is Sentiment Analysis? Sentiment analysis is the process of determining the sentiment of a piece of text, such as a tweet or a review.
Papers and Code
May 17, 2025
Abstract:We introduce \textbf{LAMP} (\textbf{L}inear \textbf{A}ttribution \textbf{M}apping \textbf{P}robe), a method that shines light onto a black-box language model's decision surface and studies how reliably a model maps its stated reasons to its predictions through a locally linear model approximating the decision surface. LAMP treats the model's own self-reported explanations as a coordinate system and fits a locally linear surrogate that links those weights to the model's output. By doing so, it reveals which stated factors steer the model's decisions, and by how much. We apply LAMP to three tasks: \textit{sentiment analysis}, \textit{controversial-topic detection}, and \textit{safety-prompt auditing}. Across these tasks, LAMP reveals that many LLMs exhibit locally linear decision landscapes. In addition, these surfaces correlate with human judgments on explanation quality and, on a clinical case-file data set, aligns with expert assessments. Since LAMP operates without requiring access to model gradients, logits, or internal activations, it serves as a practical and lightweight framework for auditing proprietary language models, and enabling assessment of whether a model behaves consistently with the explanations it provides.
Via
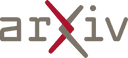
May 15, 2025
Abstract:Transformer models have revolutionized AI, powering applications like content generation and sentiment analysis. However, their deployment in Machine Learning as a Service (MLaaS) raises significant privacy concerns, primarily due to the centralized processing of sensitive user data. Private Transformer Inference (PTI) offers a solution by utilizing cryptographic techniques such as secure multi-party computation and homomorphic encryption, enabling inference while preserving both user data and model privacy. This paper reviews recent PTI advancements, highlighting state-of-the-art solutions and challenges. We also introduce a structured taxonomy and evaluation framework for PTI, focusing on balancing resource efficiency with privacy and bridging the gap between high-performance inference and data privacy.
Via
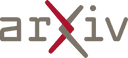
May 09, 2025
Abstract:This project performs multimodal sentiment analysis using the CMU-MOSEI dataset, using transformer-based models with early fusion to integrate text, audio, and visual modalities. We employ BERT-based encoders for each modality, extracting embeddings that are concatenated before classification. The model achieves strong performance, with 97.87\% 7-class accuracy and a 0.9682 F1-score on the test set, demonstrating the effectiveness of early fusion in capturing cross-modal interactions. The training utilized Adam optimization (lr=1e-4), dropout (0.3), and early stopping to ensure generalization and robustness. Results highlight the superiority of transformer architectures in modeling multimodal sentiment, with a low MAE (0.1060) indicating precise sentiment intensity prediction. Future work may compare fusion strategies or enhance interpretability. This approach utilizes multimodal learning by effectively combining linguistic, acoustic, and visual cues for sentiment analysis.
* 6 pages, 2 figures, 5 tables, and 19 references
Via
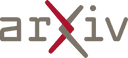
May 28, 2025
Abstract:Emotion understanding includes basic tasks (e.g., sentiment/emotion classification) and advanced tasks (e.g., sarcasm/humor detection). Current methods rely on fixed-length CoT reasoning, failing to adapt to the varying complexity of emotions. We propose a task-adaptive reasoning framework that employs DeepSeek-R1 to generate variable-length reasoning chains for different emotion tasks. By combining fine-tuning with reinforcement learning, we design a composite reward function that balances four objectives: prediction accuracy, adaptive reasoning depth control, structural diversity in reasoning paths, and suppression of repetitive logic. This approach achieves dynamic context-sensitive inference while enabling LLMs to autonomously develop deep reasoning capabilities. Experimental results demonstrate consistent improvements in both Acc and F1 scores across four tasks: emotion, sentiment, humor, and sarcasm. Notably, peak enhancements reached 3.56% F1 (2.76% Acc) for basic tasks and 37.95% F1 (23.14% Acc) for advanced tasks. Our work bridges rigid CoT reasoning and emotional complexity through adaptive-depth analysis.
Via
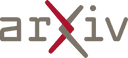
May 29, 2025
Abstract:This study introduces a novel conceptual framework distinguishing problem-seeking from problem-solving to clarify the unique features of human intelligence in contrast to AI. Problem-seeking refers to the embodied, emotionally grounded process by which humans identify and set goals, while problem-solving denotes the execution of strategies aimed at achieving such predefined objectives. The framework emphasizes that while AI excels at efficiency and optimization, it lacks the orientation derived from experiential grounding and the embodiment flexibility intrinsic to human cognition. To empirically explore this distinction, the research analyzes metadata from 157 YouTube videos discussing AI. Conducting a thematic analysis combining qualitative insights with keyword-based quantitative metrics, this mixed-methods approach uncovers recurring themes in public discourse, including privacy, job displacement, misinformation, optimism, and ethical concerns. The results reveal a dual sentiment: public fascination with AI's capabilities coexists with anxiety and skepticism about its societal implications. The discussion critiques the orthogonality thesis, which posits that intelligence is separable from goal content, and instead argues that human intelligence integrates goal-setting and goal-pursuit. It underscores the centrality of embodied cognition in human reasoning and highlights how AI's limitations come from its current reliance on computational processing. The study advocates for enhancing emotional and digital literacy to foster responsible AI engagement. It calls for reframing public discourse to recognize AI as a tool that augments -- rather than replaces -- human intelligence. By positioning problem seeking at the core of cognition and as a critical dimension of intelligence, this research offers new perspectives on ethically aligned and human-centered AI development.
* 48 pages
Via
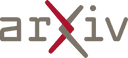
May 09, 2025
Abstract:Financial sentiment analysis (FSA) presents unique challenges to LLMs that surpass those in typical sentiment analysis due to the nuanced language used in financial contexts. The prowess of these models is often undermined by the inherent subjectivity of sentiment classifications in existing benchmark datasets like Financial Phrasebank. These datasets typically feature undefined sentiment classes that reflect the highly individualized perspectives of annotators, leading to significant variability in annotations. This variability results in an unfair expectation for LLMs during benchmarking, where they are tasked to conjecture the subjective viewpoints of human annotators without sufficient context. In this paper, we introduce the Annotators' Instruction Assisted Prompt, a novel evaluation prompt designed to redefine the task definition of FSA for LLMs. By integrating detailed task instructions originally intended for human annotators into the LLMs' prompt framework, AIAP aims to standardize the understanding of sentiment across both human and machine interpretations, providing a fair and context-rich foundation for sentiment analysis. We utilize a new dataset, WSBS, derived from the WallStreetBets subreddit to demonstrate how AIAP significantly enhances LLM performance by aligning machine operations with the refined task definitions. Experimental results demonstrate that AIAP enhances LLM performance significantly, with improvements up to 9.08. This context-aware approach not only yields incremental gains in performance but also introduces an innovative sentiment-indexing method utilizing model confidence scores. This method enhances stock price prediction models and extracts more value from the financial sentiment analysis, underscoring the significance of WSB as a critical source of financial text. Our research offers insights into both improving FSA through better evaluation methods.
Via
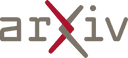
May 10, 2025
Abstract:Multi-domain sentiment classification aims to mitigate poor performance models due to the scarcity of labeled data in a single domain, by utilizing data labeled from various domains. A series of models that jointly train domain classifiers and sentiment classifiers have demonstrated their advantages, because domain classification helps generate necessary information for sentiment classification. Intuitively, the importance of sentiment classification tasks is the same in all domains for multi-domain sentiment classification; but domain classification tasks are different because the impact of domain information on sentiment classification varies across different fields; this can be controlled through adjustable weights or hyper parameters. However, as the number of domains increases, existing hyperparameter optimization algorithms may face the following challenges: (1) tremendous demand for computing resources, (2) convergence problems, and (3) high algorithm complexity. To efficiently generate the domain information required for sentiment classification in each domain, we propose a dynamic information modulation algorithm. Specifically, the model training process is divided into two stages. In the first stage, a shared hyperparameter, which would control the proportion of domain classification tasks across all fields, is determined. In the second stage, we introduce a novel domain-aware modulation algorithm to adjust the domain information contained in the input text, which is then calculated based on a gradient-based and loss-based method. In summary, experimental results on a public sentiment analysis dataset containing 16 domains prove the superiority of the proposed method.
* 17 pages, 5 figures, 3 tables
Via
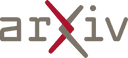
May 07, 2025
Abstract:The Tang (618 to 907) and Song (960 to 1279) dynasties witnessed an extraordinary flourishing of Chinese cultural expression, where floral motifs served as a dynamic medium for both poetic sentiment and artistic design. While previous scholarship has examined these domains independently, the systematic correlation between evolving literary emotions and visual culture remains underexplored. This study addresses that gap by employing BERT-based sentiment analysis to quantify emotional patterns in floral imagery across Tang Song poetry, then validating these patterns against contemporaneous developments in decorative arts.Our approach builds upon recent advances in computational humanities while remaining grounded in traditional sinological methods. By applying a fine tuned BERT model to analyze peony and plum blossom imagery in classical poetry, we detect measurable shifts in emotional connotations between the Tang and Song periods. These textual patterns are then cross berenced with visual evidence from textiles, ceramics, and other material culture, revealing previously unrecognized synergies between literary expression and artistic representation.
* 5 pages, 9 figures
Via
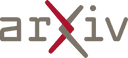
May 05, 2025
Abstract:Multimodal sentiment analysis, a pivotal task in affective computing, seeks to understand human emotions by integrating cues from language, audio, and visual signals. While many recent approaches leverage complex attention mechanisms and hierarchical architectures, we propose a lightweight, yet effective fusion-based deep learning model tailored for utterance-level emotion classification. Using the benchmark IEMOCAP dataset, which includes aligned text, audio-derived numeric features, and visual descriptors, we design a modality-specific encoder using fully connected layers followed by dropout regularization. The modality-specific representations are then fused using simple concatenation and passed through a dense fusion layer to capture cross-modal interactions. This streamlined architecture avoids computational overhead while preserving performance, achieving a classification accuracy of 92% across six emotion categories. Our approach demonstrates that with careful feature engineering and modular design, simpler fusion strategies can outperform or match more complex models, particularly in resource-constrained environments.
Via
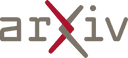
May 27, 2025
Abstract:In this paper, we present a comprehensive and systematic analysis of vision-language models (VLMs) for disparate meme classification tasks. We introduced a novel approach that generates a VLM-based understanding of meme images and fine-tunes the LLMs on textual understanding of the embedded meme text for improving the performance. Our contributions are threefold: (1) Benchmarking VLMs with diverse prompting strategies purposely to each sub-task; (2) Evaluating LoRA fine-tuning across all VLM components to assess performance gains; and (3) Proposing a novel approach where detailed meme interpretations generated by VLMs are used to train smaller language models (LLMs), significantly improving classification. The strategy of combining VLMs with LLMs improved the baseline performance by 8.34%, 3.52% and 26.24% for sarcasm, offensive and sentiment classification, respectively. Our results reveal the strengths and limitations of VLMs and present a novel strategy for meme understanding.
* 16 pages
Via
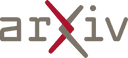